Interviews
Dr. Don Widener, Technical Director of BAE Systems’ Advanced Analytics Lab – Interview Series
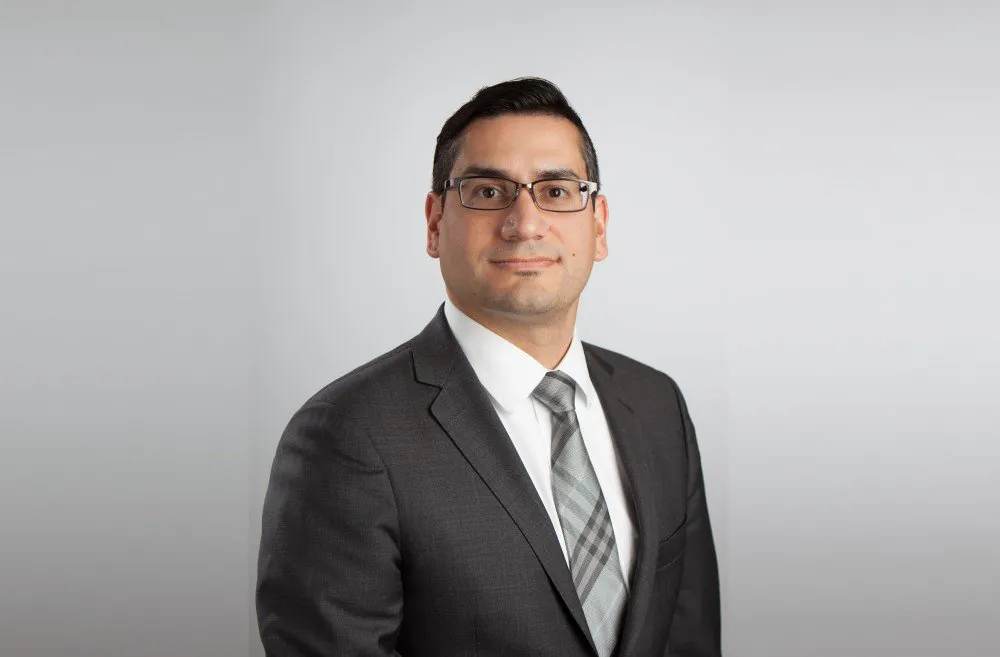
Don Widener is the Technical Director of BAE Systems’ Advanced Analytics Lab and Intelligence, Surveillance & Reconnaissance (ISR) Analysis Portfolio.
BAE Systems is a global defense, aerospace and security company employing around 83,000 people worldwide. Their wide-ranging products and services cover air, land and naval forces, as well as advanced electronics, security, information technology, and support services.
What was it that initially attracted you personally to AI and robotics?
I’ve always been interested in augmenting the ability of intelligence analysts to be more effective in their mission, whether that is through trade-craft development or technology. With an intelligence analysis background myself, I’ve focused my career on closing the gap between intelligence data collection and decision making.
In August, 2019 BAE Systems announced a partnership with UiPath, to launch the Robotic Operations Center which will bring automation and machine learning capabilities to U.S. defense and intelligence communities. Could you describe this partnership?
Democratizing AI for our 2,000+ intelligence analysts is a prime driver for BAE Systems Intelligence & Security sector’s Advanced Analytics Lab. By using Robotic Process Automation (RPA) tools like UiPath we could rapidly augment our analysts with tailored training courses and communities of practice (like the Robotic Operations Center), driving gains in efficiency and effectiveness. Analysts with no programming foundation can build automation models or “bots” to address repetitive tasks.
How will the bots from the Robotic Operations Center be used to combat cybercrime?
There is a major need for applying AI to external threat data collection for Cyber Threat analysis. At RSA 2020, we partnered with Dell to showcase their AI Ready Bundle for Machine Learning, which includes NVIDIA GPUs, libraries and frameworks, and management software in a complete solution stack. We showcased human-machine teaming by walking conference goers through an object detection model creation used to filter publicly available data to identify physical threat hot spots, which may trigger cybercrime.
Vast seas of big data will be collected to train the neural networks used by the bots. What are some of the datasets that will be collected?
BAE Systems was recently awarded the Army’s Open Source Intelligence (OSINT) contract responsible for integrating big data capabilities into our secure cloud hosting environment.
Could you describe some of the current deep learning methodologies being worked on at BAE Systems?
Some of the deep learning methodologies we are working on are Motion Imagery, Humanitarian Disaster Relief, and COVID-19.
Do you believe that object detection, and classification, is still an issue when it comes to objects which are only partially visible or obscured by other objects?
Computer vision models are less effective when partially obscured, but for national mission initiatives like Foundational Military Intelligence, even high false positive rates could still support decision advantage.
What are some of the other challenges facing computer vision?
Data labeling is a challenge. We’ve partnered with several data labeling companies for labeling unclassified data, but for classified data we are using our intelligence analyst workforce to support these CV training initiatives and this workforce is a finite resource.
Thank you for this interview. For anyone who wishes to learn more they may visit BAE Systems.
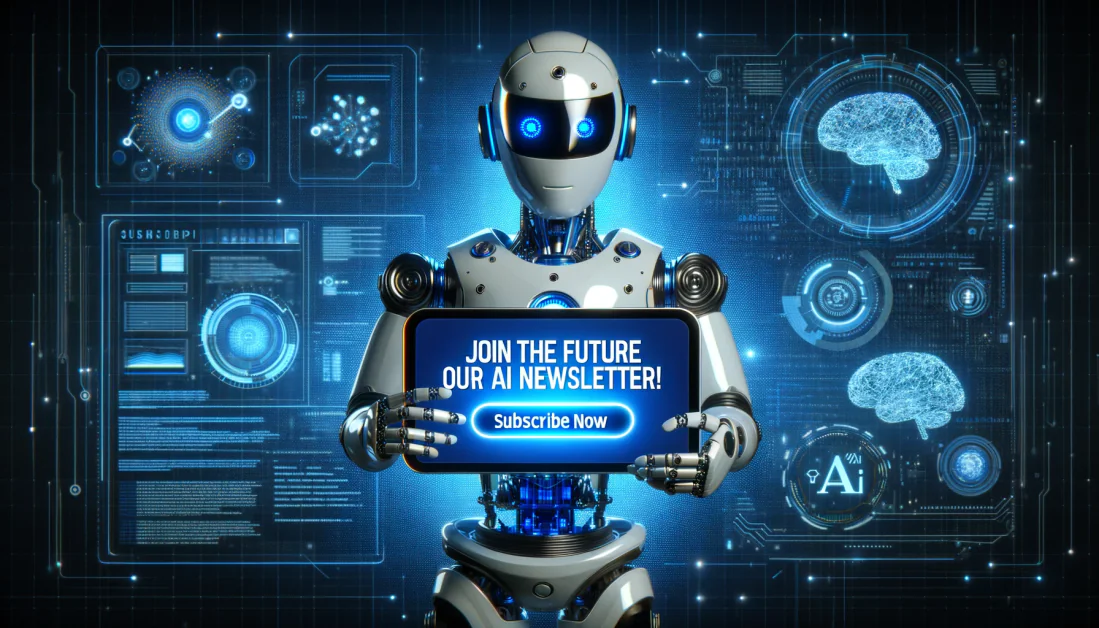
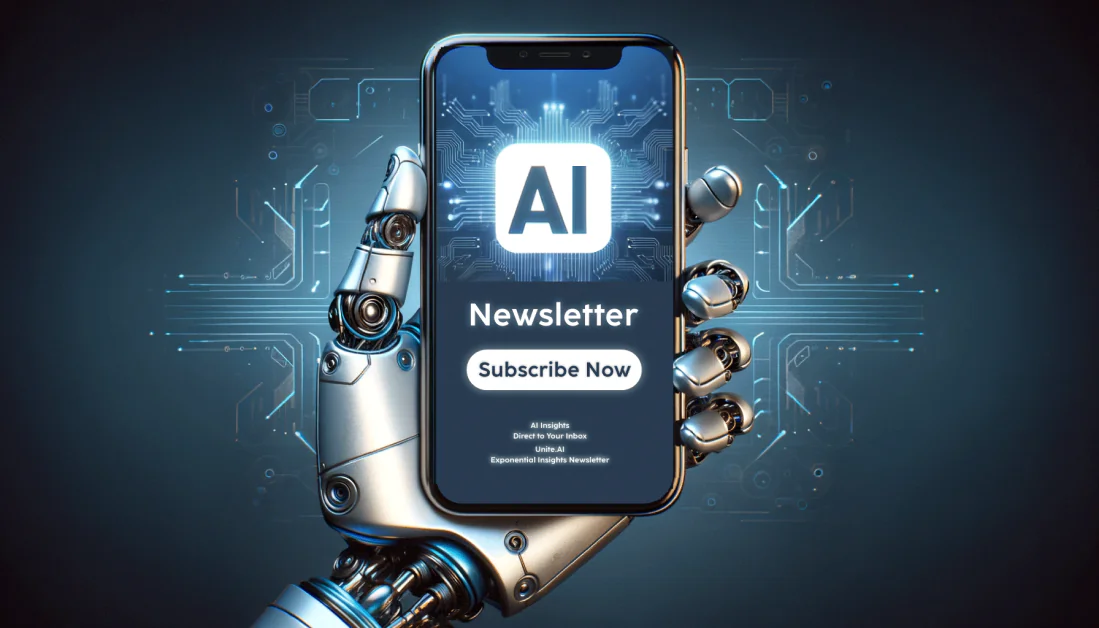