Artificial Intelligence
AI-Generated Language Is Beginning to Pollute Scientific Literature
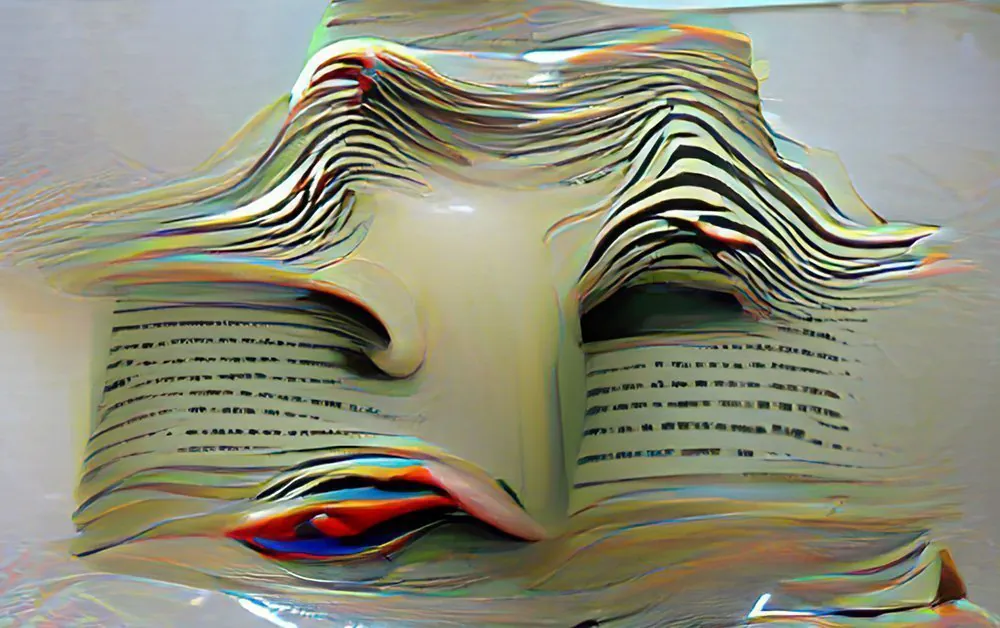
Researchers from France and Russia have published a study indicating that the use of AI-driven probabilistic text generators such as GPT-3 are introducing ‘tortured language', citations of non-existent literature and ad hoc, uncredited image reuse into previously respectable channels for the publication of new scientific literature.
Perhaps of most concern is that the papers studied also contain scientifically inaccurate or non-reproducible content presented as the fruits of objective and systematic research, indicating that generative language models are being used not only to bolster the limited English skills of the papers' authors, but actually to do the hard work involved (and, invariably, to do it badly).
The report, titled Tortured phrases: A dubious writing style emerging in science, has been compiled by researchers from the Computer Science Department at the University of Toulouse and Yandex researcher Alexander Magazinov, currently at Tel Aviv University.
The study focuses particularly on the growth of nonsensical AI-generated scientific publications at the Elsevier Journal Microprocessors and Microsystems.
By Any Other Name
Autoregressive language models such as GPT-3 are trained on high volumes of data, and are designed to paraphrase, summarize, collate and interpret that contributing data into cohesive generative language models that are capable of reproducing natural speech and writing patterns, while retaining the original intention of the training data.
Since such frameworks are frequently punished at the model training stage for offering direct and ‘non-absorbed' regurgitation of the original data, they inevitably seek synonyms – even for well-established phrases.
The apparently AI-created/aided scientific submissions unearthed by the researchers include an extraordinary number of failed attempts at creative synonyms for known phrases in the machine learning sector:
deep neural network: ‘profound neural organization'
artificial neural network: ‘(fake | counterfeit) neural organization'
mobile network: ‘versatile organization'
network attack: ‘organization (ambush | assault)'
network connection: ‘organization association'
big data: ‘(enormous | huge | immense | colossal) information'
data warehouse: ‘information (stockroom | distribution center)'
artificial intelligence (AI): ‘(counterfeit | human-made) consciousness'
high performance computing: ‘elite figuring'
fog/mist/cloud computing: ‘haze figuring'
graphics processing unit (GPU): ‘designs preparing unit'
central processing unit (CPU): ‘focal preparing unit'
workflow engine: ‘work process motor'
face recognition: ‘facial acknowledgement'
voice recognition: ‘discourse acknowledgement'
mean square error: ‘mean square (mistake | blunder)'
mean absolute error: ‘mean (outright | supreme) (mistake | blunder)'
signal to noise: ‘(motion | flag | indicator | sign | signal) to (clamor | commotion | noise)'
global parameters: ‘worldwide parameters'
random access: ‘(arbitrary | irregular) get right of passage to'
random forest: ‘(arbitrary | irregular) (backwoods | timberland | lush territory)'
random value: ‘(arbitrary | irregular) esteem'
ant colony: ‘subterranean insect (state | province | area | region | settlement)'
ant colony: ‘underground creepy crawly (state | province | area | region | settlement)'
remaining energy: ‘leftover vitality'
kinetic energy: ‘motor vitality'
naïve Bayes: ‘(credulous | innocent | gullible) Bayes'
personal digital assistant (PDA): ‘individual computerized collaborator'
In May of 2021 the researchers queried the Dimensions academic search engine in search of this kind of mangled, automated language, taking care to exclude legitimate phrases such as ‘enormous information' (which is a valid phrase, and not a failed synonym for ‘big data'). At this point they observed that Microprocessors and Microsystems had the highest number of occurrences of mishandled paraphrasing.
At the current time, it's still possible to retrieve (archive snapshot, 15/07/2021) a number of scientific papers for the nonsense phrase ‘profound neural organization' (i.e. ‘deep neural network'), and others in the above list yield similar hits.
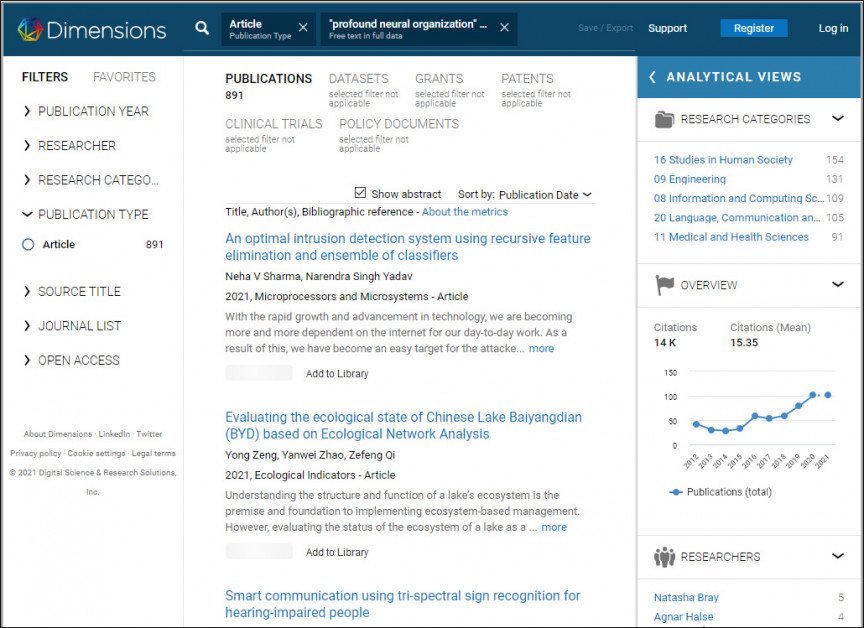
Search results for ‘profound neural organization' (‘deep neural network') at Dimensions. Source: https://app.dimensions.ai/
The Microprocessors journal was founded in 1976, and renamed to Microprocessors and Microsystems two years later.
A Growth of Nonsense Language
The researchers studied a period covering February 2018 to June of 2021, and observed a steep rise in the volume of submissions over the last two years, and particularly over the last 6-8 months:
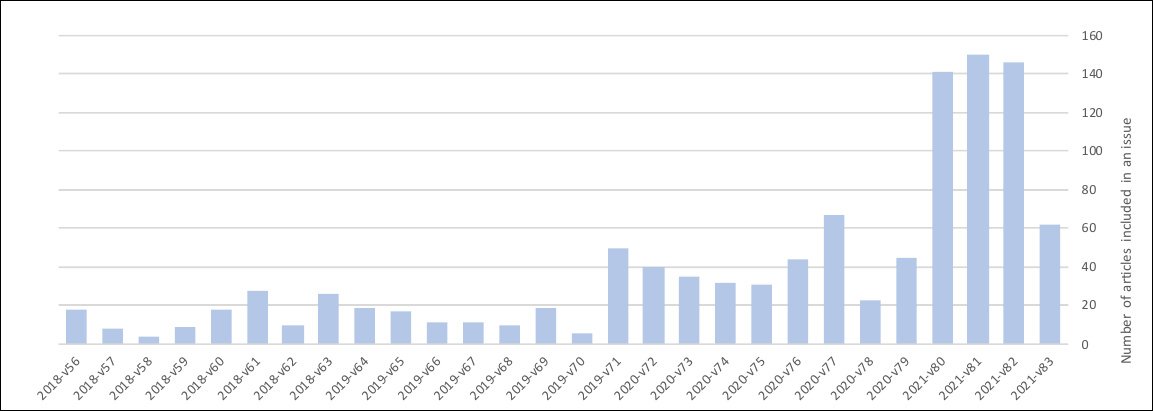
Correlation or causation? The rise in submissions to the Microprocessors and Microsystems journal seems to coincide with the growth of ‘nonsense' text and synonyms in apparently respectable submissions. Source: https://arxiv.org/pdf/2107.06751.pdf
The final dataset gathered by the collaborators contains 1,078 full length articles obtained via the Elsevier subscription of University of Toulouse.
Diminishing Editorial Oversight for Chinese Scientific Papers
The paper observes that the time period allotted for editorial assessment of the flagged submissions becomes radically shortened in 2021, falling to below 40 days; a six-fold decrease in the standard time for peer review, evident from February of 2021.
The greatest number of flagged papers originate from authors with affiliations to mainland China: out of 404 papers accepted in under 30 days, 97.5% are China-related. Conversely, in cases where the editorial process exceeded 40 days (615 papers), China-affiliated submissions represented only 9.5% of that category – a tenfold imbalance.
The report ascribes the infiltration of the flagged papers to shortcomings in the editorial process, and a possible lack of resources in the face of a growing number of submissions.
The researchers hypothesize that GPT-style generative models, and similar types of language generation frameworks, have been used to produce much of the text in the flagged papers; however, the way that a generative model abstracts its sources makes this difficult to prove, and the chief evidence lies in a common-sense evaluation of poor and unnecessary synonyms, and a meticulous examination of the submission's logical coherence.
The researchers further observe that the generative language models which they believe to be contributing to this flood of nonsense is capable not only of creating the problematic texts, but also recognizing them and flagging them systematically, in the same way that the researchers themselves have carried out manually. The work details such an implementation, using GPT-2, and offers a framework for future systems to identify problematic scientific submissions.
The incidence of ‘polluted' submissions is far higher in the Elsevier journal (72.1%) compared to other journals studied (13.6% maximum).
Not Just Semantics
The researchers emphasize that many of the journals in question are not merely using the wrong language, but contain scientifically inaccurate statements, indicating the possibility that generative language models are not just being used to improve the limited language skills of contributing scientists, but may actually be being used to formulate at least some of the core theorems and data in the paper.
In other cases the researchers posit an effective ‘resynthesis' or ‘spinning' of abstracted (and superior) prior work, in order to meet the pressures of ‘publish or perish' academic research cultures, and possibly to improve national rankings for global pre-eminence in AI research, through sheer volume.

Nonsensical content in a submitted paper. In this case, the researchers found that the text has been derived, ad hoc, from an EDN article, from where the accompanying illustration is also pilfered without attribution. The re-writing of the original content is so extreme as to render it meaningless.
Analyzing several of the submitted Elsevier papers, the researchers found sentences for which they failed to infer any meaning; references to non-existent literature; references to variables and theorems in formulae that did not actually appear in the supporting material (suggesting language-based abstraction, or ‘hallucination‘ of apparently factual data); and reuse of images with no acknowledgement of their sources (which the researchers criticize not from a copyright standpoint, but rather as an indicator of inadequate scientific rigor).
Citation Failures
The citations intended to support the arguments in a scientific paper were found in many of the flagged examples to be ‘either broken or leading to unrelated publications'.
Additionally, references to ‘related work' apparently often include authors that the researchers believe to have been ‘hallucinated' by a GPT-style system.
Wandering Attention
Another shortcoming of even state-of-the-art language models such as GPT-3 is their tendency to lose focus over a long discourse. The researchers found that the flagged papers often bring up a topic early in the paper which is actually never returned to after it is initially broached in preliminary notes or elsewhere.
They also theorize that some of the worst examples occur through multiple journeys of source text through a series of translation engines, each one distorting the meaning further.
Sources and Reasons
In attempting to discern what is behind this phenomenon, the paper's authors suggest a number of possibilities: that content from paper mills are being used as source material, introducing inaccuracies very early in a process that will inevitably produce further inaccuracies; that article spinning tools such as Spinbot are being used to mask plagiarism; and that the overwhelming pressure to publish regularly is leading under-resourced researchers to use GPT-3-style systems to either augment or entirely generate new academic papers.
The researchers close with a call to action for greater oversight and improved standards in an area of academic publishing which is proving, apparently, to become fodder for its own subject matter – machine learning systems. They also adjure Elsevier and other publishers to introduce more rigorous screening and review procedures, and broadly criticize current standards and practices in this regard, suggesting that ‘Deception with synthetic texts threatens the integrity of the scientific literature.'
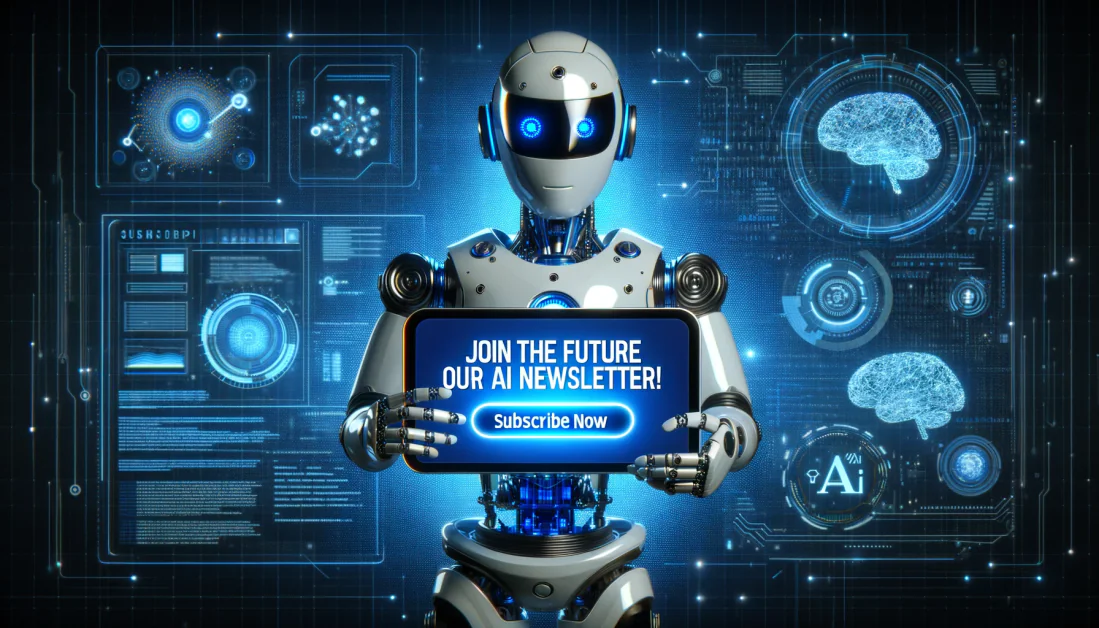
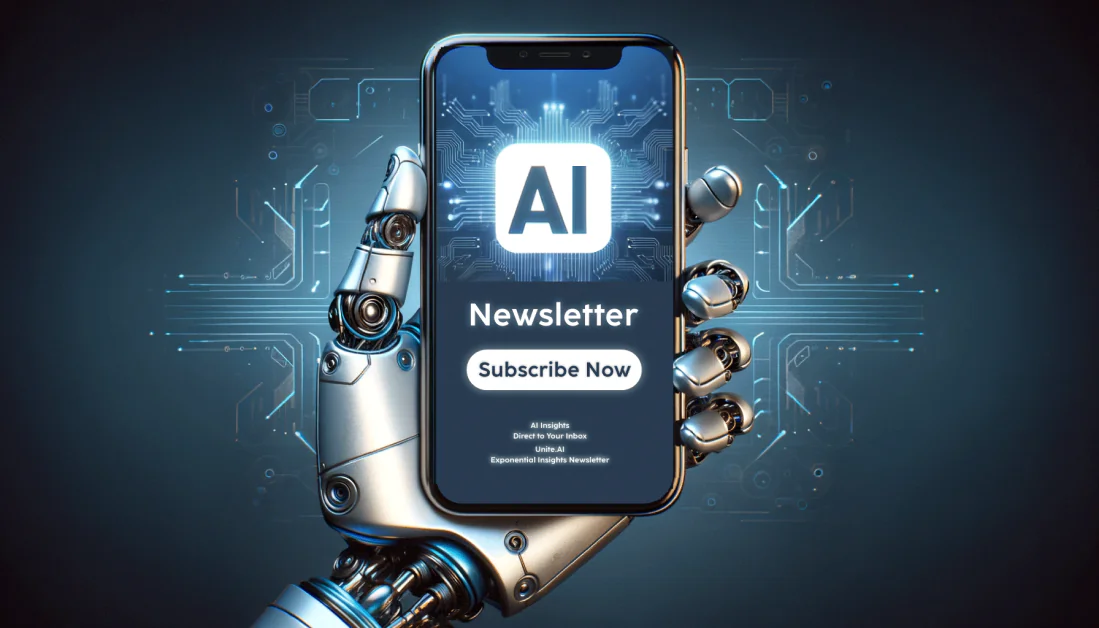