Artificial Intelligence
Researchers Gain Insight Into Making AI Think Like Us
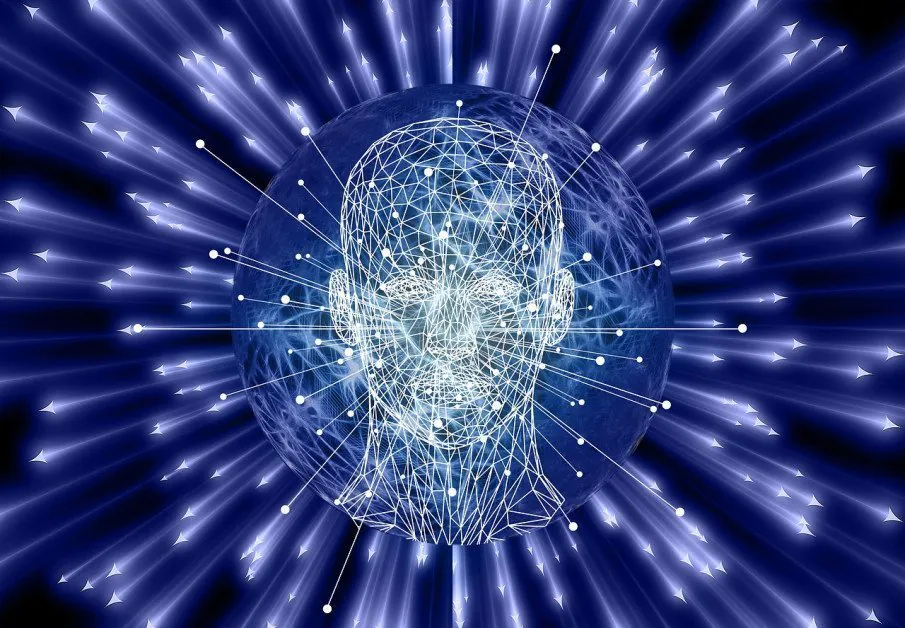
Researchers from the University of Tokyo have gained some new insight into how an artificial intelligence (AI) could be made to think like us. The researchers outline how a robot could be taught to navigate through a maze by electrically stimulating a culture of brain nerve cells that are connected to the robot.
The new research was published in Applied Physics Letters.
Nerve Cells as Physical Reservoir
The nerve cells, which are neurons, were grown from living cells. They act as the physical reservoir that enables the machine to construct coherent signals, which are regarded as homeostatic signals.
These signals inform the robot that the environment is being maintained within a certain range, and they act as a baseline as it moves freely through the maze.
In the testing, the neurons in the cell culture were distrubred by an electric impulse whenever the robot veered in the wrong direction or faced the wrong way. Throughout all of the trials, the robot was continually fed the homeostatic signals interrupted by the disturbance signals until it was able to successfully solve the maze task.
Achieving Goal-Directed Behavior
According to the researchers, these findings suggest that goal-directed behavior can be generated without any additional learning. This is achieved by sending disturbance signals to an embodied system. The robot was entirely dependent on the electrical trial-and-error impulses since it could not see the environment or obtain sensory information.
Hirokazu Takahashi is an associate professor of mechano-informatics.
“I, myself, was inspired by our experiments to hypothesize that intelligence in a living system emerges from a mechanism extracting a coherent output from a disorganized state, or a chaotic state,” Takahashi said.
The researchers used this principle to show that intelligent task-solving abilities can be produced through the use of physical reservoir computers, enabling the extraction of neuronal signals. This also enables the delivery of homeostatic or disturbance signals, and all of this allows the computer to create a reservoir that understands how to solve the task.
“A brain of [an] elementary school kid is unable to solve mathematical problems in a college admission exam, possibly because the dynamics of the brain or their ‘physical reservoir computer' is not rich enough,” said Takahashi. “Task-solving ability is determined by how rich a repertoire of spatiotemporal patterns the network can generate.”
According to the team, the use of physical reservoir computing in this context could contribute to a better understanding of how the brain works, and it could lead to the novel development of a neuromorphic computer.