Healthcare
AI Models Use Natural Language Processing To Predict Risk Of Developing Alzheimer’s
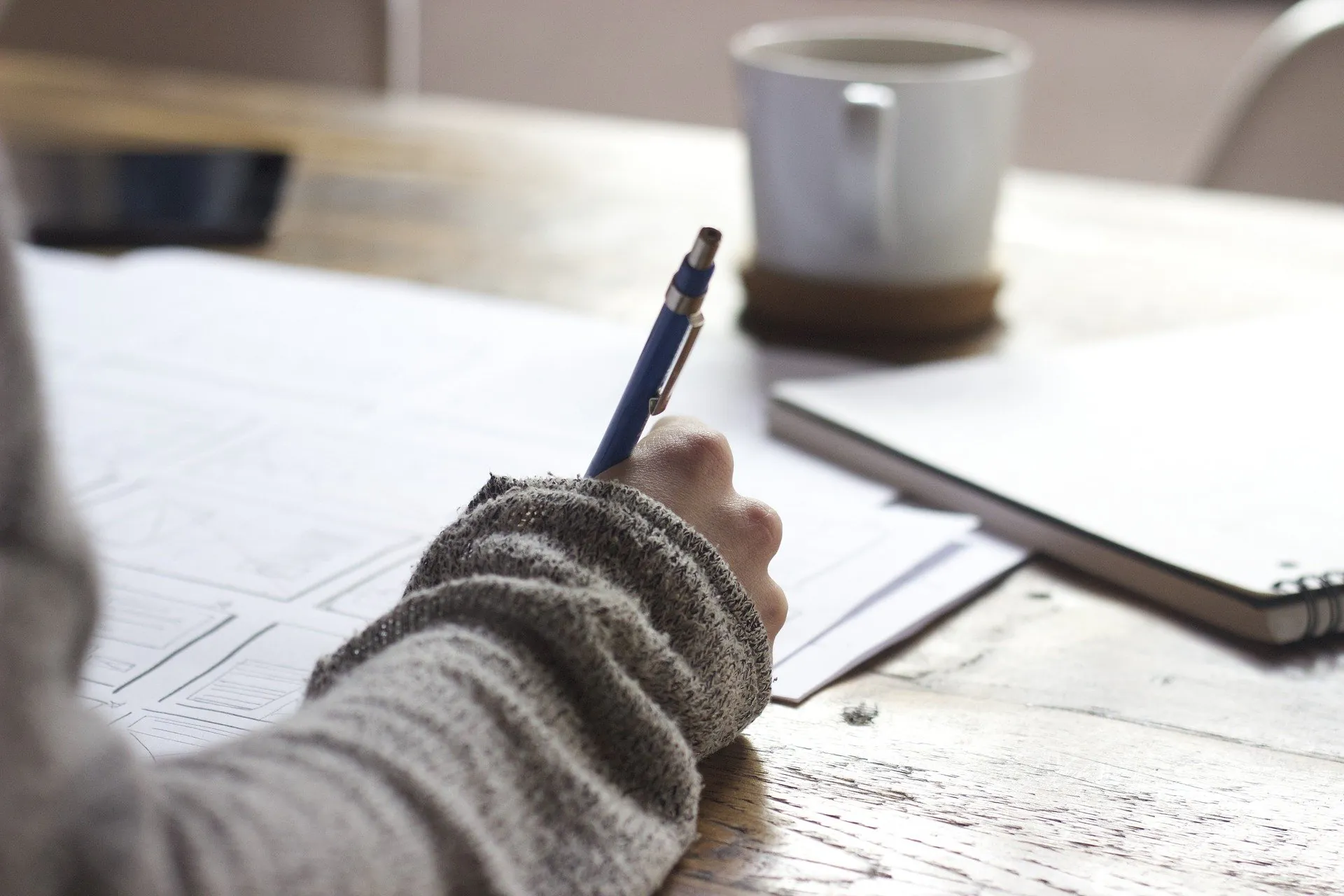
A team of AI researchers from IBM and Pfizer has developed AI algorithms that can potentially detect signs of Alzheimer’s disease by analyzing people’s writing and finding linguistic patterns.
Other AI researchers have developed models intended to predict the development of Alzheimer’s by analyzing PET scans or by interpreting clinical test data. These other models were trained on recent data, but the model developed by the IBM-Pfizer team was trained on data from the Framingham Heart Study, which includes data on over 14,000 people across three generations and six decades. The long-term nature of the data is important, as if the AI is capable of reliably detecting patterns within large populations over long periods of time, researchers could potentially predict the manifestation of Alzheimer’s years in advance of current diagnostic techniques. Furthermore, it could be a reliable diagnosis method that doesn’t require the use of scanning technology or invasive tests, increasing the range of scenarios where it can be used.
According to IBM’s vice president of healthcare and life sciences, Ajay Royyuru, the AI models developed by the research team can function as a tool that helps medical practitioners get clues about the possible development of Alzheimer’s in advance of clinical tests. The models can essentially function as early warning systems that prompt medical practitioners to pursue more extensive tests.
In order to train the AI models, the research team used transcriptions of handwritten responses to various questions. Participants in the Framingham Heart Study were asked to describe a picture of a setting using their natural language. The answers generated by the respondents were digitized and the transcriptions were fed to the machine learning algorithms as training data. According to IBM, the models were able to pick up on certain linguistic features that are correlated with the development of neurodegenerative disorders. Clinicians have long found that certain use of repeated words, misspellings, and a preference for simple phrases over more complex sentences can be indicative of the progression of Alzheimer’s, and the AI models hit on these same features.
According to the results of the study, the main model achieved approximately 70% accuracy in predicting which of the participants in the original study eventually developed Alzheimer’s disease by the age of 85. The models, and therefore the results, were derived from the historical data within the original study. They didn’t really predict future events. In addition, the AI model was trained on the oldest subsection of the Framingham population. This population was primarily non-Hispanic white, and as a result, there are limits to how generalizable the results are for other ethnicities and other populations around the world. The sample size for the study was rather small as well, consisting of just 40 individuals who developed dementia and 40 who didn’t.
Despite these limitations, the study has value as one of the first studies to analyze large-scale real-life data collected over a long time period. The accuracy of the model could potentially be increased if certain features left out of the study are included in future training data, such as handwriting. A similar approach could also be used with audio recordings of speech, which includes pauses that aren’t represented in written language.
According to Royyuru, the advantage of using language samples is that, regardless of whether the samples are spoken or written, they are noninvasive methods of ascertaining people’s cognitive conditions. Collecting language data can be done remotely and relatively cheaply by leveraging the internet, although it’s important that privacy safeguards and informed consent are in place when collecting such data.
Co-author on the study and researcher for neuroimaging and computational psychiatry at IBM, Guillermo Cecchi, explained to Scientific American that the process is being adapted to understand other forms of disease as well:
“We are in the process of leveraging this technology to better understand diseases such as schizophrenia, [amyotrophic lateral sclerosis] and Parkinson's disease and are doing so in prospective studies [that] analyze spoken speech samples, given with consent from similar cognitive verbal tests.”
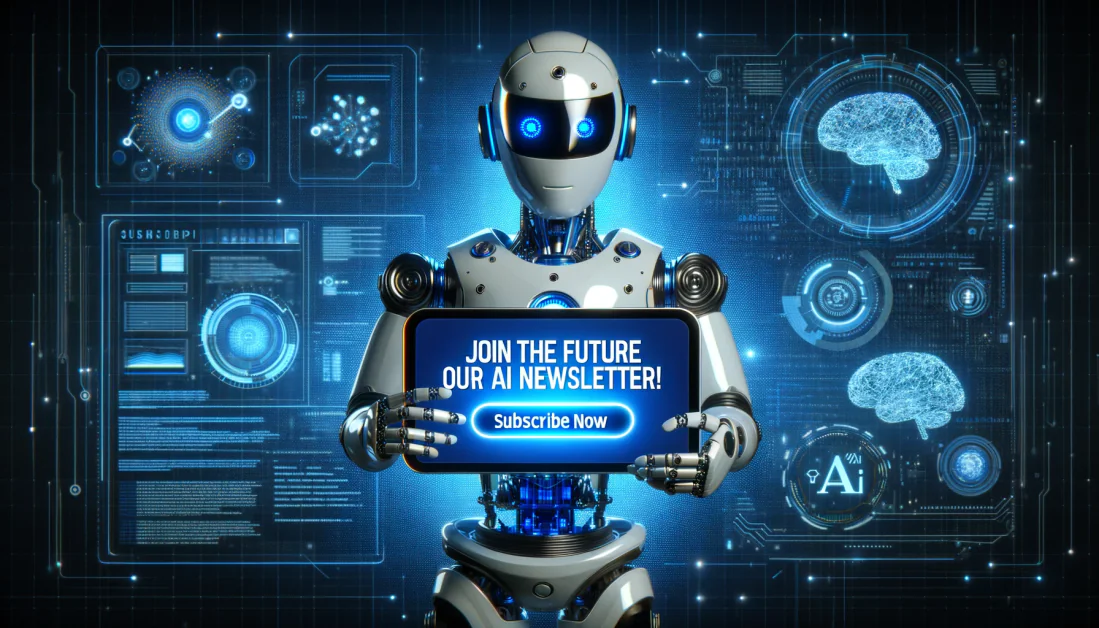
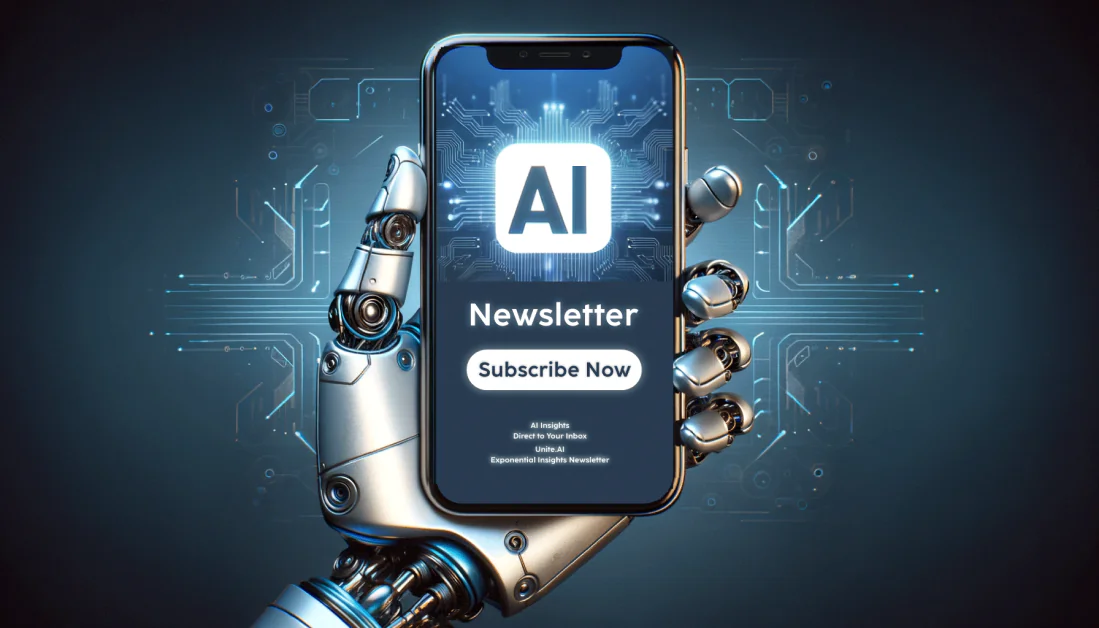