Interviews
Dr. Neil Yager, Co-Founder and Chief Scientist of Phrasee – Interview Series
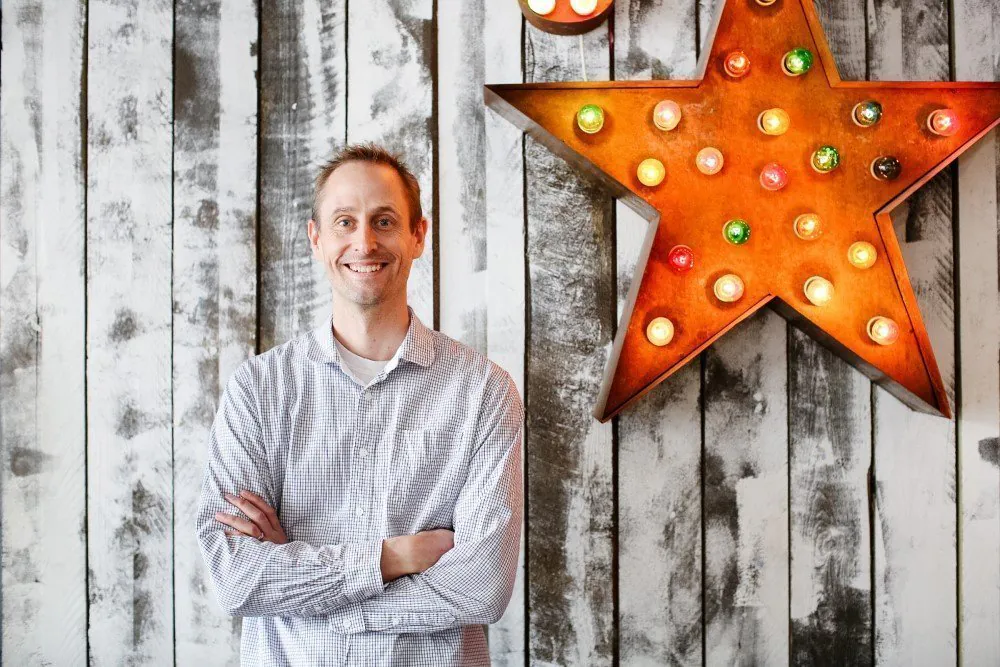
Dr. Neil Yager is the Chief Scientist of Phrasee, and the architect of the Phrasee method, an AI-powered copywriting tool that has helped to optimize marketing copy for some of the world’s most recognized brands, including eBay, Groupon, and Virgin – plus many more, from Australia to America, in over 20 languages, from English to Japanese.
Dr. Yager has written over a dozen academic publications, authored a book on data mining, and holds several patents. As one of the world’s leading experts in the commercialization of artificial intelligence, he holds a PhD in Computer Science from the University of New South Wales in Australia.
You’re a 20-year veteran of the tech industry, what were some of your previous AI related roles?
I’ve been involved in AI related work since my PhD in the mid-2000’s. However, the field has gone through several rebrandings since then. For example, 15 years ago I studied “statistical pattern recognition”. A few years later this was more commonly known as “machine learning”, which is a much more captivating name. More recently, machine learning (and “deep learning” in particular) has become synonymous with “artificial intelligence” in general. I have mixed feelings about this. On the one hand, my work with Phrasee had taught me the importance of branding. On the other hand, the term “artificial intelligence” brings baggage with it and can lead to misconceptions about the technology. I wonder where we would be if we were all still calling it “statistical pattern recognition”.
Most of my previous work was in the fields of signal processing and computer vision. I didn’t have much exposure to natural language processing prior to Phrasee. I have since learned that language is probably the most difficult problem in AI.
In 2008 you co-wrote a book called ‘Biometric System and Data Analysis: Design, Evaluation, and Data Mining’, which bridges aspects of statistics and machine learning to provide a comprehensive guide to evaluating, interpreting and understanding biometric data. Outside of more computing resources, do you believe that this field has evolved since this book was published? Could you describe how?
Deep learning has rocked the fields of computer vision, language processing and machine learning since I wrote that book. It would not be possible to write that book today without a section on deep learning.
The deep learning revolution really took off in 2012 when a deep learning model won a competition called ImageNet. ImageNet is a visual object recognition dataset where the computer determines what is in an image (e.g. “dog” or “balloon”). For decades researchers had been making incremental gains on benchmark datasets like this. Each subfield operated independently and relied heavily on domain-specific expertise. Almost overnight, all of the models painstakingly built over many years were made obsolete. Deep learning algorithms designed by outsiders were winning competitions by significant margins. This transformed the AI industry.
The field is still fast moving and has evolved even since starting Phrasee just a few years ago. For example, the deep learning tools we now rely on didn’t even exist when we founded the company. The pace of innovation brings challenges of its own.
Could you share with us what it is that Phrasee is able to do for businesses?
Phrasee solves two problems for business. Firstly, there is the problem of writing marketing copy. There are more advertising channels now than ever before (e.g. email, AdWords, social, print, podcasts, etc). It's hard to write copy for all of these that is high quality and conforms to the brand’s style and tone of voice. Phrasee addresses the problem of scale by automatically generating copy. Secondly, it is important that all of the language being used is effective. Not only does Phrasee generate language but it also uses machine learning to predict the impact of the messaging and optimize accordingly.
What was it that attracted you to the idea of using Natural Language Processing (NLP), and Deep Learning to improve the power of ad copy?
Using AI to maximize the impact of digital marketing campaigns is not a new idea. There are teams of people with PhDs in physics who have been recruited to work on ad optimization. However, in most cases they are focusing their R&D efforts on things like audience segmentation, personalization, delivery timing, ad placement, fonts, etc. When we were first bouncing ideas around for Phrasee we noticed that almost everything about ads is being optimized except the actual language being used! We identified this as a gap in the marketplace, and a huge opportunity.
Phrasee is able to improve marketing copy in over 20 languages, including Japanese. Could you discuss some of the unique Natural Language Processing issues which are encountered with foreign languages?
The most recent addition to our set of supported languages is Russian. This is a Slavic language, and it is quite distinct from other Indo-European languages. In this case it was necessary to build new rules into our language generation system so that the output is fluent and grammatically correct. This isn’t just a language issue. It is also a software development issue. When the output of our system is in the developer’s native language it is relatively easy to spot errors and verify that everything is working correctly. However, when working on Russian or Japanese we could be outputting nonsense and have no idea. It is important to have a native speaker closely involved in the QA process.
The challenge isn’t just with foreign languages. There are some interesting regional differences as well. For example, English has spelling variations for the US, UK, Australia, Canada, etc. Furthermore, there are grammatical differences. In British English you “have a look”, while in American English you “take a look”. The meaning of words can also vary from place to place. A “rubber” is an eraser in the UK, but a condom in North America! In order for NLG systems to be used for business applications they need to handle all of these subtleties.
Could you also share some details in how deep learning is used in Phrasee?
There are 2 main AI components to Phrasee’s technology. The first is Natural Language Generation (NLG), which actually produces language. The second is deep learning, and the focus here is on performance. Performance can mean different things depending on the context. For example, the goal of an email subject line is to entice the recipient to open the email and see the content inside. For Facebook, the goal might be to maximize likes or shares. Given large volumes of historical data, it is possible to find subtle trends and patterns that would never be noticed by a human. This is a standard machine learning problem.
Deep learning offers a few advantages over the traditional machine learning approach. With traditional machine learning there is a strong focus on “feature engineering”. This means that the developer needs to decide what they believe are the most important features of the language. e.g. words, length, emoji usage, etc. The problem is that this is limited by the skill and imagination of the engineer. However, with deep learning the raw text is fed into the model, and it builds its own machine representation of language (this is known as end-to-end learning). Therefore, it is free from human bias and it is a powerful approach. However, the downside is that it can be hard to understand why the model behaves the way it does. “Explainability” is an active area of research within the deep learning community. However, there is a fundamental tradeoff between the complexity of a system and our ability to understand it. Human language is messy, so successful NLP solutions typically have a high degree of complexity.
One of the functionalities of Phrasee is the ability to write in a brand's unique tone, could you elaborate on how this is performed?
When we sign up a new customer, the first thing we do is collect information about their brand’s style of communication. This includes any formal brand guidelines, historical marketing campaigns, and a series of questionnaires that we’ve developed for this purpose. All of this information is used by an in-house team of language technicians to build a customer-specific “language model”. Our language models are generative, meaning that they are able to produce never-seen-before language in a customer’s unique style.
Language models can be updated at any time. For example, at the moment we are at the peak of the COVID-19 crisis. Our language team is reviewing our models to make sure that inappropriate language cannot be created. A phrase like “These deals are going viral!” might have been harmless a few months ago but is clearly inappropriate in the midst of a global pandemic. This demonstrates the flexibility of our system.
What type of data is needed by a company that wants to get started with Phrasee?
To be honest, not a lot of data is needed to get started with us. The first step is to identify a suitable project area. For example, this might be the subject lines for weekly promotion emails. Ideally, this will have a relatively large audience and communications will be regular. Once the project has been identified we need information about the intended topic and brand voice in order to build the language model. Phrasee needs performance results on an ongoing basis. Since our solution uses machine learning, it is important that we measure and track key metrics over time. This information is fed back into our system so that it can continually optimize for engagement.
Is there anything else that you would like to share about Phrasee?
When Parry, Victoria and I started Phrasee five years ago we were sure that it would just be a matter of time before lots of other startups cropped up with similar products. Our plan was to get a jump on the competition and stay one step ahead. However, we have been surprised at the lack of entrants to this space. Where is everyone else? I think there are a few reasons for this, but one of the main ones is that language is such a difficult problem. I suspect others have tried to create similar products but failed early in the R&D stages. This is a testament to how unique our technology is.
Thank you for the informative interview on Natural Language Processing, Natural Language Generation, and Deep Learning. To learn more visitors may visit Phrasee.
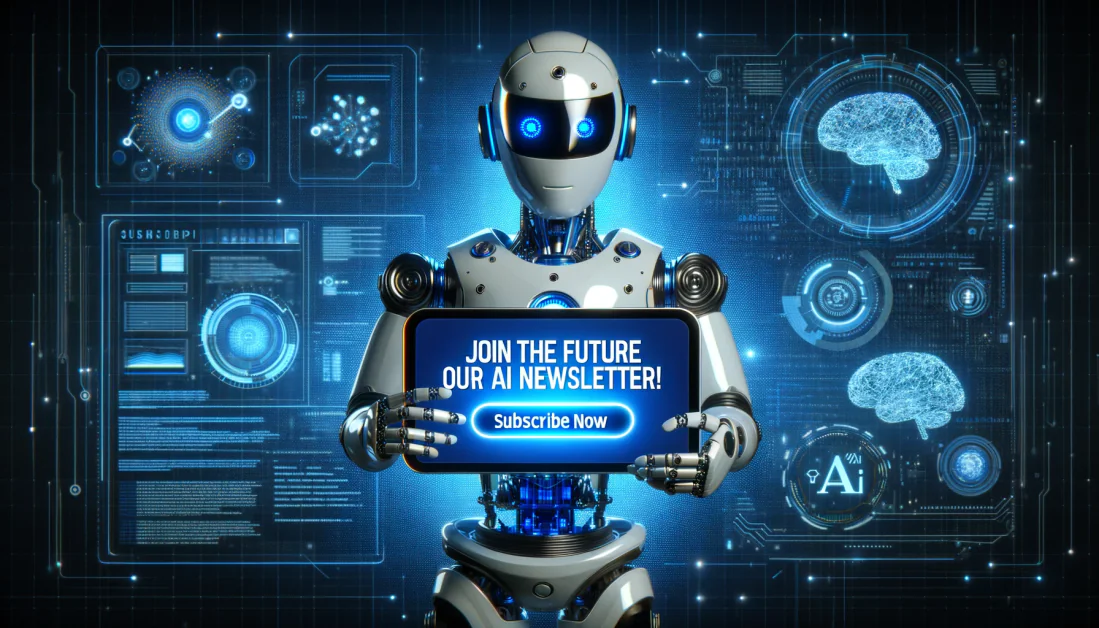
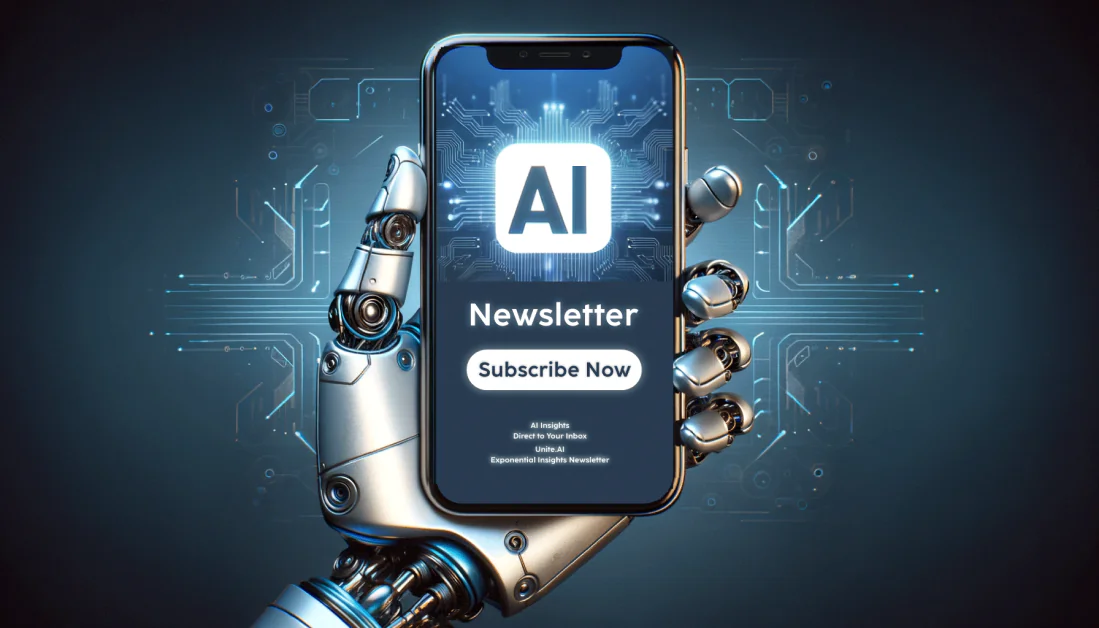