Artificial Intelligence
Segment Anything Model – Computer Vision Gets A Massive Boost
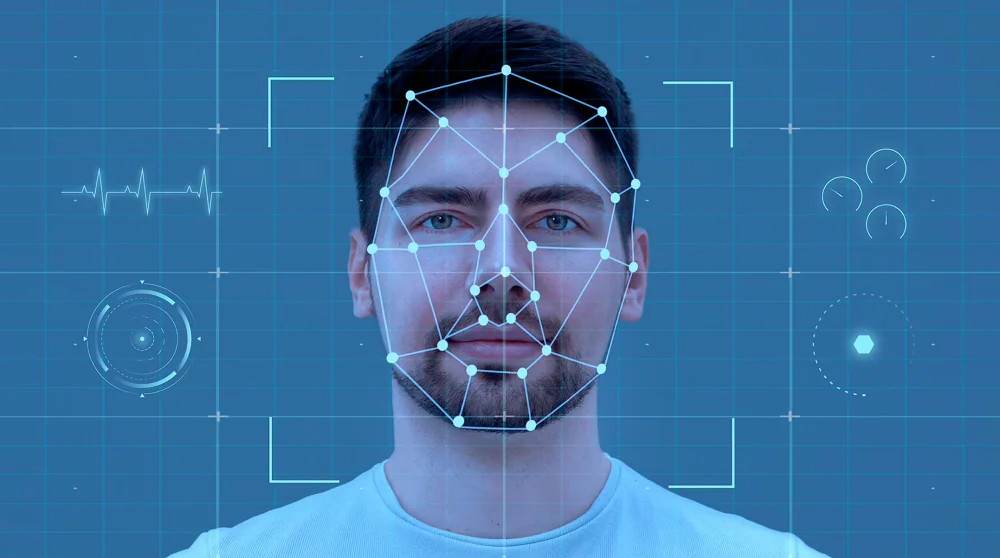
Computer vision (CV) has reached 99% accuracy from 50% within 10 years. The technology is expected to improve further to an unprecedented level with modern algorithms and image segmentation techniques. Recently, Meta's FAIR lab has released the Segment Anything Model (SAM) – a game-changer in image segmentation. This advanced model can produce detailed object masks from input prompts, taking computer vision to new heights. It can potentially revolutionize how we interact with digital technology in this era.
Let's explore image segmentation and briefly uncover how SAM impacts computer vision.
What is Image Segmentation & What Are its Types?
Image segmentation is a process in computer vision that divides an image into multiple regions or segments, each representing a different object or area of the image. This approach allows experts to isolate specific parts of an image to obtain meaningful insights.
lmage segmentation models are trained to improve output by recognizing important image details and reducing complexity. These algorithms effectively differentiate between different regions of an image based on features such as color, texture, contrast, shadows, and edges.
By segmenting an image, we can focus our analysis on the regions of interest for insightful details. Below are different image segmentation techniques.
- Semantic segmentation involves labeling pixels into semantic classes.
- Instance segmentation goes further by detecting and delineating each object in an image.
- Panoptic segmentation assigns unique instance IDs to individual object pixels, resulting in more comprehensive and contextual labeling of all objects in an image.
Segmentation is implemented using image-based deep learning models. These models fetch all the valuable data points and features from the training set. Then, turn this data into vectors and matrices to understand complex features. Some of the widely used deep learning models behind image segmentation are:
- Convolutional Neural Networks (CNNs)
- Fully Connected Networks (FCNs)
- Recurrent Neural Networks (RNNs)
How Image Segmentation Works?
In computer vision, most image segmentation models consist of an encoder-decoder network. The encoder encodes a latent space representation of the input data which the decoder decodes to form segment maps, or in other words, maps outlining each object’s location in the image.
Usually, the segmentation process consists of 3 stages:
- An image encoder that transforms the input image into a mathematical model (vectors and matrices) for processing.
- The encoder aggregates the vectors at multiple levels.
- A fast mask decoder takes the image embeddings as input and produces a mask that outlines different objects in the image separately.
The State of Image Segmentation
Starting in 2014, a wave of deep learning-based segmentation algorithms emerged, such as CNN+CRF and FCN, which made significant progress in the field. 2015 saw the rise of the U-Net and Deconvolution Network, improving the accuracy of the segmentation results.
Then in 2016, Instance Aware Segmentation, V-Net, and RefineNet further improved the accuracy and speed of segmentation. By 2017, Mark-RCNN and FC-DenseNet introduced object detection and dense prediction to segmentation tasks.
In 2018, Panoptic Segmentation, Mask-Lab, and Context Encoding Networks were at the center of the stage as these approaches addressed the need for instance-level segmentation. By 2019, Panoptic FPN, HRNet, and Criss-Cross Attention introduced new approaches for instance-level segmentation.
In 2020, the trend continued with the introduction of Detecto RS, Panoptic DeepLab, PolarMask, CenterMask, DC-NAS, and Efficient Net + NAS-FPN. Finally, in 2023, we have SAM, which we will discuss next.
Segment Anything Model (SAM) – General Purpose Image Segmentation
The Segment Anything Model (SAM) is a new approach that can perform interactive and automatic segmentation tasks in a single model. Previously, interactive segmentation allowed for segmenting any object class but required a person to guide the method by iteratively refining a mask.
Automatic segmentation in SAM allows the segmentation of specific object categories defined ahead of time. Its promotable interface makes it highly flexible. As a result, SAM can address a wide range of segmentation tasks using a suitable prompt, such as clicks, boxes, text, and more.
SAM is trained on a diverse and insightful dataset of over 1 billion masks, making it possible to recognize new objects and images unavailable in the training set. This modern framework will widely revolutionize the CV models in applications like self-driving cars, security, and augmented reality.
SAM can detect and segment objects around the car in self-driving cars, such as other vehicles, pedestrians, and traffic signs. In augmented reality, SAM can segment the real-world environment to place virtual objects in appropriate locations, creating a more realistic and engaging UX.
Image Segmentation Challenges in 2023
The increasing research and development in image segmentation also bring significant challenges. Some of the foremost image segmentation challenges in 2023 include the following:
- The increasing complexity of datasets, especially for 3D image segmentation
- The development of interpretable deep models
- The use of unsupervised learning models that minimize human intervention
- The need for real-time and memory-efficient models
- Eliminating the bottlenecks of 3D point-cloud segmentation
The Future of Computer Vision
The global computer vision market impacts multiple industries and is projected to reach over $41 billion by 2030. Modern image segmentation techniques like the Segment Anything Model coupled with other deep learning algorithms will further strengthen the fabric of computer vision in the digital landscape. Hence, we'll see more robust computer vision models and intelligent applications in the future.
To learn more about AI and ML, explore Unite.ai – your one-stop solution to all queries about tech and its modern state.
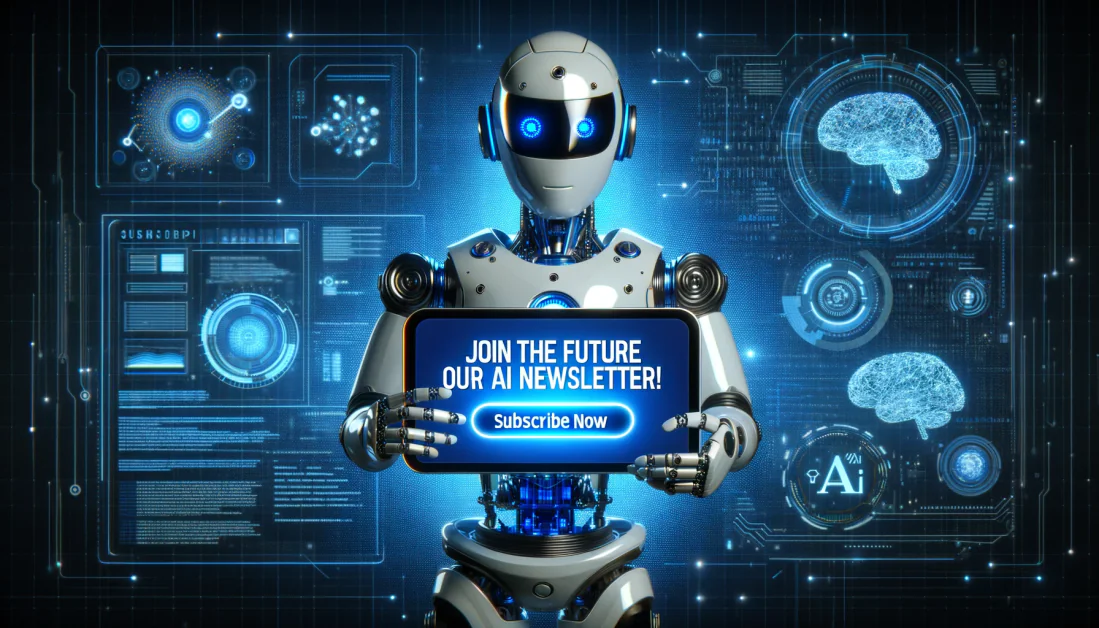
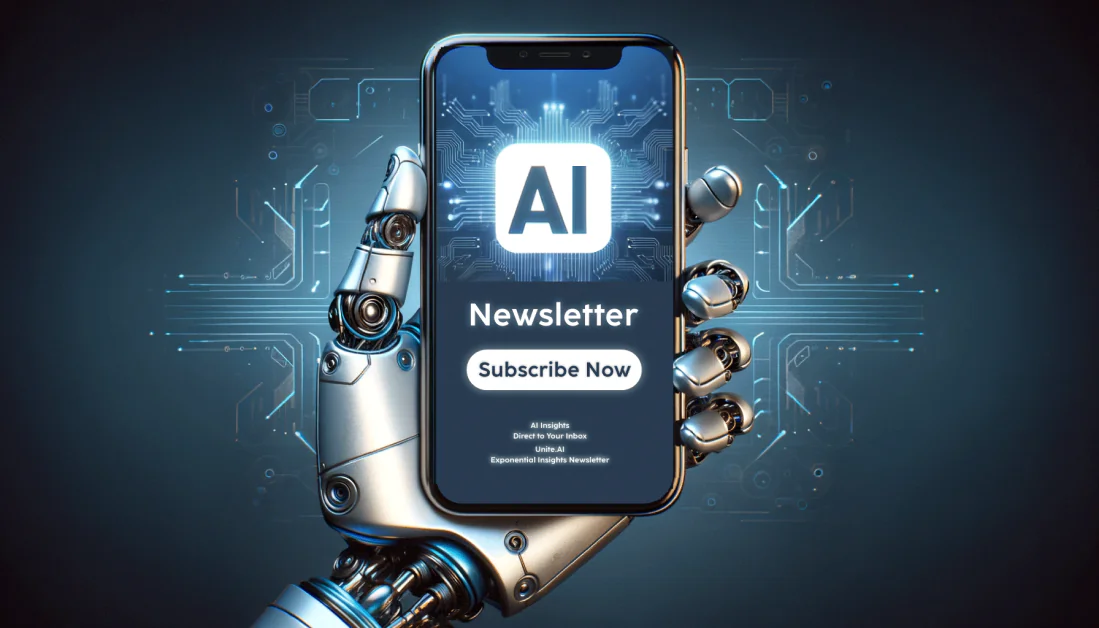