Brain Machine Interface
Researchers Use Generative Adversarial Networks to Improve Brain-Computer Interfaces
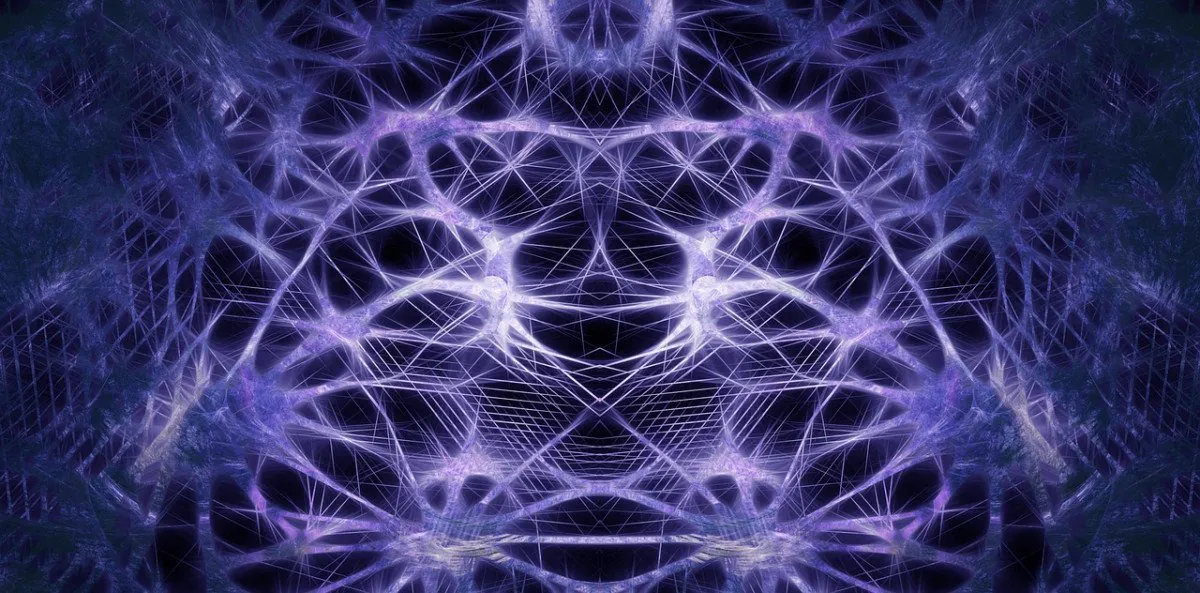
Researchers at the University of Southern California (USC) Viterbi School of Engineering are using generative adversarial networks (GANs) to improve brain-computer interfaces (BCIs) for people with disabilities.
GANs are also used to create deepfake videos and photo realistic human faces.
The research paper was published in Nature Biomedical Engineering.
The Power of BCIs
The team was able to teach an AI to generate synthetic brain activity data through this approach. That data is in the form of neural signals called spike trains, which can be fed into machine learning algorithms to improve BCIs among those with disabilities.
BCIs analyze an individual’s brain signals before translating the neural activity into commands, which enables the user to control digital devices with just their thoughts. These devices, which can include things like computer cursors, are able to improve the quality of life for patients suffering from motor dysfunction or paralysis. They can also benefit individuals with locked-in syndrome, which occurs when the person is unable to move or communicate despite being fully conscious.
There are many different types of BCIs already on the market, such as those that measure brain signals and devices that are implanted into brain tissues. The technology is constantly improving and being applied in new ways, including neurorehabilitation and depression treatment. However, it is still difficult to make the systems fast enough to operate efficiently in the real-world.
BCIs require massive amounts of neural data and long training periods, calibrations, and learning to understand their inputs.
Laurent Itti is a computer science professor and co-author of the research.
“Getting enough data for the algorithms that power BCIs can be difficult, expensive, or even impossible if paralyzed individuals are not able to produce sufficiently robust brain signals,” Itti said.
The technology is user-specific, meaning it has to be trained for each individual.
Generative Adversarial Networks
GANs can improve this entire process since they are capable of creating an unlimited amount of new, similar images by going through a trial-and-error process.
Shixian Wen, a Ph.D student advised by Itti and lead author of the study, decided to look at GANs and the possibility that they could create training data for BCIs by generating synthetic neurological data that is indistinguishable from the real counterpart.
The team carried out an experiment where they trained a deep-learning spike synthesizer with one session of data that was recorded from a monkey reaching for an object. They then used a synthesizer to generate a large amount of similar, but fake neural data.
The synthesized data was then combined with small amounts of new real data to train a BCI. With this approach, the system was able to get up and running much faster than current methods. More specifically, the GAN-synthesized neural data improved the BCIs overall training speed by up to 20 times.
“Less than a minute’s worth of real data combined with the synthetic data works as well as 20 minutes of real data,” Wen said.
“It is the first time we’ve seen AI generate the recipe for thought or movement via the creation of synthetic spike trains. This research is a critical step towards making BCIs more suitable for real-world use.”
Following the first experimental sessions, the system was able to adapt to new sessions with limited additional neural data.
“That’s the big innovation here — creating fake spike trains that look just like they come from this person as they imagine doing different motions, then also using this data to assist with learning on the next person,” Itti said.
These new developments with GAN-generated synthetic data could also lead to breakthroughs in other areas of the field.
“When a company is ready to start commercializing a robotic skeleton, robotic arm or speech synthesis system, they should look at this method, because it might help them with accelerating the training and retraining,” Itti said. “As for using GAN to improve brain-computer interfaces, I think this is only the beginning.”
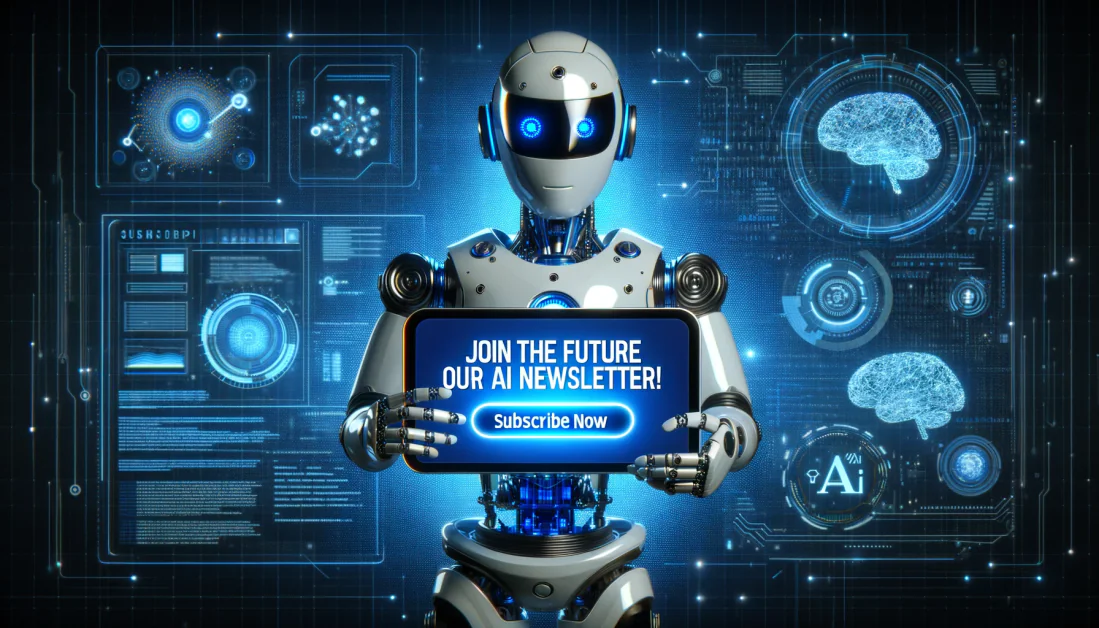
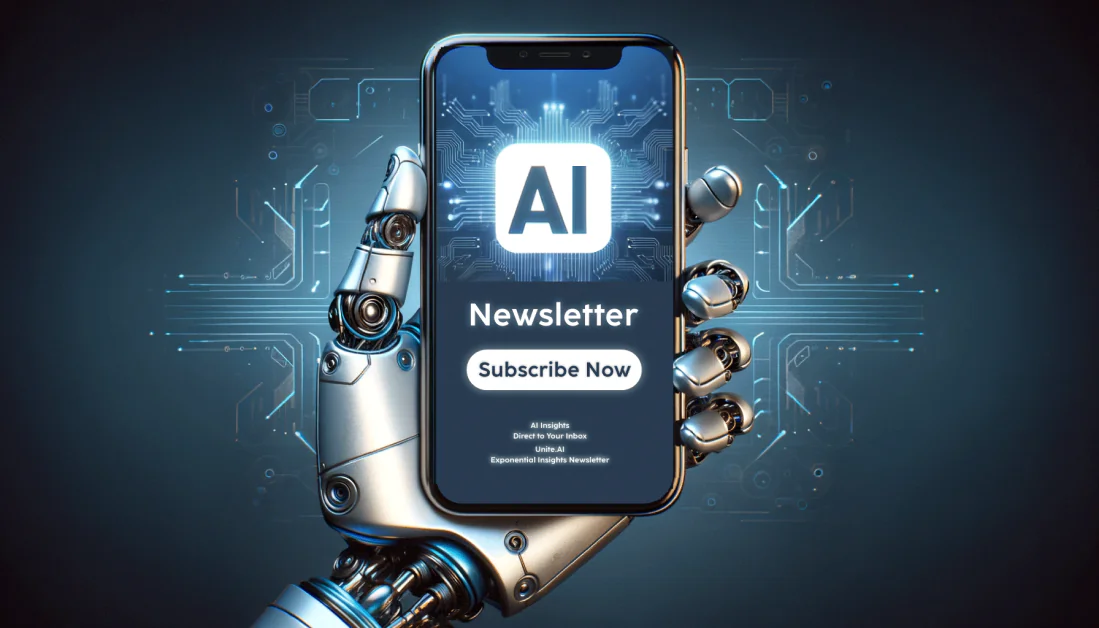