Artificial Intelligence
Algorithm Solves Complex Learning Tasks With Extreme Energy Efficiency
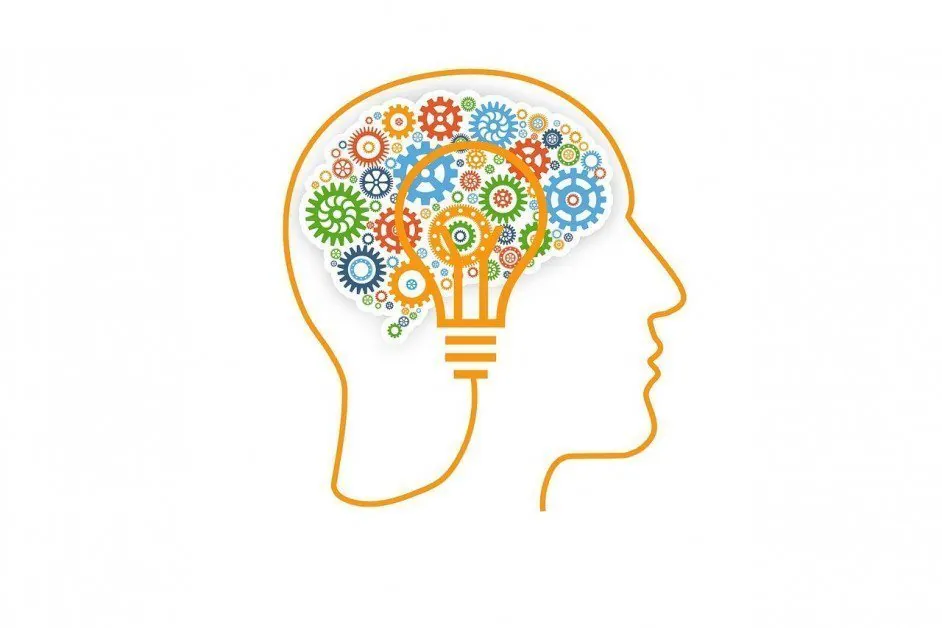
An interdisciplinary research team has made major progress in developing a machine that can process information as efficiently as the human brain, which has long been sought in the field of artificial intelligence. The team at Heidelberg University in Germany and the University of Bern in Switzerland was led by Dr. Mihai Petrovici.
The research results were published in the journal Nature Machine Intelligence.
Biologically-Inspired Artificial Neural Networks
The team looked towards biologically-inspired artificial neural networks to address this challenge. Spiking neural networks mimic the structure and function of a natural nervous system, and they are seen as a promising candidate given their features that make them powerful, fast, and energy-efficient. One of the key challenges has been to train such complex systems, and the team is now getting close to doing just that with the newly implemented algorithm.
Neurons in the brain transmit information using short electrical pulses, which are referred to as spikes. These are triggered when a certain stimulus threshold is exceeded. The exchange of information is greatly impacted by both the frequency with which a single neuron produces spikes and the temporal sequence of the individual spikes.
Julian Göltz is a doctoral candidate in the research group.
“The main difference of biological spiking networks to artificial neural networks is that, because they are using spike-based information processing, they can solve complex tasks such as image recognition and classification with extreme energy efficiency,” states Göltz.
Reaching Full Potential
The human brain and the artificial spiking neural networks both require the individual neurons to be properly connected to one another to reach their full potential. This is why the researchers needed to discover how brain-inspired, or neuromorphic systems can be adjusted to process spiking input correctly.
Laura Kriener is another member of the research team.
“This question is fundamental for the development of powerful artificial networks based on biological models,” Kriener says.
Special algorithms must be used to guarantee that neurons in a spiking neural network will fire at the right time, and these algorithms adjust the connections between the neurons so that the network can perform the required tasks. This task can be something like classifying images with high precision.
This is the type of algorithm that the team developed.
“Using this approach, we can train spiking neural networks to code and transmit information exclusively in single spikes. They thereby produce the desired results especially quickly and efficiently,” explains Göltz.
The researchers also succeeded in implementing a neural network trained with this algorithm on a physical platform. The platform was the BrainScaleS-2 neuromorphic hardware platform developed at Heidelberg University.
The researchers say that the BrainScaleS system processes information up to a thousand times faster than the human brain, all while requiring far less energy than conventional computer systems.
The system is part of the European Human Brain Project. The project integrates technologies such as neuromorphic computing into an open platform called EBRAINS.
“However, our work is not only interesting for neuromorphic computing and biologically inspired hardware. It also acknowledges the demand from the scientific community to transfer so-called Deep Learning approaches to neuroscience and thereby further unveil the secrets of the human brain,” says. Dr. Petrovici.
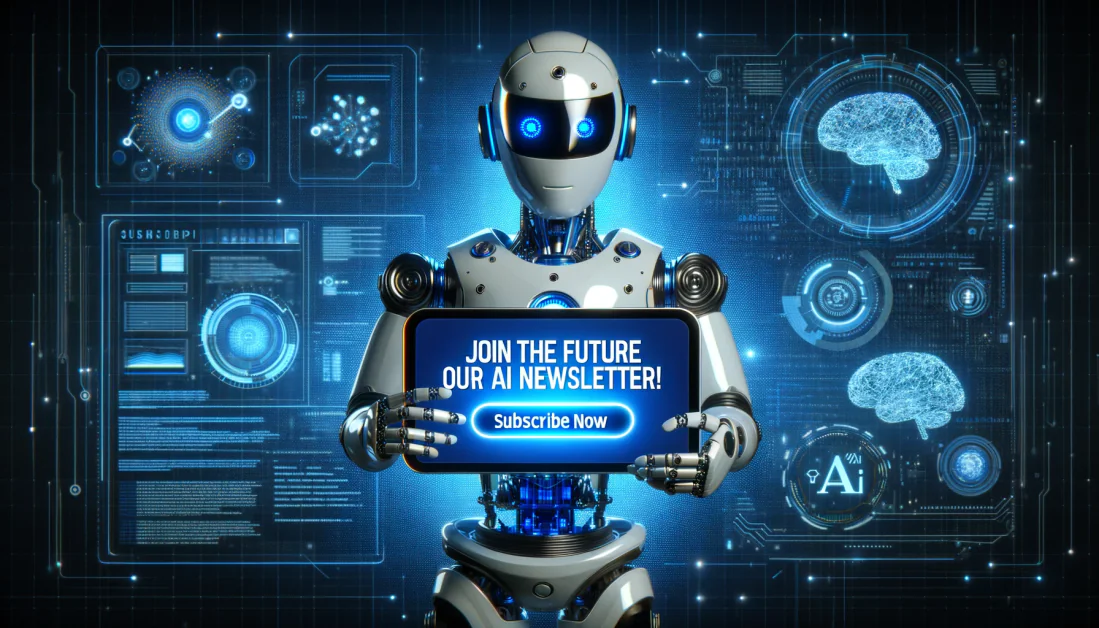
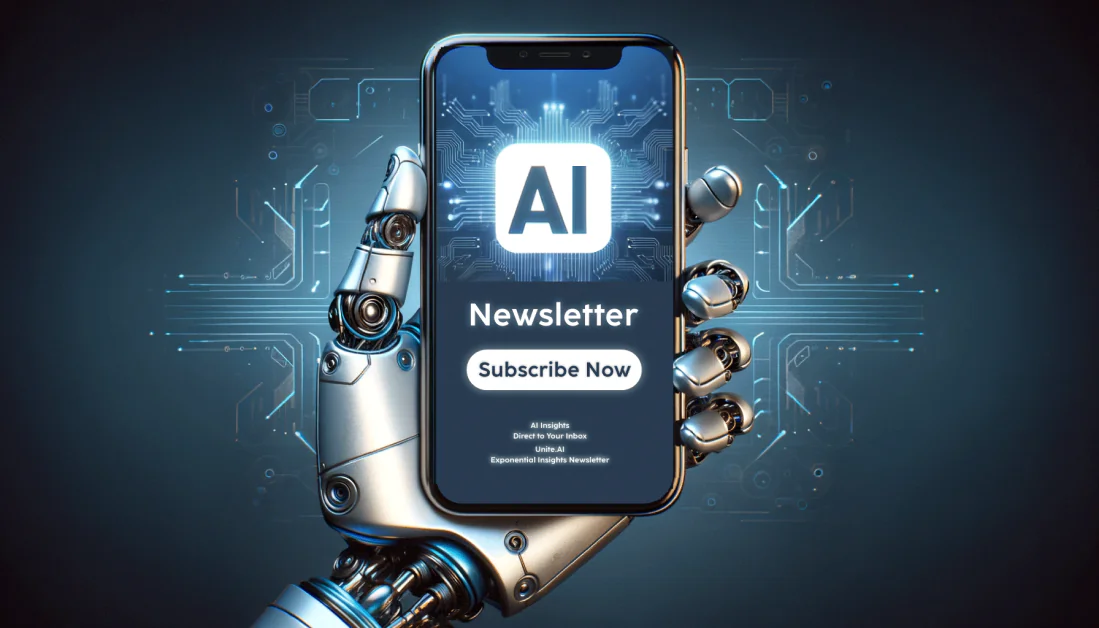