Artificial Intelligence
AI Models Help Predict Large Tropical Waves and Ocean Currents
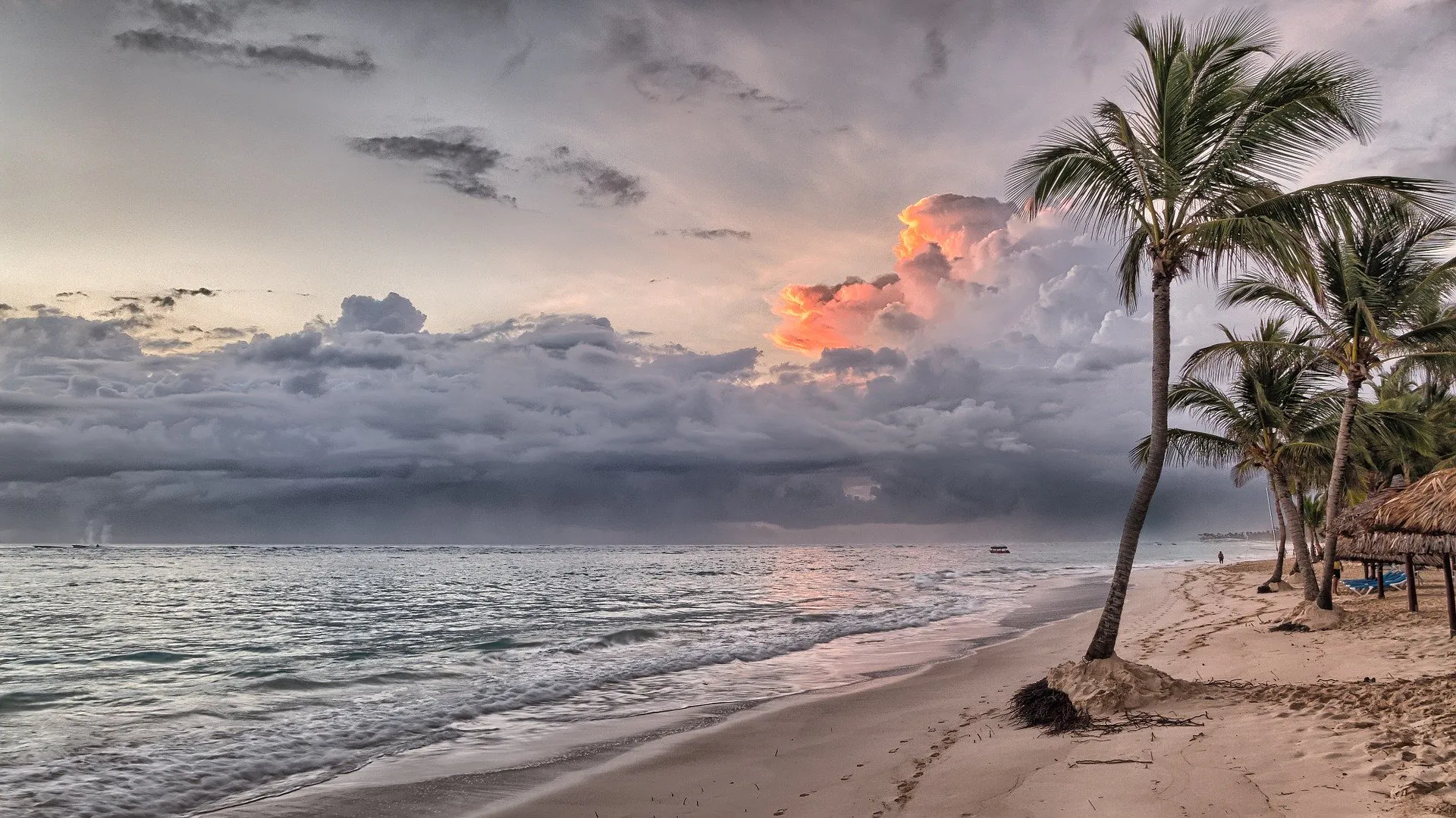
A team of researchers has recently designed an AI model that is capable of forecasting oceanic phenomena that stretch over hundreds of miles/kilometers, like tropical instability waves (TIW). Tropical Instability Waves (TIW) are an oceanic event that takes place within the Pacific Ocean, near the equator. The Pacific TIW involves the motion of curved, triangular waves that move westward along the edges of the tropic pacific cold tongue – a region of the tropics notably colder than the ocean surrounding it.
The environmental factors that give rise to TIW are extraordinarily complex and the phenomenon is hard to forecast. Forecasting the TIW is traditionally done with complex statistical and physical models. However, a team of researchers has recently designed an AI model intended to better predict TIWs and other ocean phenomena.
According to Phys.org, the research team was headed by the Institute of Oceanology of the Chinese Academy of Sciences (IOCAS) Professor LI Xiaofeng, and the team also included members from various Chinese oceanic sciences divisions like Shanghai Ocean University and the Second Institute of Oceanology of Ministry of Natural Resources. The team made of use satellite data to design a deep learning model intended to analyze the instability waves as they move thousands of kilometers through the ocean. Even with global satellite data, the environmental factors that impact oceanic phenomena can be difficult to discern, but the goal is that AI models can do a better job of deciphering these variables and making predictions than traditional models.
The deep learning model that the researchers designed made use of sea surface temperature data collected by satellites, analyzing current patterns and contrasting them with surface temperature data collected in years past. The researchers had the model trained and tested on approximately nine years of data. When the results were analyzed, the researchers found that the model was able to accurately and consistently forecast the change in sea surface temperature and predict temporal and spatial variations within TIW as a result.
The study implies that AI models supported by big datasets can be reliable ways of forecasting even some of the most complex phenomena in the oceans.
“AI-based models, statistical models, and traditional numerical models can complement each other and provide a novel perspective for studying complicated oceanic features,” LI Xiaofeng explained according to Phys.
It’s hoped that as the model is improved and refined it will assist in the prediction of large waves and storms, which has practical applications for ocean navigation systems and the prediction of severe weather events that may harm coastal cities. This type of research is particularly valuable in a world where climate change is altering how ocean currents move and interact with the environment around them. The research conducted by LI Xiaofeng and colleagues is part of a growing trend of using AI algorithms and satellite data to learn about and predict the movement of ocean currents and related phenomena.
As another example of AI being used to track and predict oceanic phenomena, earlier this year a team of researchers from the Plymouth Marine Laboratory and the University of the Aegean published a study examining how machine learning algorithms and satellite data can be used to identify areas of concentrated plastic waste and track its spread.
The team took satellite images of plastic refuse and trained an image identification system on them, aiming to see if the system could accurately discern patches of plastic trash from wood, seaweed, and other natural floating objects. According to the results of the study, the algorithm was able to correctly identify garbage approximately 86% of the time. The researchers want to improve upon the model and create a system that could facilitate the identification and cleanup of plastic waste along coastlines and rivers.
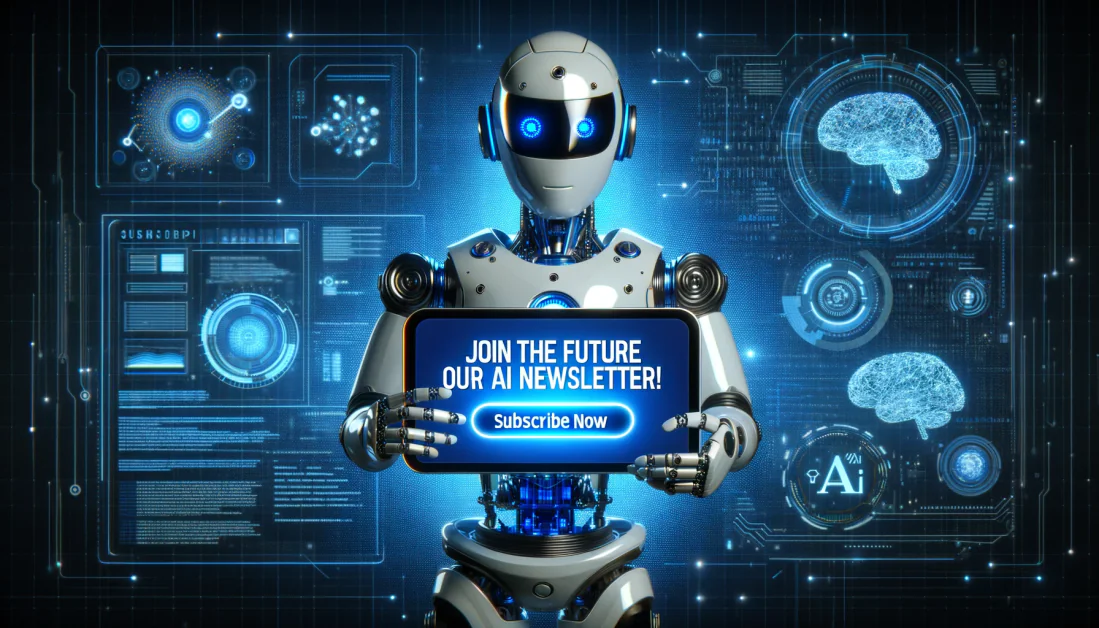
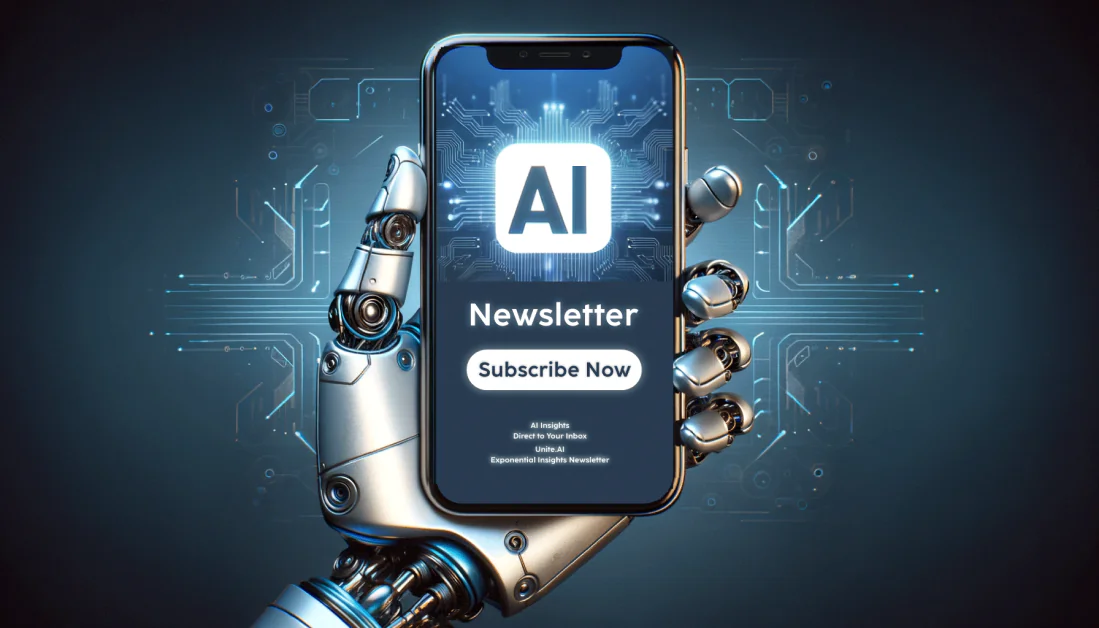