Artificial Intelligence
Researchers Imitate Brain Neurons Using Semiconductor MaterialÂ
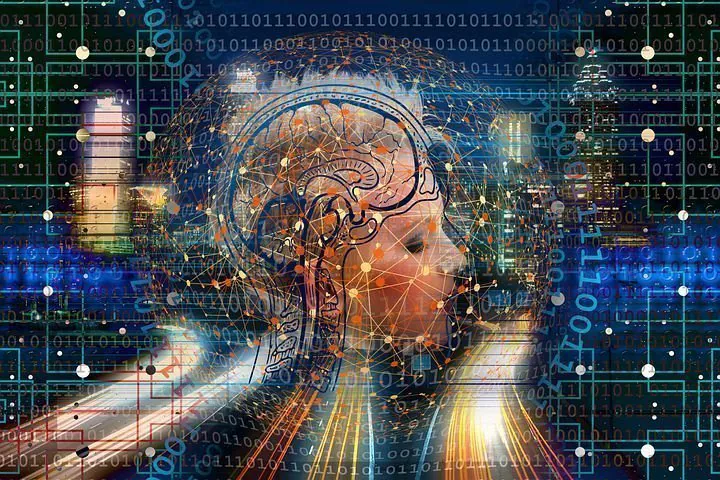
Computer chips are one of the most important aspects of artificial intelligence (AI). The powerful little pieces are foundational to automatic image recognition and are partly responsible for teaching robots how to do certain activities like walk. With the increasing potential of AI technology, todayâs computer chips are required to be both extremely powerful and economical, but this is a difficult thing to accomplish.Â
Since conventional microelectronics can only be optimized so much due to physical limitations, researchers have turned to the human brain, as they often do, for inspiration on how to process and store information more efficiently.Â
Scientists at TU Dresden and the Helmholtz-Zentrum Dresden-Rossendorf (HZDR) have successfully imitated the workings of brain neurons through the use of semiconductor materials, for the first time ever.
The research was published in the journal Nature Electronics.Â
The work was done by three primary authors, including HZDR physicist Larysa Baraban, and it was an international collaboration between six institutions.Â
Todayâs Microelectronics vs the Artificial Neuron
The technique most often used today to improve the performance of microelectronics is by reducing component size. In the case of silicon computer chips, this reduction takes place for the individual transistors.
According to Baraban, âThat canât go on indefinitely — we need new approaches.âÂ
The researchers set out to mimic the brain and create an artificial neuron that could combine data processing and data storage.
âOur group has extensive experience with biological and chemical electronic sensors, âBarbara says. âSo we simulated the properties of neurons using the principles of biosensors and modified a classical field-effect transistor to create an artificial neuron transistor.â
This approach allows there to be simultaneous storage and information processing, all within one single component. In the transistor technology that is most used today, these two processes are separated, resulting in slower processing times and performance limitations.
The Human Brain
Researchers have been working on constructing computers based on the human brain for many years, but much of it has been unsuccessful. Some of the first attempts involved nerve cells being linked to electronics in Petri dishes, but as Gianaurelio Cuniberti puts it, who is Professor for Materials Science and Nanotechnology at TU Dresden, âa wet computer chip that has to be fed all the time is of no use to anybody.â
The team of researchers was successful in implementing the neurotransistor.Â
âWe apply a viscous substance – called solgel – to a conventional silicon wafer with circuits. This polymer hardens and becomes a porous ceramic,â says Cuniberti. âIons move between the holes. They are heavier than electrons and slower to return to their position after excitation. This delay, called hysteresis, is what causes the storage effect. The more an individual transistor is excited, the sooner it will open and let the current flow. This strengthens the connection. The system is learning.â
According to the team, the chip will be less precise and would estimate mathematical computations, compared to calculating them down to the last decimal.
âBut they would be more intelligent,â Cuniberti says. âFor example, a robot with such processors would learn to walk or grasp; it would possess an optical system and learn to recognize connections. And all this without having to develop any software.âÂ
One of the other major benefits of this type of computer is that the plasticity allows it to make changes and adapt during operation. Much like the human brain, this means the computer can end up encountering and solving problems that it was never programmed to begin with.