Robotics
Googleās AI teaches robots how to move by watching dogs
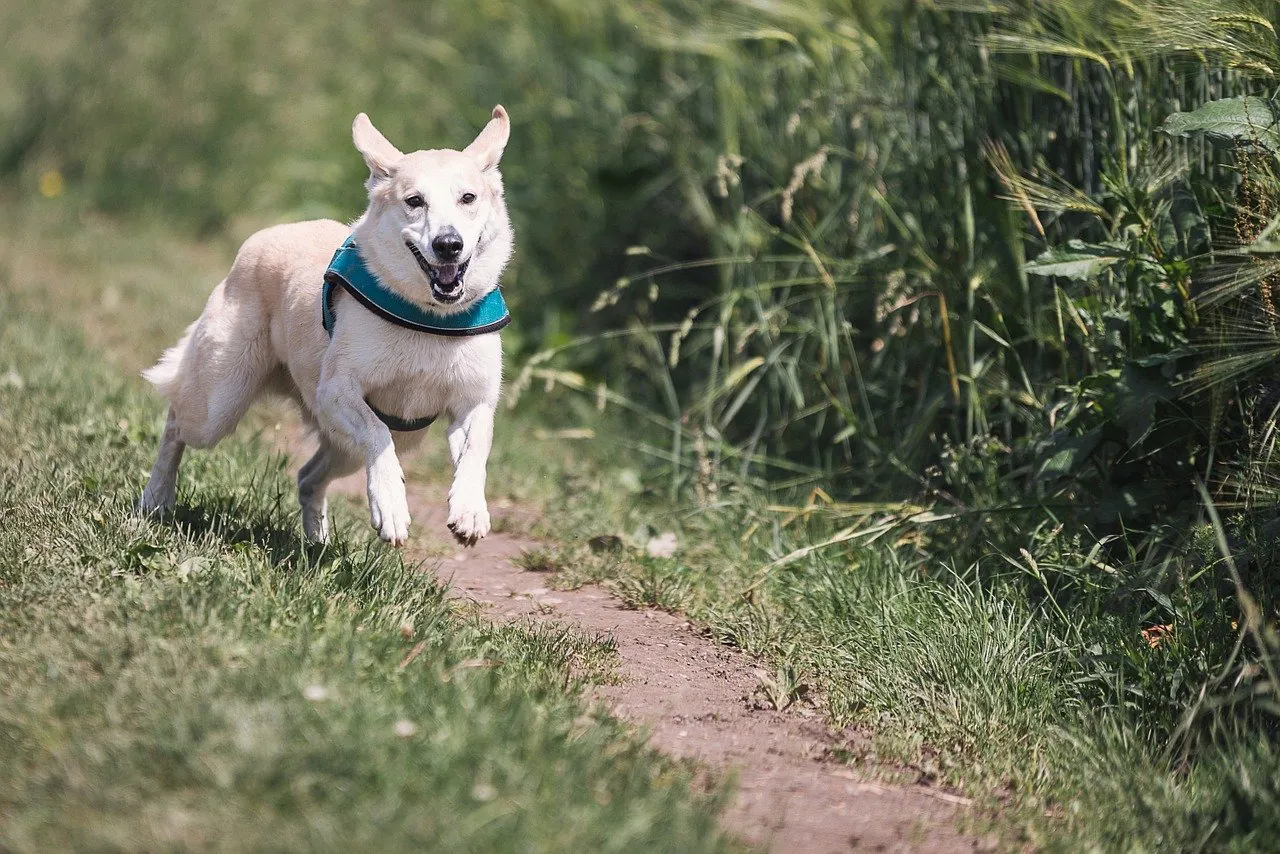
Even some of the most advanced robots today still move in somewhat clunky, jerky ways. In order to get robots to move in more lifelike, fluid ways, researchers at Google have developed an AI system that is capable of learning from the motions of real animals. The Google research team published a preprint paper that detailed their approach late last week. In the paper and an accompanying blog post, the research team describes the rationale behind the system. The authors of the paper believe that endowing robots with more natural movement could help them accomplish real-world tasks that require precise movement, such as delivering items between different levels of a building.
As VentureBeat reported, the research team utilized reinforcement learning to train their robots. The researchers began by collecting clips of real animals moving and using reinforcement learning (RL) techniques to push the robots towards imitating the movements of the animals in the video clips. In this case, the researchers trained the robots on clips of a dog, designed in a physics simulator, instructing a four-legged Unitree Laikago robot to imitate the dogās movements. After the robot was trained it was capable of accomplishing complex motions like hopping, turning, and walking swiftly, at a speed of around 2.6 miles per hour.
The training data consisted of approximately 200 million samples of dogs in motion, tracked in a physics simulation. The different motions were then run through reward functions and policies that the agents learned with. After the policies were created in the simulation, they were transferred to the real world using a technique called latent space adaptation. Because the physics simulators used to train the robots could only approximate certain aspects of real-world motion, the researchers randomly applied various perturbations to the simulation, intended to simulate operation under different conditions.
According to the research team, they were able to adapt the simulation policies to the real-world robots utilizing just eight minutes of data gathered from across 50 different trials. The researchers managed to demonstrate that the real-world robots were able to imitate a variety of different, specific motions like trotting, turning around, hopping, and pacing. They were even able to imitate animations created by animation artists, such as a combination hop and turn.
The researchers summarize the findings in the paper:
āWe show that by leveraging reference motion data, a single learning-based approach is able to automatically synthesize controllers for a diverse repertoire [of] behaviors for legged robots. By incorporating sample efficient domain adaptation techniques into the training process, our system is able to learn adaptive policies in simulation that can then be quickly adapted for real-world deployment.ā
The control policies used during the reinforcement learning process had their limitations. Because of constraints imposed by the hardware and algorithms, there were a few things the robots simply couldnāt do. They werenāt able to run or make large jumps, for instance. The learned policies also didnāt exhibit as much stability when compared with movements that were manually designed. The research team wants to take the work farther by making the controllers more robust and capable of learning from different types of data. Ideally, future versions of the framework will be able to learn from video data.