Artificial Intelligence
AI Models Provide Insight Into How Brain Processes Language
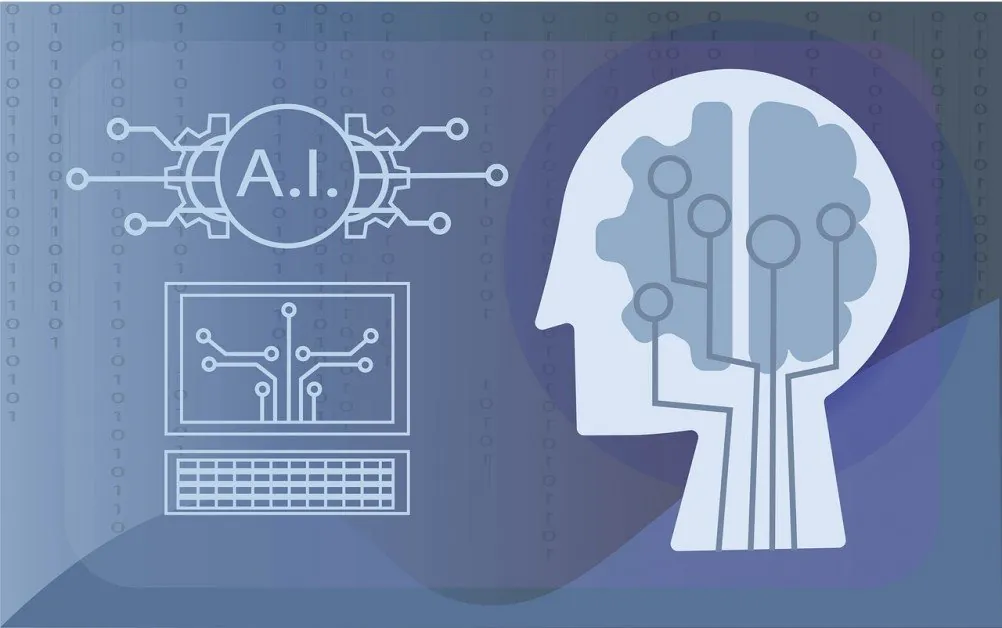
New research coming out of the Massachusetts Institute of Technology suggests that the underlying function of ânext-word predictionâ computational models resembles the function of language-processing centers in the human brain.Â
Meaning of Language
The newest predictive language models could be learning something about the underlying meaning of language, which would be a huge step forward in the field. The models predict the word that comes next, but they are also performing tasks that require a degree of genuine understanding. These tasks include question answering, document summarization, and story completion.Â
The models were designed to optimize performance for predicting text without attempting to mimic anything regarding how the human brain understands language. However, the MIT team of neuroscientists suggest that something is happening in this regard.Â
One of the more interesting insights of this research is that computer models that perform well on other types of language tasks do not show this similarity to the human brain. This is seen as evidence that the human brain could be using next-word prediction to carry out language processing.Â
Nancy Kanwisher is Walter A. Rosenblith Professor of Cognitive Neuroscience. She is also a member of MITâs McGovern Institute for Brain Research and Center for Brains, Minds, and Machines (CBMM), and an author of the study.
“The better the model is at predicting the next word, the more closely it fits the human brain,” says Kanwisher. “It's amazing that the models fit so well, and it very indirectly suggests that maybe what the human language system is doing is predicting what's going to happen next.”
The study appeared in the Proceedings of the National Academy of Sciences.Â
It also included senior authors Joshue Tenenbaum, professor of cognitive science at MIT and a member of CBMM and MITâs Artificial Intelligence (CSAIL); and Eveline Fedorenko, Frederick A. and Carole J. Middleton Career Development Associate Professor of Neuroscience and a member of the McGovern Institute. The first author of the paper was Martin Schrimpf, an MIT graduate student.Â
The Study
The MIT team compared language-processing centers in the human brain with language-processing models. They analyzed 43 different language models, including those that are optimized for next-word prediction, such as GPT-3. Other models were designed to perform different language tasks, such as filling in a blank.Â
Each model was presented with a string of words, and the researchers measured the activity of the nodes that make up the network, The patterns were then compared to activity in the brain, which were measured in subjects performing three language tasks: listening to stories, reading sentences one at a time, and reading sentences in which one word is revealed at a time.Â
The human datasets included functional magnetic resonance (fMRI) data and intracranial electrocorticographic measurements that were taken from people undergoing brain surgery for epilepsy.
The researchers found that the best-performing next-word prediction models had activity patterns that closely resembled those seen in the human brain. Those same models also demonstrated activity that was highly correlated with measures of human behavioral measures like how fast people can read the text.
“We found that the models that predict the neural responses well also tend to best predict human behavior responses, in the form of reading times. And then both of these are explained by the model performance on next-word prediction. This triangle really connects everything together,” Schrimpf says.
The researchers will now look to build variants of the language processing models, which could enable them to see how small changes in their architecture affect performance and their ability to fit human neural data.Â