Artificial Intelligence
DARPA Aims To Bring Computer Vision AI Systems Into “Third-Wave” of Development
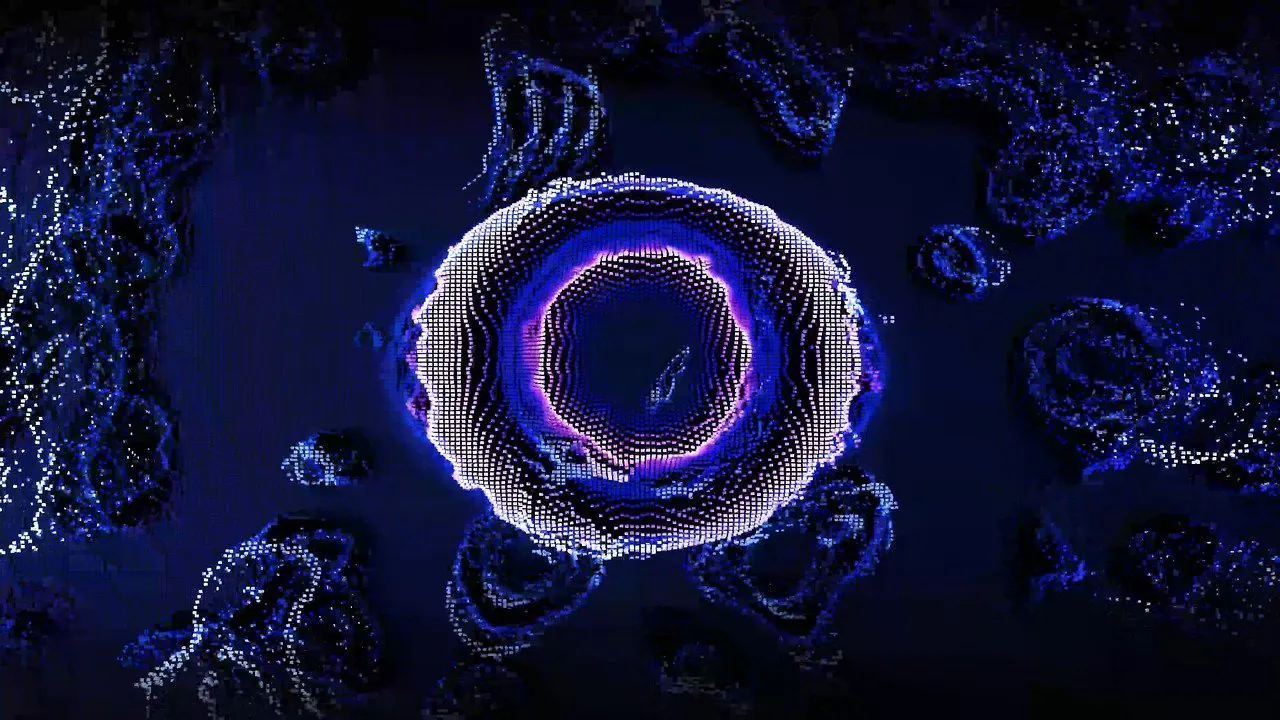
The Defense Advanced Research Projects Agent (DARPA) is starting a project intended to improve computer vision techniques and start a “third wave” of AI research. The third wave of AI research seeks to address the limitation of the first and second waves of AI technologies, which includes improvements to image recognition algorithms.
DARPA is the primary advanced research group for the US military, and it has played a critical role in the creation of many elements of modern technology, such as the earliest computer networks and earliest AI systems. DARPA recently posted an announcement about an upcoming opportunity, looking for a researcher to take part in the development of “third wave” AI theory and applications. The notice made reference to the opportunity to work on DARPA’s Pixel Intelligent Processing (IP2) system. IP2 is intended to improve the general reliability, usability, and accuracy of image/video recognition systems. IP2 is especially important for edge-computing instances, as these devices don’t have access to the computational resources needed to handle large workloads.
IP2 aims to solve two problems that limit the deployment of AI systems in edge computing devices. One issue is the creation of low-weight, low-power, and low-latency AI algorithms that can still maintain the accuracy needed to be useful. The other problem that needs solving is data complexity. The more that the complexity of the dataset can be reduced, the less processing power is needed to analyze the dataset.
DARPA researchers will aim to reduce the complexity of image datasets by using neural networks that process individual pixels. This technique reduces the dimensionality of the dataset and increasing the sparsity of the images/video in the dataset. These techniques allow the data to be processed on the backend without sacrificing the model’s accuracy. The dimensionality reduction techniques enable the AI system to extract just the most relevant information from the images/video and deliver it to the recurrent neural network model that actually makes predictions about the data. The recurrent neural net itself is also simplified to reduce power consumption.
According to DARPA officials, as quoted in NextGov:
“By immediately moving the data stream to sparse feature representation, reduced complexity [neural networks] will train to high accuracy while reducing overall compute operations by 10x.”
The IP2 project will need performers to display state-of-the-art accuracy while also institution a 20x reduction in AI algorithm processing energy-delay when handling large datasets. For example, IP2 should be able to deliver state-of-the-art results on the University of California-Berkley’s BDD100K dataset, which is a large dataset used to train self-driving vehicles by incorporating a variety of image classification tasks alongside occlusions and diversity in weather, geographic, and environmental conditions.
As DARPA gears up to tackle the third wave of computer vision algorithms and systems it is also leading an effort to automate aspects of aircraft control, recently carrying out a series of simulated tests that put AI-controlled F-16 fighter jets against an opponent. These tests were Phase 1 of a larger mission to integrate AI into the military’s fighter jets. The end of Phase 1 is focused on the transition to simulations to real-world flights, with DARPA preparing for live-fly tests later in 2021.
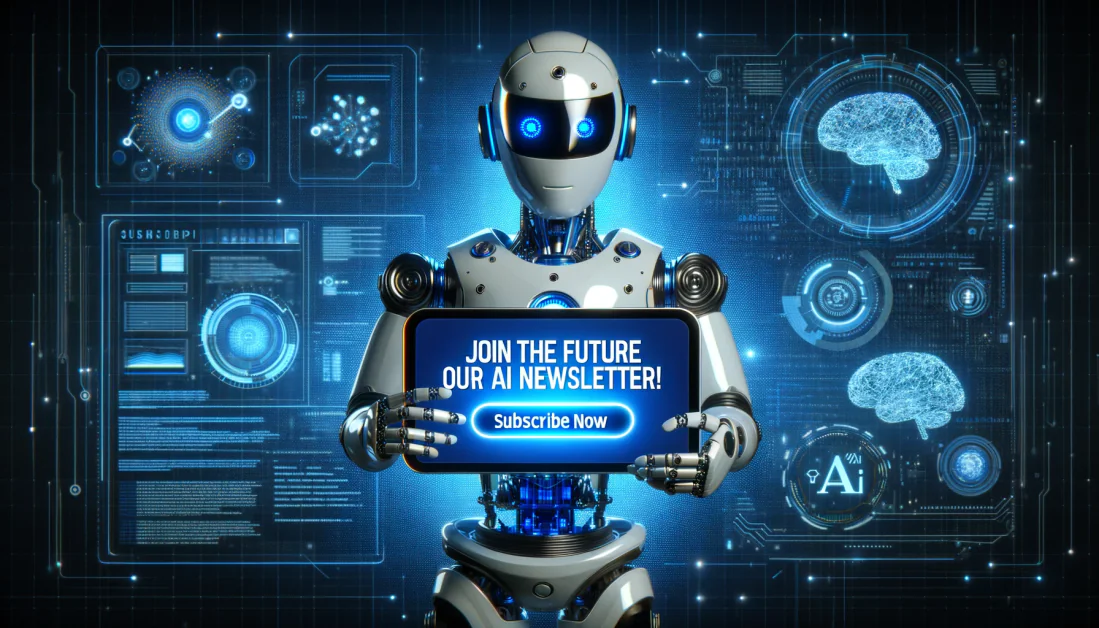
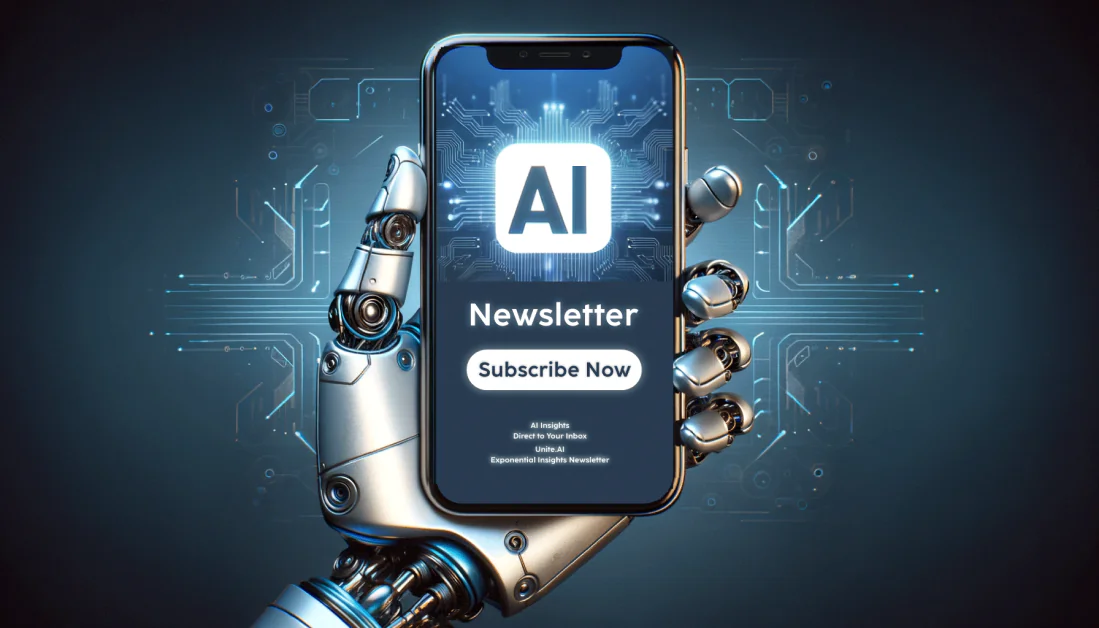