Interviews
Vinay Kumar Sankarapu, Co-Founder & CEO of Arya.ai – Interview Series
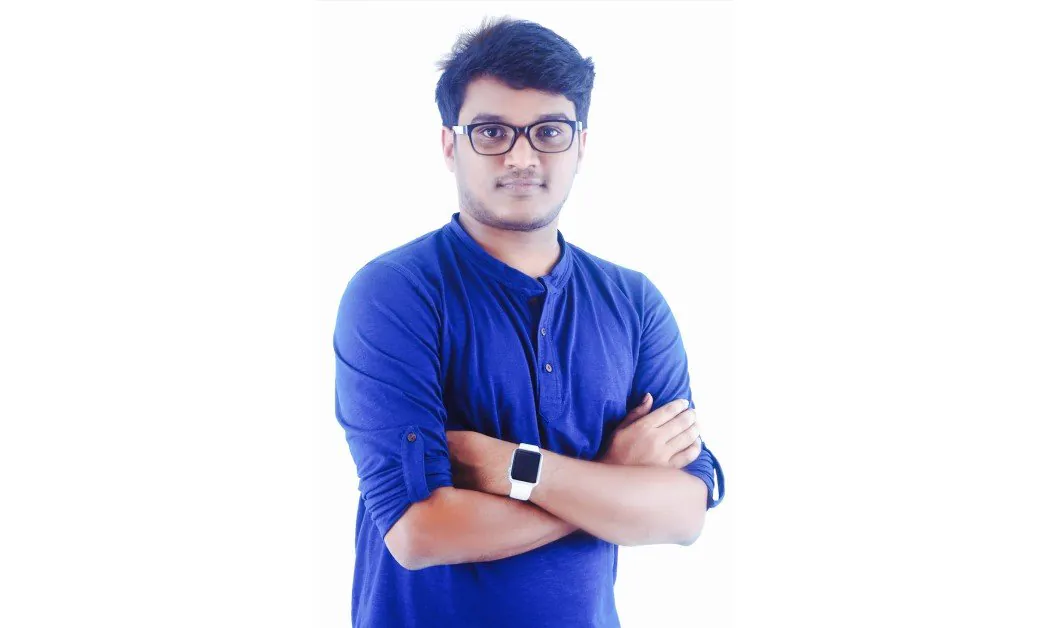
Vinay Kumar Sankarapu, is the Co-Founder & CEO of Arya.ai, a platform that offers the ‘AI' cloud for Banks, Insurers and Financial Services (BFSI) institutions to find the right AI APIs, Expert AI Solutions and comprehensive AI Governance tools required to deploy trustable and self-learning AI engines.
Your background is in math, physics, chemistry and mechanical engineering, could you discuss your journey to transitioning to computer science and AI?
At IIT Bombay, we have ‘Dual Degree Program’ that provides a 5-year course to cover both Bachelors of Technology and Masters of Technology. I did Mechanical Engineering with a specialization in ‘Computer Aided Design and Manufacturing, where Computer Science is part of our curriculum. For our Post-grad research, I chose to work on Deep Learning. While I started using DL to build a failure prediction framework for continuous manufacturing, I finished my research on using CNNs for RUL prediction. This was around 2013/14.
You launched Arya.ai while still in college, could you share the genesis story behind this startup?
As part of academic research, we had to spend 3-4 months on a literature review to create a detailed study on the topic of interest, the scope of work done so far and what could be a possible area of focus for our research. During 2012/13, the tools we used were quite basic. Search engines like Google scholar and Scopus were just doing a keyword search. It was really tough to comprehend the volume of knowledge that was available. I thought this problem would only going to get worse. In 2013, I think at least 30+ papers were published every minute. Today, that’s at least 10x-20x than that.
We wanted to build an ‘AI’ assistant like a ‘professor’ for researchers to help them suggest a topic of research, find a suitable paper that is most up to date and anything around STEM research. With our experience in deep learning, we thought we could solve this problem. In 2013, we started Arya.ai with a team of 3, and then it expanded to 7 in 2014 while I was still in college.
Our first version of the product was built by scraping more than 30 million papers and abstracts. We used state-of-art techniques in deep learning at that time to build an AI STEM research assistant and a contextual search engine for STEM. But when we showcased the AI assistant to a few professors and peers, we realized that we were too early. Conversational flows were limited, and users were expecting a free flow and continuous conversions. Expectations were very unrealistic at that time (2014/15) even though it was answering complex questions.
Post that, we pivoted to use our research and focus on ML tools for researchers and enterprises as a workbench to democratize deep learning. But again, very few data scientists were using DL in 2016. So, we started verticalizing it for one vertical and focused on building specialized product layers for one vertical, ie., Financial Services Institutions (FSIs). We knew this would work because while large players aim to win the horizontal play, verticalization can create a big USP for startups. This time we were right!
We are building the AI cloud for Banks, Insurers and Financial Services with the most specialized vertical layers to deliver scalable and responsible AI solutions.
How big of an issue is the AI black box problem in finance?
Extremely important! Only 30% of financial institutions are using ‘AI’ to its full potential. While one of the reasons is accessibility, another is the lack of ‘AI’ trust and auditability. Regulations are now clear in a few geographies on the legalities of using AI for Low, medium and high-sensitive use cases. It is required by law in EU to use transparent models for ‘high-risk’ use cases. Many use cases in financial institutions are high-risk use cases. So, they are required to use white-box models.
Hype cycles are also settling down because of early experience with AI solutions. There are a growing number of examples in recent times on the effects of using black box ‘AI’, failures of ‘AI’ because of not monitoring them and challenges with legal and risk managers because of limited auditability.
Could you discuss the difference between ML monitoring and ML observability?
The job of a monitoring tool is simply to monitor and alert. And the job of an observability tool is not only to monitor & report but, most importantly, to provide enough evidence to find the reasons for failure or predict these failures over time.
In AI/ML, these tools play a critical role. While these tools can deliver required roles or monitoring, the scope of ML observability
Why are industry specific platforms needed for ML observability versus general purpose platforms?
General-purpose platforms are designed for everyone and any use case, regardless of the industry– any user can come on board and start using the platform. The customers of these platforms are usually developers, data scientists, etc. The platforms, however, create several challenges for the stakeholders because of their complex nature and ‘one size fits all’ approach.
Unfortunately, most businesses today require data science experts to use general-purpose platforms and need additional solutions/product layers to make these models ‘usable’ by the end users in any vertical. This includes explainability, auditing, segments/scenarios, human-in-the-loop processes, feedback labelling, auditing, tool-specific pipelines etc.
This is where industry-specific AI platforms come in as an advantage. An industry-specific AI platform owns the entire workflow to solve a targeted customer’s need or use cases and is developed to provide a complete product from end to end, from understanding the business needs to monitoring product performance. There are many industry-specific hurdles, such as regulatory and compliance frameworks, data privacy requirements, audit and control requirements, etc. Industry-specific AI platforms and offerings accelerate AI adoption and shorten the path to production by reducing the development time and associated risks in AI rollout. Moreover, this will also help bring together AI expertise in the industry as a product layer that helps to improve acceptance of ‘AI’, push compliance efforts and figure out common approaches to ethics, trust, and reputational concerns.
Could you share some details on the ML Observability platform that is offered by Arya.ai?
We have been working in financial services institutions for more than 6+ years. Since 2016. This gave us early exposure to unique challenges in deploying complex AI in FSIs. One of the important challenges was ‘AI acceptance. Unlike in other verticals, there are many regulations on using any software (also applicable for ‘AI’ solutions), data privacy, ethics and most importantly, the financial impact on the business. To address these challenges at scale, we had to continuously invent and add new layers of explainability, audit, usage risks and accountability on top of our solutions – claims processing, underwriting, fraud monitoring etc. Over time, we made an acceptable and scalable ML Observability framework for various stakeholders in the financial services industry.
We are now releasing a DIY version of the framework as AryaXAI (xai.arya.ai). Any ML or business team can use AryaXAI to create a highly comprehensive AI Governance for mission-critical use cases. The platform brings transparency & auditability to your AI Solutions that are acceptable to every stakeholder. AryaXAI makes AI safer and acceptable for mission-critical uses cases by providing a reliable & accurate explainability, offering evidence that can support regulatory diligence, managing AI uncertainty by providing advanced policy controls and ensuring consistency in production by monitoring data or model drift and alerting users with root cause analysis.
AryaXAI also acts as a common workflow and provides insights acceptable by all stakeholders – Data Science, IT, Risk, Operations and compliance teams, making the rollout and maintenance of AI/ML models seamless and clutter-free.
Another solution that is offered is a platform that enhances the applicability of the ML model with contextual policy implementation. Could you describe what this is specifically?
It becomes difficult to monitor and control ML models in production, owing to the sheer volumes of features and predictions. Moreover, the uncertainty of model behavior makes it challenging to manage and standardize governance, risk, and compliance. Such failures of the models can result in heavy reputational and financial losses.
AryaXAI offers ‘Policy/Risk controls’, a critical component which preserves business and ethical interests by enforcing policies on AI. Users can easily add/edit/modify policies to administer policy controls. This enables cross-functional teams to define policy guardrails to ensure continuous risk assessment, protecting the business from AI uncertainty.
What are some examples of use cases for these products?
AryaXAI can be implemented for various mission-critical processes across industries. The most common examples are:
BFSI: In an environment of regulatory strictness, AryaXAI makes it easy for the BFSI industry to align on requirements and collect the evidence needed to manage risk and ensure compliance.
- Credit Underwriting for secure/unsecured loans
- Identifying fraud/suspicious transactions
- Audit
- Customer lifecycle management
- Credit decisioning
Autonomous cars: Autonomous vehicles need to adhere to regulatory strictness, operational safety and explainability in real-time decisions. AryaXAI enables an understanding how the AI system interacts with the vehicle
- Decision Analysis
- Autonomous vehicle operations
- Vehicle health data
- Monitoring AI driving system
Healthcare: AryaXAI provides deeper insights from medical, technological, legal, and patient perspectives. Right from drug discovery to manufacturing, sales and marketing, Arya-xAI fosters multidisciplinary collaboration
- Drug discovery
- Clinical research
- Clinical trial data validation
- Higher quality care
What’s your vision for the future of machine learning in finance?
Over the past decade, there has been an enormous education and marketing around ‘AI’. We have seen multiple hype cycles during this time. We’d probably be at 4th or 6th hype cycle now. The first one is when Deep Learning won ImageNet in 2011/12 followed by work around image/text classification, speech recognition, autonomous cars, generative AI and currently with large language models. The gap between the peak hype and mass usage is reducing with every hype cycle because of the iterations around the product, demand and funding.
These three things have happened now:
- I think we’ve cracked the framework of scale for AI solutions, at least by a few experts. For example, Open AI is currently a non-revenue generating organisation, but they are projecting to do $1 Billion in revenue within 2 years. While not every AI company may not achieve a similar scale but the template of scalability is clearer.
- The definition of Ideal AI solutions is almost clear by all verticals: Unlike earlier, where the product was built through iterative experiments for every use case and every organization, stakeholders are increasingly educated to understand what they need from AI solutions.
- Regulations are now catching up: The need for clear regulations around Data privacy and AI usage is now gaining great traction. Governing bodies and regulating bodies are able to publish or are in the process of publishing frameworks required for the safe, ethical and responsible use of AI.
What’s next?
The explosion of ‘Model-as-a-service(MaaS)’:
We are going to see an increasing demand for ‘Model-as-a-service’ propositions not just horizontally but vertically as well. While ‘OpenAI’ represents a good example of ‘Horitzonal MaaS’, Arya.ai is an example of vertical ‘MaaS’. With the experience of deployments and datasets, Arya.ai has been collecting critical vertical data sets that are leveraged to train models and provide them as plug-and-use or pre-trained models.
Verticalization is the new horizontal: We have seen this trend in ‘Cloud adoption’. While horizontal cloud players focus on ‘platforms-for-everyone’, vertical players focus on the requirements of the end-user and provide them as a specialized product layer. This is true even for MaaS offerings.
XAI and AI governance will become a norm in enterprises: Depending on the sensitivity of regulations, each vertical will achieve an acceptable XAI and governance framework that’d get implemented as part of the design, unlike today, where it is treated as an add-on.
Generative AI on tabular data may see its hype cycles in enterprises: Creating synthetic data sets is supposedly one of the easy-to-implement solutions to solve data-related challenges in enterprises. Data science teams would highly prefer this as the problem is in their control, unlike relying on the business as they may take time, be expensive and not guaranteed to follow all the steps while collecting data. Synthetic data solves bias issues, data imbalance, data privacy, and insufficient data. Of course, the efficacy of this approach is still yet to be proven. Still, with more maturity in new techniques like transformers, we may see more experimentation on traditional data sets like tabular and multi-dimensional data. Upon success, this approach can have a tremendous impact on enterprises and MaaS offerings.
Is there anything else that you would like to share about Arya.ai?
The focus of Arya.ai is solving the ‘AI’ for Banks, Insurers and Financial Services. Our approach is the verticalization of the technology to the last layer and making it usable and acceptable by every organization and stakeholder.
AryaXAI (xai.arya.ai) will play an important role in delivering it to the masses within the FSI vertical. Our ongoing research on synthetic data succeeded in a handful of use cases, but we aim to make it a more viable and acceptable option. We will continue to add more layers to our ‘AI’ cloud to serve our mission.
I think we are going to see more startups like Arya.ai, not just in FSI vertical but in every vertical.
Thank you for the great interview, readers who wish to learn more should visit Arya.ai.
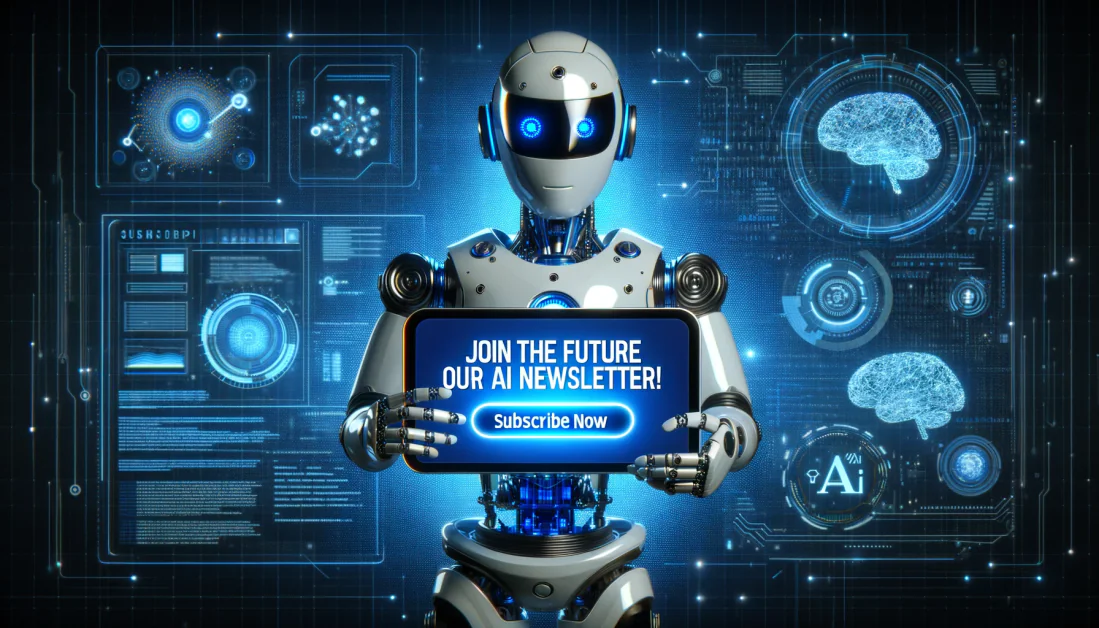
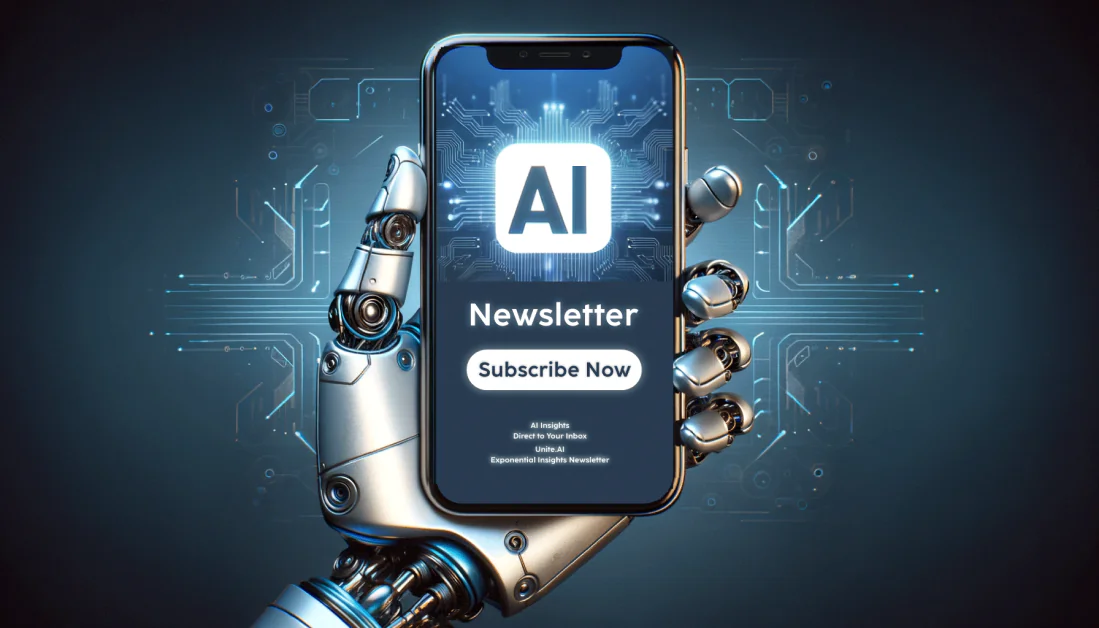