Artificial Intelligence
Researchers Use Deep Learning to Turn Landmark Photos 4D
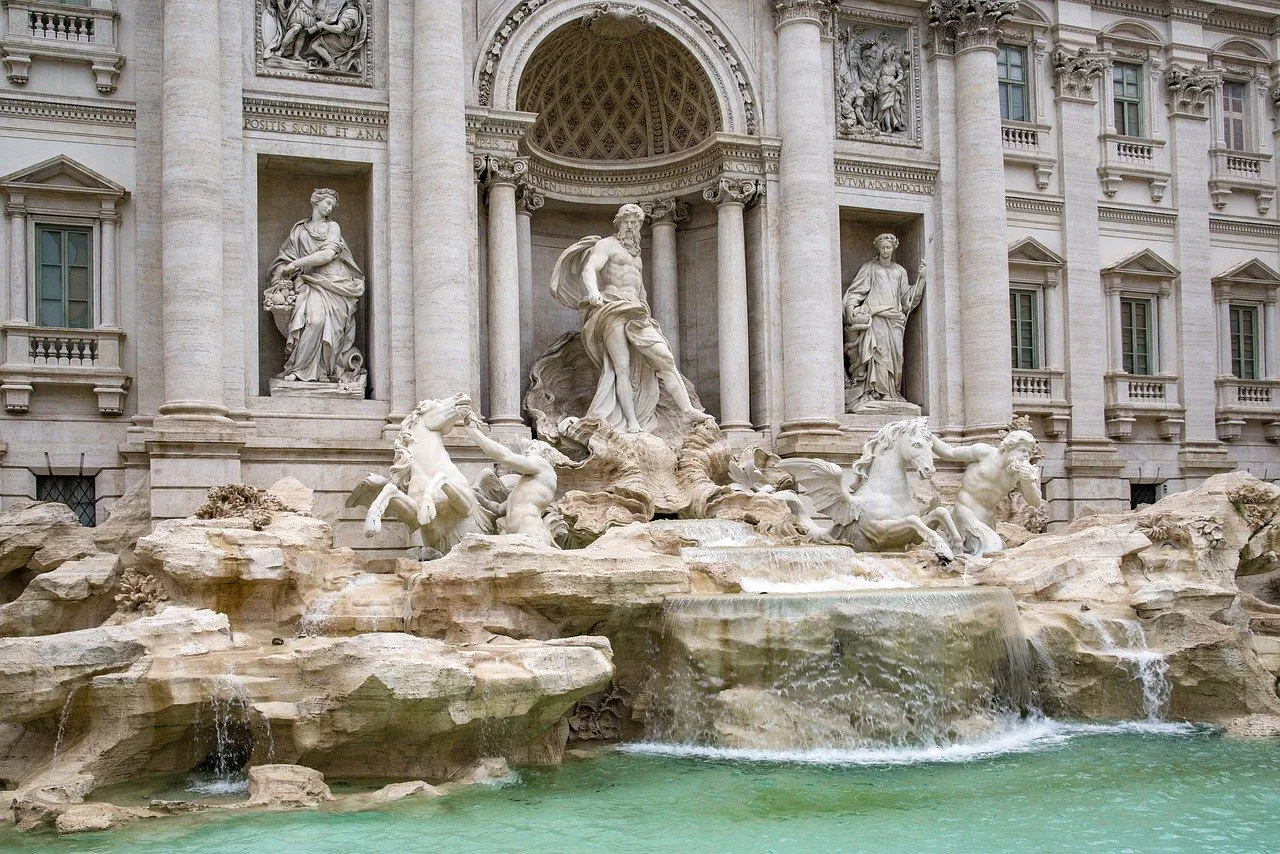
Researchers at Cornell University have developed a new method that utilizes deep learning in order to turn world landmark photos 4D. The team relied on publicly available tourist photos of major points like the Trevi Fountain in Rome, and the end results are 3D images that are maneuverable and can show changes in appearance over time.
The newly developed method takes in and synthesizes tens of thousands of untagged and undated photos, and it is a big step forward for computer vision.
The work is titled “Crowdsampling the Plenoptic Function,” and it was presented at the virtual European Conference on Computer Vision, which took place between Aug. 23-28.
Noah Snavely is an associate professor of computer science at Cornell Tech and senior author of the paper. Other contributors include Cornell doctoral student Zhengqi Li, first author of the paper, as well as Abe Davis, assistant professor of computer science in the Faculty of Computing and Information Science, and Cornell Tech doctoral student Wenqi Xian.
“It’s a new way of modeling scene that not only allows you to move your head and see, say, the fountain from different viewpoints, but also gives you controls for changing the time,” Snavely said.
“If you really went to the Trevi Fountain on your vacation, the way it would look would depend on what time you went — at night, it would be lit up by floodlights from the bottom. In the afternoon, it would be sunlit, unless you went on a cloudy day,” he continued. “We learned the whole range of appearances, based on time of day and weather, from these unorganized photo collections, such that you can explore the whole range and simultaneously move around the scene.”
Traditional Computer Vision Limitations
Since there can be so many different textures present that need to be reproduced, it is difficult for traditional computer vision to represent places accurately through photos.
“The real world is so diverse in its appearance and has different kinds of materials — shiny things, water, thin structures,” Snavely said.
Besides those barriers, traditional computer vision also struggles with inconsistent data. Plenoptic function is how something appears from every possible viewpoint in space and time, but in order to reproduce this, hundreds of webcams are required at the scene. Not only that, but they would have to be recording all throughout the day and night. This could be done, but it is an extremely resource-heavy task when looking at the number of scenes where this method would be required.
Learning from Other Photos
In order to get around this, the team of researchers developed the new method.
“There may not be a photo taken at 4 p.m. from this exact viewpoint in the data set. So we have to learn from a photo taken at 9. p.m. at one location, and a photo taken at 4:03 from another location,” said Snavely. “And we don’t know the granularity of when these photos were taken. But using deep learning allows us to infer what the scene would have looked like at any given time and place.”
A new scene representation called Deep Multiplane Images was introduced by the researchers in order to interpolate appearance in four dimensions, which are 3D and changes over time.
According to Snavely, “We use the same idea invented for creating 3D effects in 2D animation to create 3D effects in real-world scenes, to create this deep multilayer image by fitting it to all these disparate measurements from the tourists’ photos. It’s interesting that it kind of stems from this very old, classic technique used in animation.”
The study demonstrated that the trained model could create a scene with 50,000 publicly available images from various sites. The team believes that it could have implications in many areas, including computer vision research and virtual tourism.
“You can get the sense of really being there,” Snavely said. “It works surprisingly well for a range of scenes.”
The project received support from former Google CEO and philanthropist Eric Schmidt, as well as Wendt Schmidt.
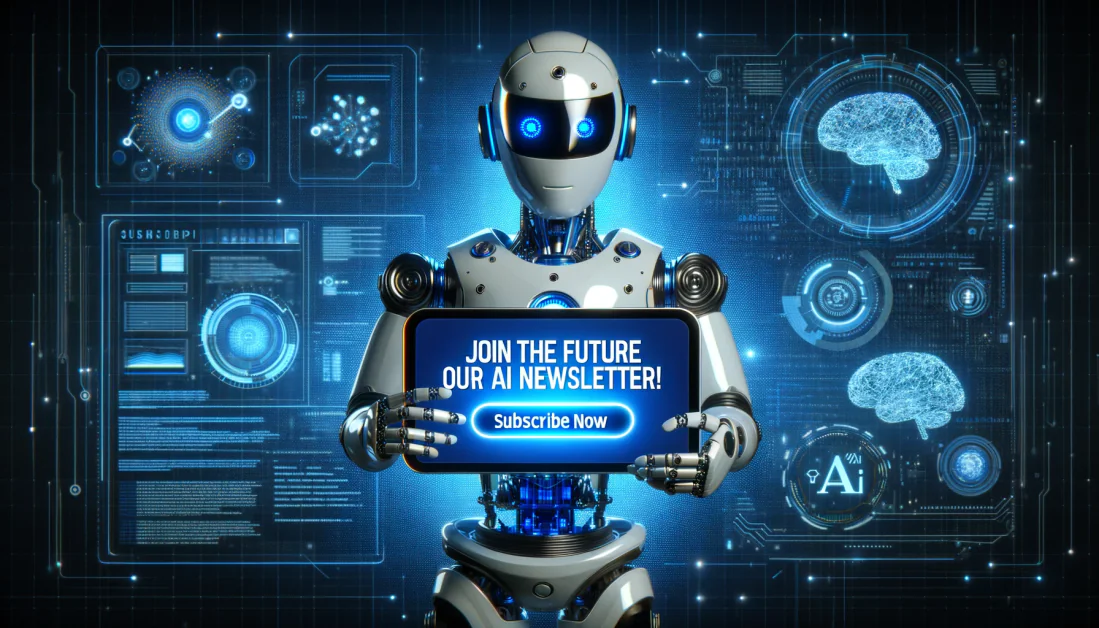
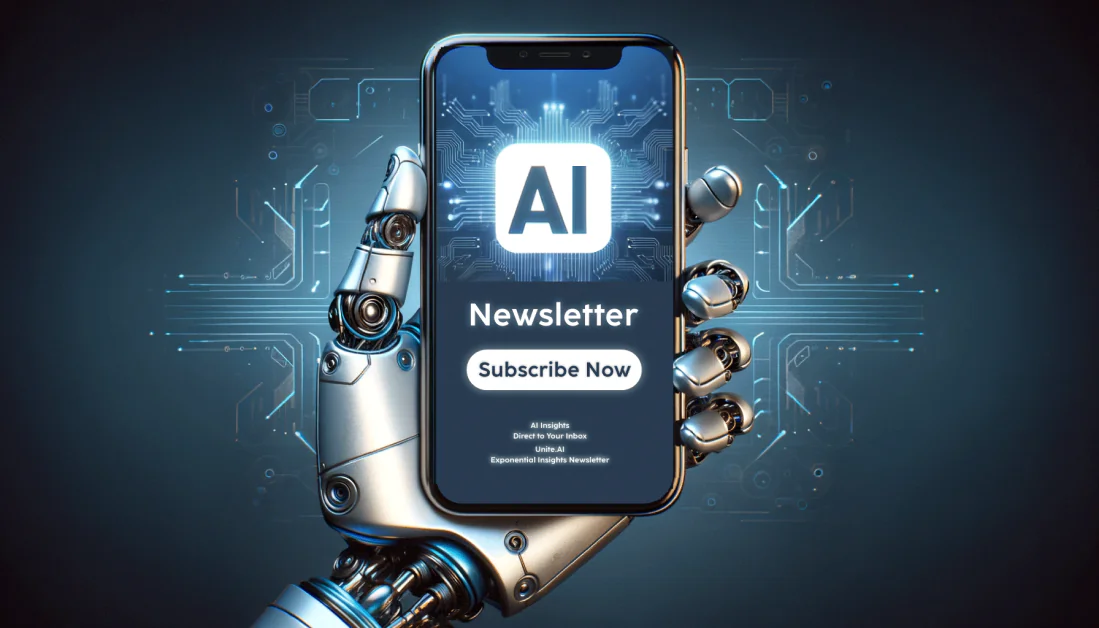