Artificial Intelligence
Computer Vision Technique Predicts Human Behavior from Videos
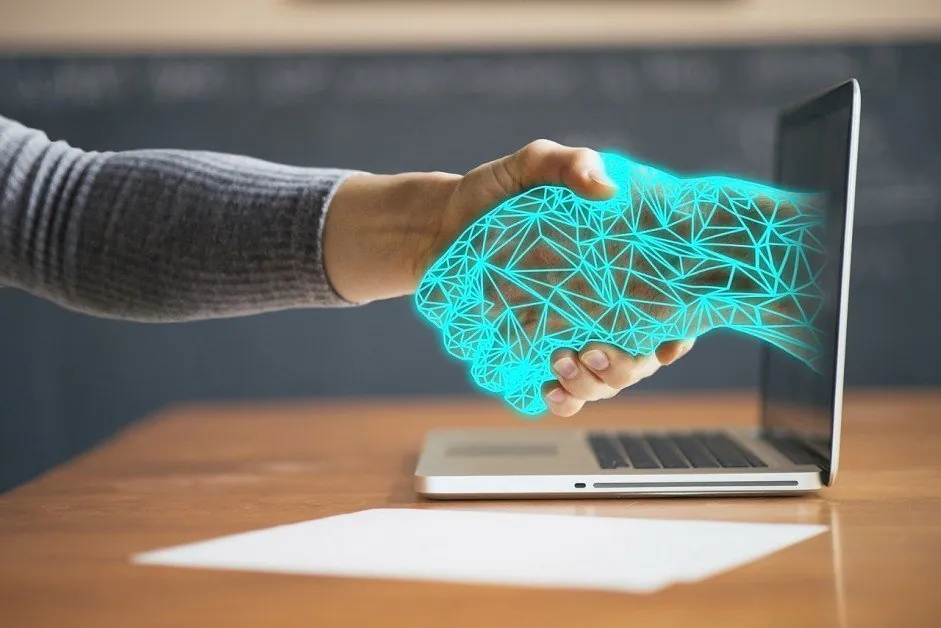
A new computer vision technique developed by Columbia Engineering researchers can predict human behavior from videos. The new technique gives machines an intuitive sense enabling them to predict what will happen next by using higher-level associations between people, animals, and objects.
Carl Vondrick is assistant professor of computer science at Columbia. Vondrick directed the study, which was presented on June 24 at the International Conference on Computer Vision and Pattern Recognition.
“Our algorithm is a step toward machines being able to make better predictions about human behavior, and thus better coordinate their actions with ours,” said Vondrick. “Our results open a number of possibilities for human-robot collaboration, autonomous vehicles, and assistive technology.”
The new method is the most accurate of its kind to date for prediction of video action events several minutes in the future. The system first analyzed thousands of hours of movies, sports games, and shows before going on to predict hundreds of activities, such as handshaking and fist bumping.
If the system cannot predict a specific action, it finds a higher-level concept that links them, such as the word “greeting.”
Past Attempts
Past attempts at predictive machine learning usually focused on predicting one action at a time, with the algorithms deciding to classify the action, for example, as a hug, handshake, high-five, or non-action. However, high uncertainty means most machine learning models are not able to find commonalities between possible options.
The team included Columbia Engineering PhD students Didac Suris and Ruoshi Liu, and the pair looked at the longer-range prediction problem a bit differently.
Suris is co-lead author of the paper.
“Not everything in the future is predictable,” said Suris. “When a person cannot foresee exactly what will happen, they play it safe and predict at a higher level of abstraction. Our algorithm is the first to learn this capability to reason abstractly about future events.”
Developing the New System
Suris and Liu relied on unusual geometries to develop AI models that organize high-level concepts and predict human behavior in the future.
Aude Oliva, who was not involved in the study, is senior research scientist at the Massachusetts Institute of Technology and co-director of the MIT-IBM Watson AI Lab.
“Prediction is the basis of human intelligence,” said Oliva. “Machines make mistakes that humans never would because they lack our ability to reason abstractly. This work is a pivotal step towards bridging this technological gap.”
The researchers developed a mathematical framework that enables machines to organize events by how predictable they are in the future. For example, the new system learns how to categorize activities like swimming and running as their own, rather than just exercising. The system is also able to account for uncertainty, which leads to more specific actions.
According to Liu, co-lead author of the paper, the newly developed technique could help computers to make nuanced decisions rather than pre-programmed actions, and it is crucial for building trust between humans and computers.
“Trust comes from the feeling that the robot really understands people,” he explains. “If machines can understand and anticipate our behaviors, computers will be able to seamlessly assist people in daily activity.”
The team will now look to verify the technique works in the real-world, and it could be deployed for safety, health, and security.
“Human behavior is often surprising,” Vondrick says. “Our algorithms enable machines to better anticipate what they are going to do next.”
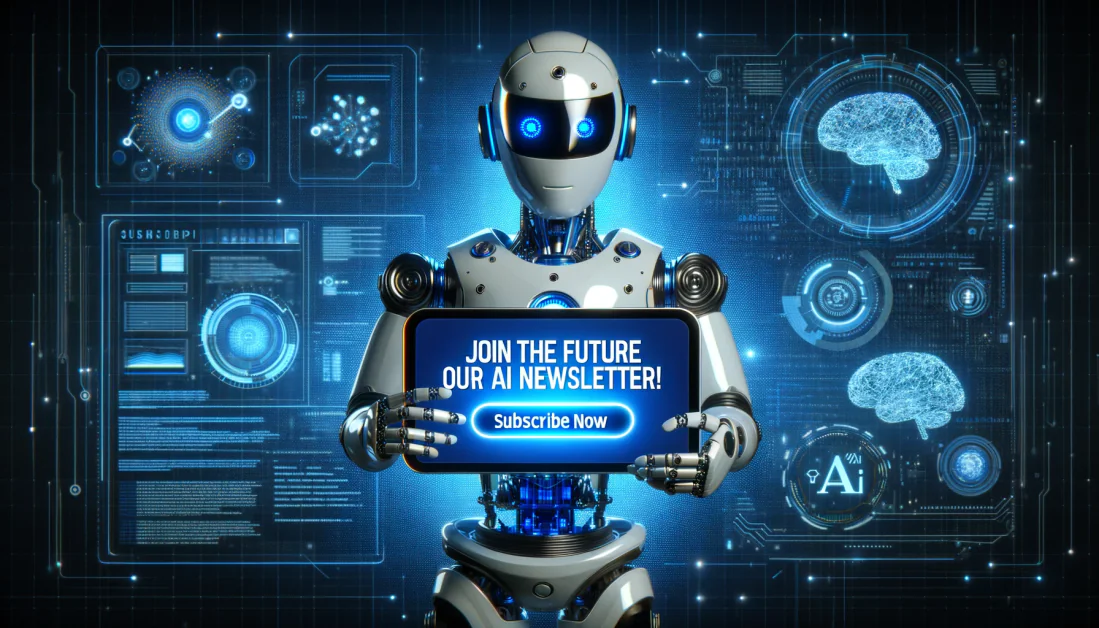
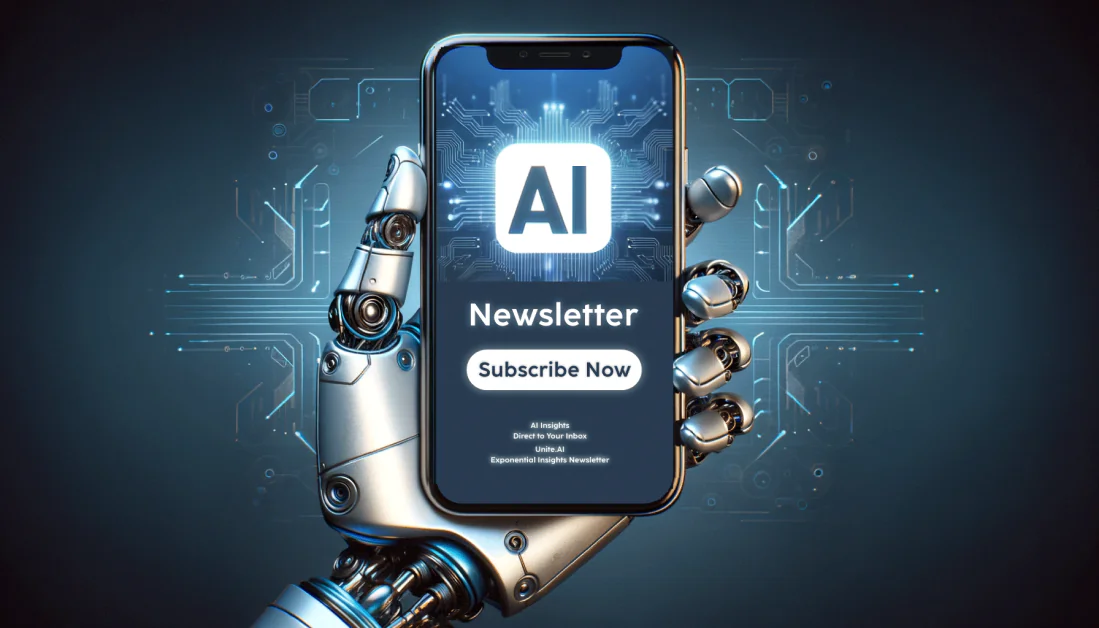