Artificial Intelligence
Researchers Develop “DeepTrust” Tool to Help Increase AI Trustworthiness
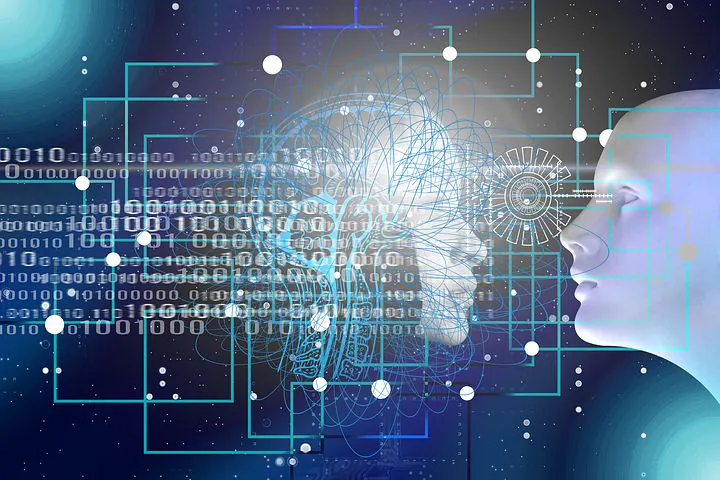
The safety and trustworthiness of artificial intelligence (AI) is one of the biggest aspects of the technology. It is constantly being improved and worked on by top experts within the different fields, and it will be crucial to the full implementation of AI throughout society.
Some of that new work is coming out of the University of Southern California, where USC Viterbi Engineering researchers have developed a new tool capable of generating automatic indicators for whether or not AI algorithms are trustworthy in their data and predictions.
The research was published in Frontiers in Artificial Intelligence, titled “There is Hope After All: Quantifying Opinion and Trustworthiness in Neural Networks”. The authors of the paper include Mingxi Cheng, Shahin Nazarian, and Paul Bogdan of the USC Cyber Physical Systems Group.
Trustworthiness of Neural Networks
One of the biggest tasks in this area is getting neural networks to generate predictions that can be trusted. In many cases, this is what stops the full adoption of technology that relies on AI.
For example, self-driving vehicles are required to act independently and make accurate decisions on auto-pilot. They need to be capable of making these decisions extremely quickly, while deciphering and recognizing objects on the road. This is crucial, especially in scenarios where the technology would have to decipher the difference between a speed bump, some other object, or a living being.
Other scenarios include the self-driving vehicle deciding what to do when another vehicle faces it head-on, and the most complex decision of all is if that self-driving vehicle needs to decide between hitting what it perceives as another vehicle, some object, or a living being.
This all means we are putting an extreme amount of trust into the capability of the self-driving vehicle’s software to make the correct decision in just fractions of a second. It becomes even more difficult when there is conflicting information from different sensors, such as computer vision from cameras and Lidar.
Lead author Minxi Cheng decided to take this project up after thinking, “Even humans can be indecisive in certain decision-making scenarios. In cases involving conflicting information, why can’t machines tell us when they don’t know?”
DeepTrust
The tool that was created by the researchers is called DeepTrust, and it is able to quantify the amount of uncertainty, according to Paul Bogdan, an associate professor in the Ming Hsieh Department of Electrical and Computer Engineering.
The team spent nearly two years developing DeepTrust, primarily using subjective logic to assess the neural networks. In one example of the tool working, it was able to look at the 2016 presidential election polls and predict that there was a greater margin of error for Hillary Clinton winning.
The DeepTrust tool also makes it easier to test the reliability of AI algorithms normally trained on up to millions of data points. The other way to do this is by independently checking each one of the data points to test accuracy, which is an extremely time consuming task.
According to the researchers, the architecture of these neural network systems is more accurate, and accuracy and trust can be maximized simultaneously.
“To our knowledge, there is no trust quantification model or tool for deep learning, artificial intelligence and machine learning. This is the first approach and opens new research directions,” Bogdan says.
Bogdan also believes that DeepTrust could help push AI forward to the point where it is “aware and adaptive.”
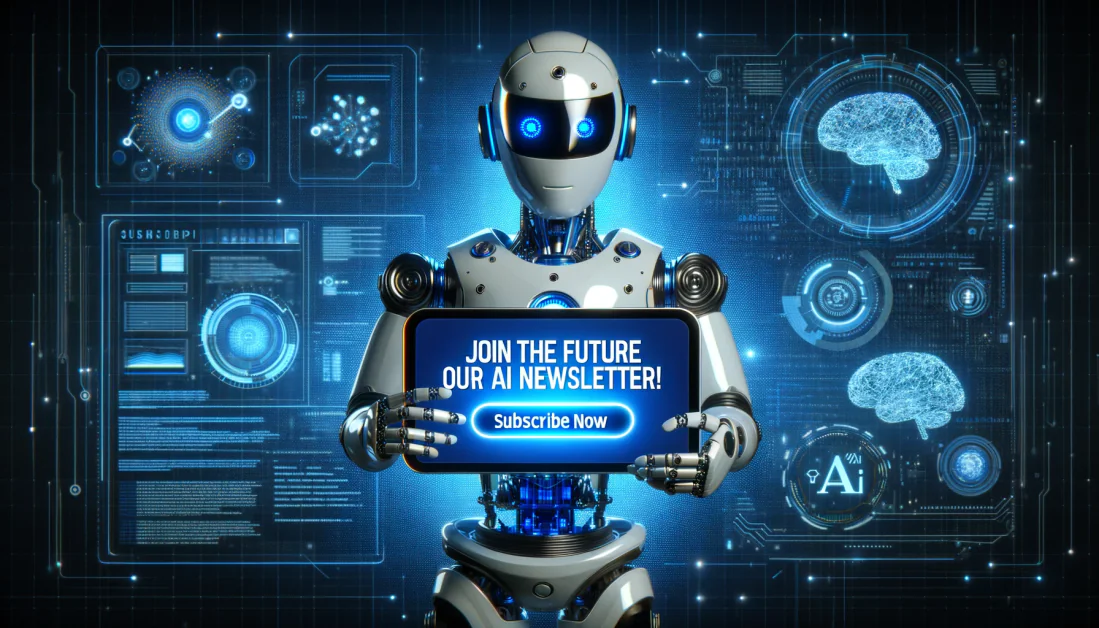
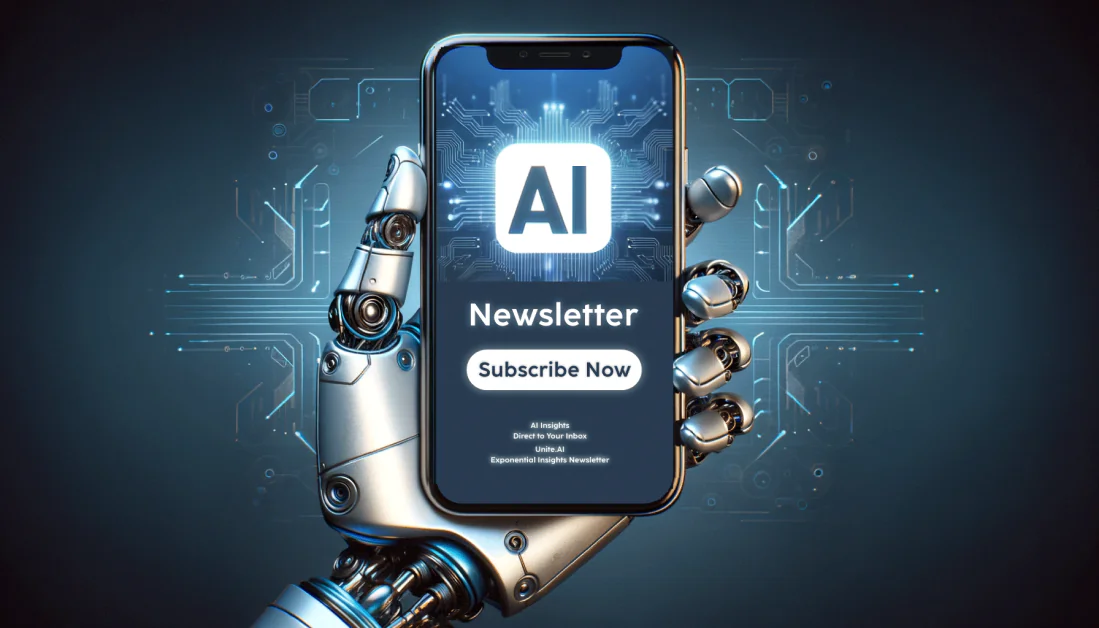