Interviews
Josh Miller, CEO of Gradient Health – Interview Series
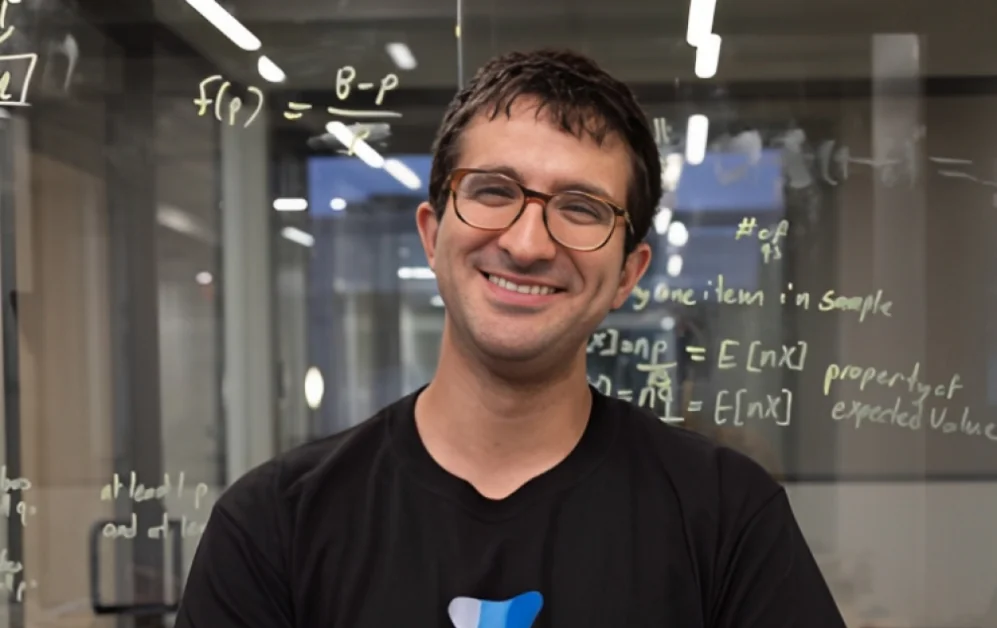
Josh Miller is the CEO of Gradient Health, a company founded on the idea that automated diagnostics must exist for healthcare to be equitable and available to everyone. Gradient Health aims to accelerate automated A.I. diagnostics with data that’s organized, labeled, and available.
Could you share the genesis story behind Gradient Health?
My cofounder Ouwen and I had just exited our first start-up, FarmShots, which utilized computer vision to help reduce the amount of pesticides used in agriculture, and we were looking for our next challenge.
We’ve always been motivated by the desire to find a tough problem to solve with technology that a) has the opportunity to do a lot of good in the world, and b) leads to a solid business. Ouwen was working on his medical degree, and with our experience in computer vision, medical imaging was a natural fit for us. Because of the devastating impact of breast cancer, we chose mammography as a potential first application. So we said, “Ok where do we start? We need data. We need a thousand mammograms. Where do you get that scale of data?” and the answer was “Nowhere”. We realized immediately, it’s really hard to find data. After months, this frustration grew into a philosophical problem for us, we thought “anyone that’s trying to do good in this space shouldn’t have to fight and struggle to get the data they need to build life-saving algorithms”. And so we said “hey, maybe that’s actually our problem to solve”.
What are the current risks in the marketplace with unrepresentative data?
From countless studies and real-world examples, we know that if we build an algorithm, using only data from the west coast, and you bring it to the southeast, it just won’t work. Time and again we hear stories of AI that works great in the northeastern hospital it was created in, and then when they deploy it elsewhere the accuracy drops to less than 50%.
I believe the fundamental purpose of AI, on an ethical level, is that it should decrease health discrepancies. The aim is to make quality care affordable and accessible to everyone. But the problem is when you have it built on poor data, you actually increase the discrepancies. We’re failing at the mission of healthcare AI if we let it only work for white guys from the coasts. People from underrepresented backgrounds will actually suffer more discrimination as a result, not less.
Could you discuss how Gradient Health sources data?
Sure, we partner up with all types of health systems around the world whose data is otherwise stored away, costing them money, and not benefiting anyone. We thoroughly de-identify their data at source and then we carefully organize it for researchers.
How does Gradient Health ensure that the data is unbiased and as diverse as possible?
There are lots of ways. For example, when we’re collecting data, we make sure we include lots of community clinics, where you often have much more representative data, as well as the bigger hospitals. We also source our data from a large number of clinical sites. We try to get as many sites as possible from as wide a range of populations as possible. So not just having a high number of sites, but having them geographically and socio-economically diverse. Because if all your sites are all from downtown hospitals it’s still not representative data, is it?
To validate all this, we run stats across all of these datasets, and we customize it for the client, to make sure they’re getting data that is diverse in terms of technology and demographics.
Why is this level of data control so important to design robust AI algorithms?
There are many variables that an AI might encounter in the real world, and our aim is to ensure the algorithm is as robust as it possibly can be. To simplify things, we think of five key variables in our data. The first variable we think about is “equipment manufacturer”. It’s obvious, but if you build an algorithm only using data from GE scanners, it’s not going to perform as well on a Hitachi, say.
Along similar lines is the “equipment model” variable. This one is actually quite interesting from a health inequality perspective. We know that the large, well-funded research hospitals tend to have the latest and greatest versions of scanners. And, if they only train their AI on their own 2022 models, it’s not going to work as well on an older 2010 model. These older systems are exactly the ones found in less affluent and rural areas. So, by only using data from newer models they’re inadvertently introducing further bias against people from these communities.
The other key variables are gender, ethnicity, and age, and we go to great lengths to make sure our data is proportionately balanced across all of them.
What are some of the regulatory hurdles MedTech companies face?
We’re starting to see the FDA really investigate bias in datasets. We’ve had researchers come to us and say “the FDA has rejected our algorithm because it was missing a 15% African American population” (the approximate percentage of African Americans that are part of the US population). We’ve also heard of a developer being told they need to include 1% Pacific Hawaiian Islanders in their training data.
So, the FDA is starting to realize that these algorithms, which were just trained at a single hospital, don’t work in the real world. The fact is, that if you want CE marking & FDA clearance you’ve got to come with a dataset that represents the population. It’s, rightly, no longer acceptable to train an AI on a small or non-representative group.
The risk for MedTechs is that they invest millions of dollars getting their technology to a place where they think they’re ready for regulatory clearance, and then if they can’t get it through, they’ll never get reimbursement or revenue. Ultimately, the path to commercialization and the path to having the sort of beneficial impact on healthcare that they want to have requires them to care about data bias.
What are some of the options for overcoming these hurdles from a data perspective?
Over recent years, data management methods have evolved, and AI developers now have more options available to them than ever before. From data intermediaries and partners to federated learning and synthetic data, there are new approaches to these hurdles. Whatever method they choose, we always encourage developers to consider if their data is truly representative of the population that will use the product. This is by far the most difficult aspect of sourcing data.
A solution that Gradient Health offers is Gradient Label, what is this solution and how does it enable labeling data at scale?
Medical imaging AI doesn’t just require data, but also expert annotations. And we help companies get those expert annotations, including from radiologists.
What’s your vision for the future of AI and data in healthcare?
There are already thousands of AI tools out there that look at everything from the tips of your fingers to the tips of your toes, and I think this is going to continue. I think there are going to be at least 10 algorithms for every condition in a medical textbook. Each one is going to have multiple, probably competitive, tools to help clinicians provide the best care.
I don’t think we’re likely to end up seeing a Star Trek style Tricorder that scans someone and addresses every possible issue from head to toe. Instead, we’ll have specialist applications for each subset.
Is there anything else that you would like to share about Gradient Health?
I’m excited about the future. I think we’re moving towards a place where healthcare is inexpensive, equal, and available to all, and I’m keen that Gradient gets the chance to play a fundamental role in making this happen. The whole team here genuinely believes in this mission, and there’s a united passion across them that you don’t get at every company. And I love it!
Thank you for the great interview, readers who wish to learn more should visit Gradient Health.
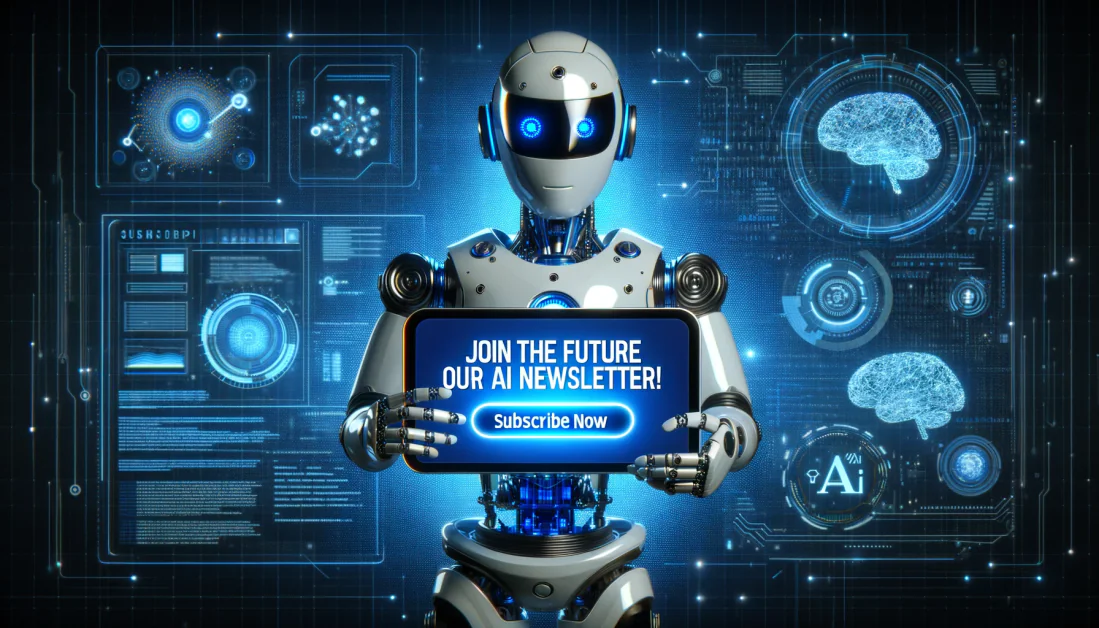
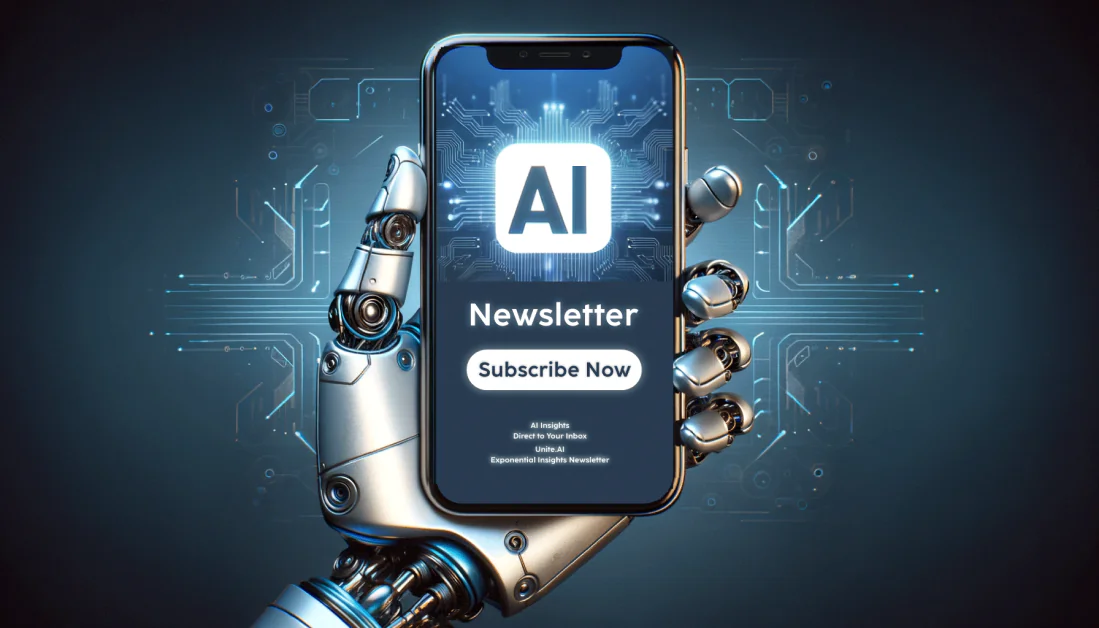