Interviews
Vikrant Tomar, CTO and Founder of Fluent.ai – Interview Series
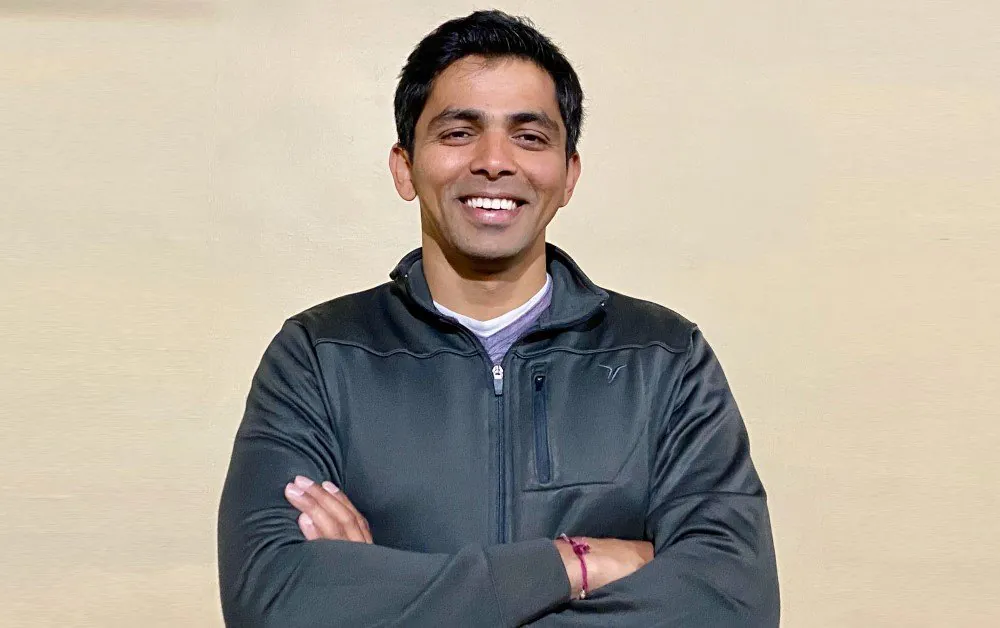
Vikrant Tomar, is the CTO and Founder of Fluent.ai, a speech understanding and voice user interface software for device OEMs and service providers.
What initially attracted you to studying acoustic modeling for speech recognition?
Really, being able to talk to the devices in the same manner we talk to another human being. This vision has been fascinating for me. I started studying speech recognition during the final year of my undergraduate degree. This is also when I started becoming interested in research, so I took a speech recognition course and a related research project. I was able to publish a research paper in InterSpeech conference, one of the largest and most reputed speech recognition conference, from this work. All this motivated me to choose research in speech recognition as a focus for the long term, hence the PhD.
In 2015 you launched Fluent.ai, could you share the genesis story behind this startup?
I have had an entrepreneurial yearning in me for a long time. I, along with two other friends, had attempted to start a company after our undergraduate degree, however, for a few reasons, that effort did not succeed. During my PhD at McGill, I kept an eye on Montreal’s startup scene. During this time, I also happened to get in touch with people from TandemLaunch – the startup foundry where I created Fluent.ai. By this time, I was towards the end of my PhD, and I was giving trying my hand at entrepreneurship again a serious thought. Through my work experience, research and association with other speech research groups, I realized that most of these experiences had been focused on doing speech recognition in a particular way: going from speech to text transcription and then natural language processing. However, this left a gap in the usability. A large portion of population cannot benefit from speech solutions developed in this manner. The amount of data required for such methods is so large that it would not make financial sense to develop separate models for languages with fewer speakers. Furthermore, many dialects and languages have no distinct written form. Even my own family was not able to use tools developed by me (they speak a dialect of Hindi). Considering all this, I started thinking about different ways of creating speech models, where the amount of data required was less, and/or the end-user could themselves train or update the models. I was aware of the work done at KU Leuven University (KUL) that could fit some of these requirements. With part of the technology coming from KUL, we were able to take the first steps toward what Fluent is today.
Could you elaborate on Fluent.ai’s intuitive speech understanding solutions?
Fluent.ai’s speech recognition solutions are inspired by how humans acquire and recognize languages. Conventional speech recognition systems first transcribe the input speech into text, and then extract meaning from that text. This is not how humans recognize speech. Take an example of kids before they learn to read and write: despite not knowing anything about the written representation of languages, they are able to have a spoken conversation with ease. In a similar manner, Fluent’s deep neural networks-based models are capable of directly extracting the meaning out of speech sounds without having to first transcribe that into a text. Technically, this is true Spoken Language Understanding. There are multiple advantages of this approach. Traditional speech recognition is a cumbersome approach, where several modules that are trained disjointly are weaved together to provide a final response. This results in a non-optimal solution that suffers from variations in results for accents, noise, background conditions etc. Fluent’s automatic intent recognition (AIR) system is end-to-end optimized; it’s entirely a neural network-based architecture, where all the modules are trained jointly to provide the most optimal solution. In addition, we are able to remove a number of computationally heavy modules commonly present in the conventional speech recognition system. This allows us to create low-footprint speech recognition systems that can run in as little as 40KB of RAM on a low-power microcontroller running at 50 MHz. Finally, our spoken language understanding based AIR systems are able exploit similarities between different languages in a unique way to provide unparalleled features such as the ability to recognize multiple languages in the same model.
What are some of the AI challenges behind overcoming the ambient noise problem?
Noise is one of the biggest challenges for speech recognition. What makes it a really challenging problem is that there are many different types of noise and they affect the spectrum of speech in different ways. Sometimes noise can also have an impact on the microphone response. In many cases, it is not possible to separate the speech sources from the noise sources. In some cases, noise results in masking the information available in speech spectrum, whereas in others, it can completely remove the useful information. Both result in low accuracy. While it is easy to remove consistent noise types, such as fan noise, some noise types, such as babble or people talking in the background or music, are very difficult to remove because how they affect the speech spectrum.
Could you define what Edge AI is and how Fluent.ai is using this type of AI?
Edge AI is an umbrella term used to cover a number of different ways in which AI applications could be moved to low-power devices. More and more this term is used for the cases where the edge devices are performing certain intelligent calculations themselves. At Fluent, we are focused on bringing high quality spoken language understanding to the edge. We have developed efficient algorithms that allows low-power compute devices to recognize the input speech themselves without having to send the data to a cloud-based server for processing. The advantages are twofold: first, the user’s privacy is not compromised by streaming and storing their voice data to the cloud. Second, such approach reduces latency because the speech data and the response does not have to travel between the cloud server and the device.
What other types of machine learning technologies are being used?
Our primary focus is on deep-learning based approaches for speech recognition. We are using RL (reinforcement learning) methods, e.g., NASIL[1], to discover new, previously unknown AI model architectures (so AI creating AI in some sense). And we are using AutoML to tune our predetermined AI models to achieve reliable results for different applications, thus increasing reliability and reproducibility. Model compression and other mathematical approaches further help optimize the model performance.
What do you see happening in the next 5 years for both natural language understanding and natural language processing?
I think the systems will evolve to provide more natural interactions. Despite the progress in the recent years, most current systems can either only answer simple queries or perform a voice activated internet search. We will see more and more solutions that can reason and answer a complete query for a person instead of merely functioning as a glorified voice-based search engine.
The other interested aspect is privacy. Current popular solutions are primarily internet connected devices that stream all of a user’s voice data to a cloud server. However, privacy of such solutions is becoming an issue. We are also starting to see the applications of voice UI beyond consumer electronics in industrial settings, in professional audio space, as well as, in hospitality and conference rooms. A key requirement for these applications is privacy, therefore current connected solutions do not suffice – so we will see a lot more edge AI or on-device natural language solutions.
As I mentioned earlier, speech and natural language solutions remain inaccessible to a large portion of worldwide population. There is significant amount of work going to creating new kind of AI models that can train with small amount of data resulting in reduced development costs, and inturn enabling development of models in languages with fewer speakers. Along the same line, we will see solutions that can learn to recognize multiple languages in the same model. Overall, we will see more and more deployment of multilingual AI models that can answer a user’s query in their native language.
Is there anything else that you would like to share about Fluent.ai?
Speech technology has come a long way in the last few years, and has a great deal of growth potential on the road ahead. At Fluent.ai, we’re always searching for new use cases of our existing technology while continuously innovating internally. The COVID-19 pandemic has created a heightened sensitivity to high-touch areas, such as elevator buttons, kiosks in restaurants and more, which sparked a new demand for voice enabled technology. Fluent.ai hopes to help fill those gaps, as our solutions are multilingual, and therefore more inclusive, and operate offline, offering an additional layer of privacy. These functions, as mentioned, are likely going to be the future of speech technology.
Thank you for the great interview, readers who wish to learn more should visit r of Fluent.ai.
[1] https://www.researchgate.net/profile/Farzaneh_Sheikhnezhad_Fard/publication/341083699_Nasil_Neural_Archit
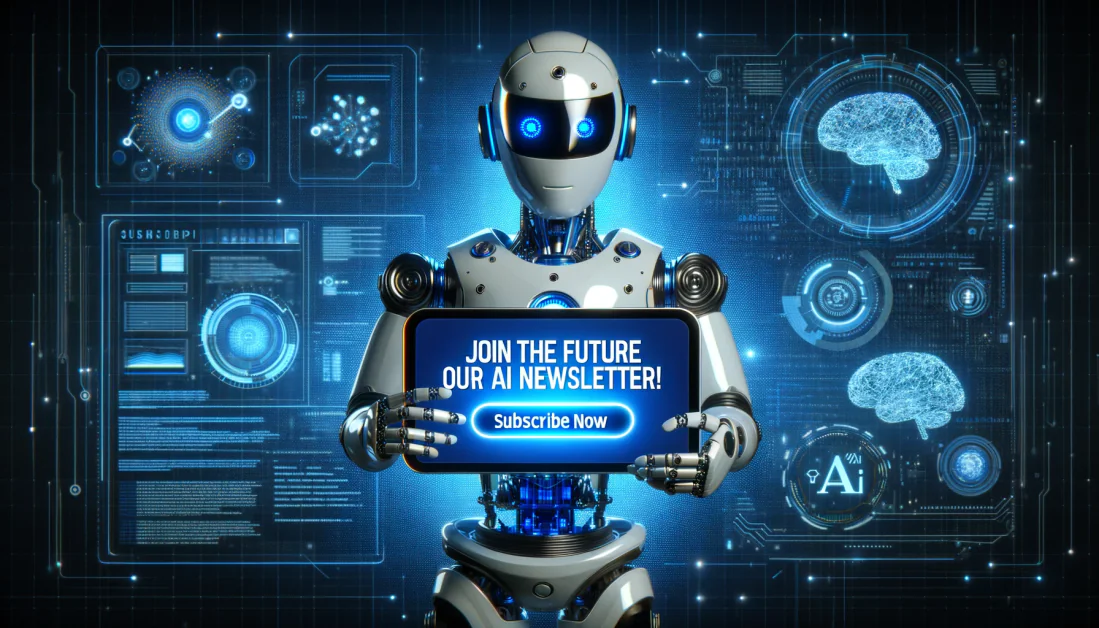
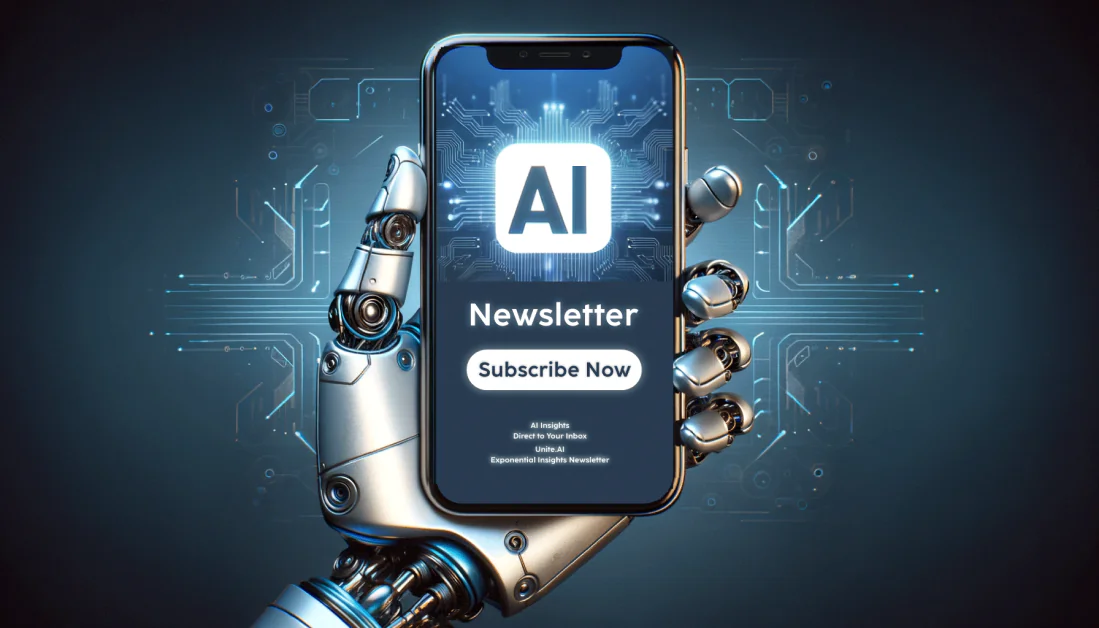