Interviews
Jim McGowan, head of product at ElectrifAi – Interview Series
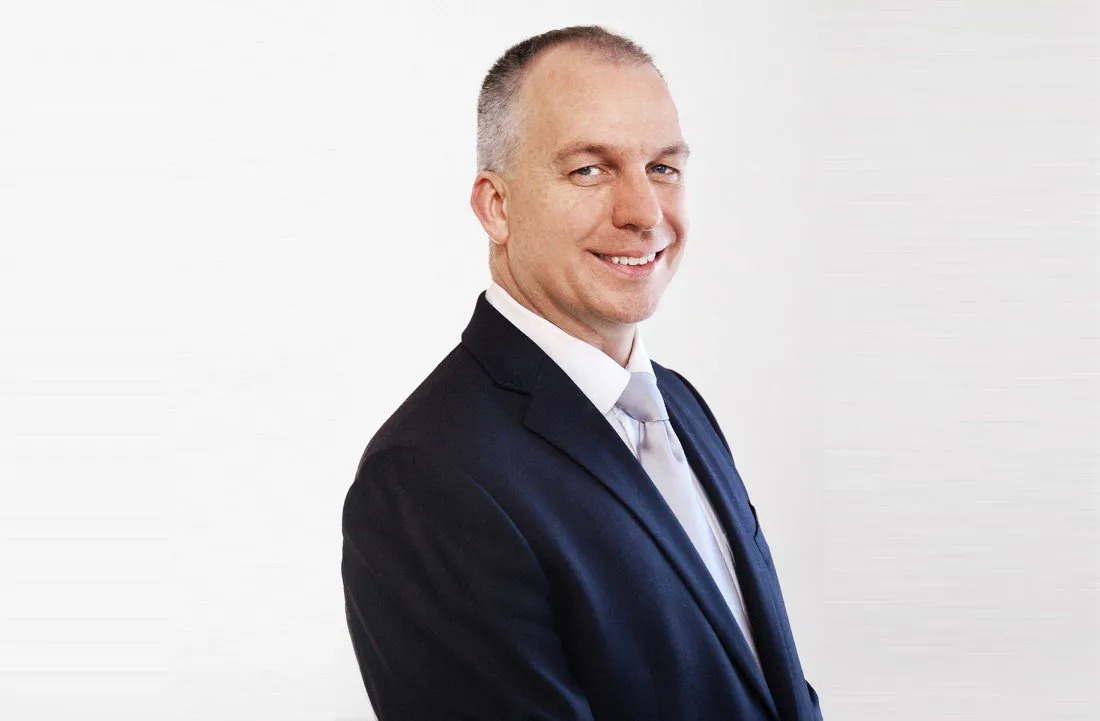
Jim McGowan, is the head of product at ElectrifAi, they specialize in extracting massive amounts of disparate data, transforming chaotic structured and unstructured data into actionable business insights.
What is it that attracted you to the world of machine learning and AI?
I first encountered Machine Learning while earning a doctorate for work in cognitive science. AI systems largely consisted of distilling an expert’s experience down to a flow chart. This seemed intuitively to work, but the systems quickly grew too complex and weren’t living up to their promise. Small problems could be solved, but practical solutions to real-world problems were out of reach. You can say that building practical systems was itself impractical. Then Machine Learning came along. That changed everything. Machine Learning unlocked the promise of AI. ElectrifAi fulfills that promise by building solutions to help our clients run their businesses better.
ElectrifAi uses something called Practical AI to guide companies to do more with the data that they already have. Can you elaborate on how ElectrifAi defines Practical AI?
We leverage client’s data to provide clear, actionable insights, for real business needs. We help them make better decisions, faster. Practical AI is solving a real-world business problem with a solution that works well, is based on a clear understanding of the data, has a definitive outcome, fits into existing processes and tools, ships on time, and delivers tremendous business value. We don’t for companies to replace their data systems. We don’t require a specific business model. We don’t take a year to maybe deliver something that’s a compromise on what a client set out to do. We provide a flexible, high-quality solution that is simple to use and does what it supposed to do very, very well. That’s Practical AI.
We make sure with every solution that we achieve the following:
- Best-in-class time to value
- Best-in-class data cleansing
- Best-in-class insights
- Best-in-class ROIs
Could you give some details on how ElectrifAi enables companies to use this Practical AI?
We pull data from all systems—whether they are custom developed databases, highly customized solutions from major vendors, or even a data dump from some legacy application. We clean and understand that data, and find clear, meaningful signals in all that chaos. We then use machine learning to extract valuable insights from those signals, and finally, we indicate how to act on those insights. SpendAi is a great example. We use machine learning to clean the data, and the more machine learning to categorize 98-99% of data from all a client’s procurement systems. We even let the client control that categorization and a granular level, in just seconds, through a drag-and-drop interface. That’s unique, and incredibly powerful. Then we apply another group of machine learning algorithms to give a clear, simple view of where spend is going. We use machine learning to parse contracts and extract key clauses. We then apply still more machine learning to make specific recommendations. For instance, a client may be due a discount from a clause buried in a contract term. Or they may be over-relying on a vendor in a category, who is at financial risk themselves. A client may be under-leveraging their position with a vendor because the vendor operates under multiple names and across multiple divisions of the company. We surface and clean all that, so the client can reduce their spend, increase their working capital, and reduce their risk.
Could you discuss PulmoAi CT, and how it may increase efficiency for radiologists and improves radiological outcomes?
PulmoAi CT is an advanced image analytic product designed specifically for pulmonary CTscans. Combining Practical AI, Machine Learning (ML), and image processing technology, PulmoAi CT automatically segments pulmonary scans pixel by pixel, without the blurring or distortion experienced with similar technologies. The result: Crisply rendered 3D imagery— enabling the immediate identification of indications for tumors, nodules, COVID-19, and other anomalies. With PulmoAi CT, radiologists can easily zoom in on pulmonary details, viewing them side-byside with both clinical analyses and original images. PulmoAi CT quantifies each lung feature with precise metrics, including feature size, and morphological and volumetric extent. This enables the careful monitoring of anomaly progression, even in the presence of multiple morbidities.
PulmoAi CT is a very different technology than any product in the market or even research laboratory. The results are game changing. There’s nothing else like it. It’s not a brute-force approach that requires tens of thousands of samples to work. PulmoAi CT produces results while other Ai solutions are still looking for training data. It’s powerful and it will change what radiologists can do.
Another ElectrifAi product is the PulmoAi X-ray which directly addresses the use of X-rays in crisis zones. Could you discuss this technology?
PulmoAi X-ray directly addresses the use of X-rays in crisis zones today. Adapting to the specific challenges of the pandemic, PulmoAi X-ray goes a step further than distinguishing healthy lungs from COVID-19-infected lungs. The cloud-based solution identifies the crucial differences between coronavirus-positive patients sent home who recover safely, and those sent home who return in need of intubation. Pre-trained on pulmonary scans from hospitals in crisis zones, PulmoAi X-ray leverages deep learning neural network technologies to identify critical abnormalities associated with COVID-19. PulmoAi X-ray is unique because it is narrowly tuned to answer the problem that hospitals in crisis zones are trying to answer: will self-quarantine work, or does the patient need hospitalization?
Another product is ContractAi which uses practical AI, Machine Learning, and Natural Language Processing (NLP) to automatically read, analyze, and compare contracts across the enterprise. Could you discuss this product and the best use cases for it?
ContractAi is designed for users who interact with contracts in a day-to-day operational role. For example, ContractAi helps people in a procurement group who are analyzing spend against vendor agreements. Recently, with economic shock due to COVID-19, the software is helping companies understand any leverage they may have to exit supplier contracts. When this capability is connected to our SpendAi product, one can immediately understand the financial impact of this leverage. One of the largest advantages of the technology is that it works with contract data in any format—there is no manual entry and no specific format required. Another advantage is that the technology is specifically designed for users who use contracts in an operational role. Many of the existing contract processing technologies are designed for attorneys, who have a different set of concerns.
Is there anything else that you would like to share about ElectrifAi?
As a global machine learning company, we have a unique view in to how various markets are developing and using Machine Learning. One advantage of this view is our ability to understand how machine learning (ML) capabilities can be translated from one geography and/or vertical market to another to help solve substantial problems.
For example, we have spent years around the world helping businesses engage their customers using data science. We have now leveraged that expertise to help the US healthcare industry with patient engagement, helping restart healthcare and getting patients back in to the hospitals for critical elective surgeries.
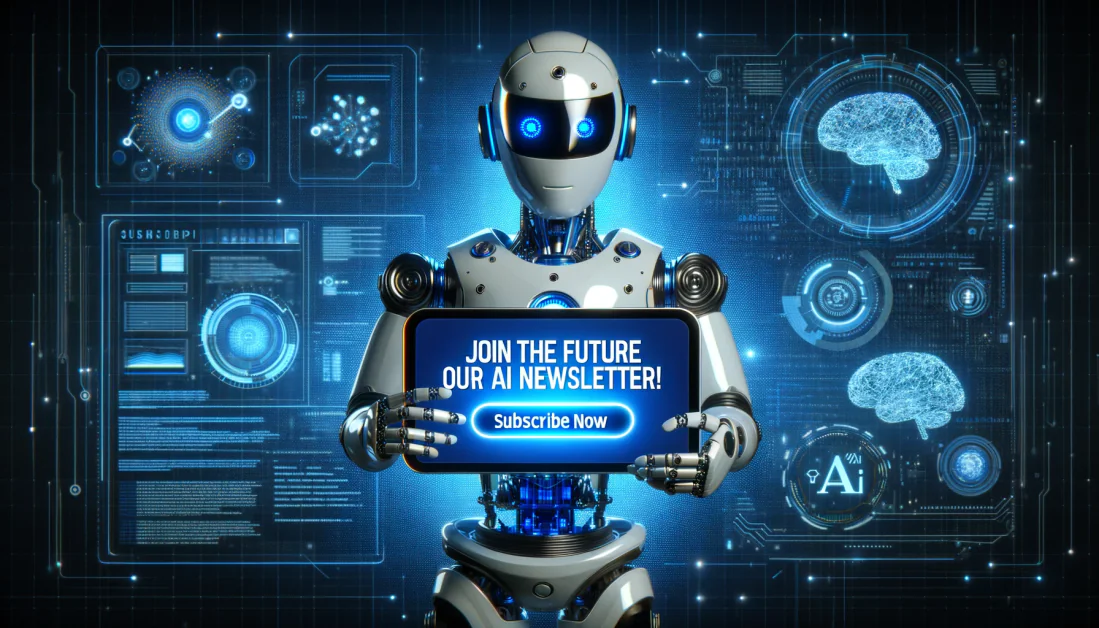
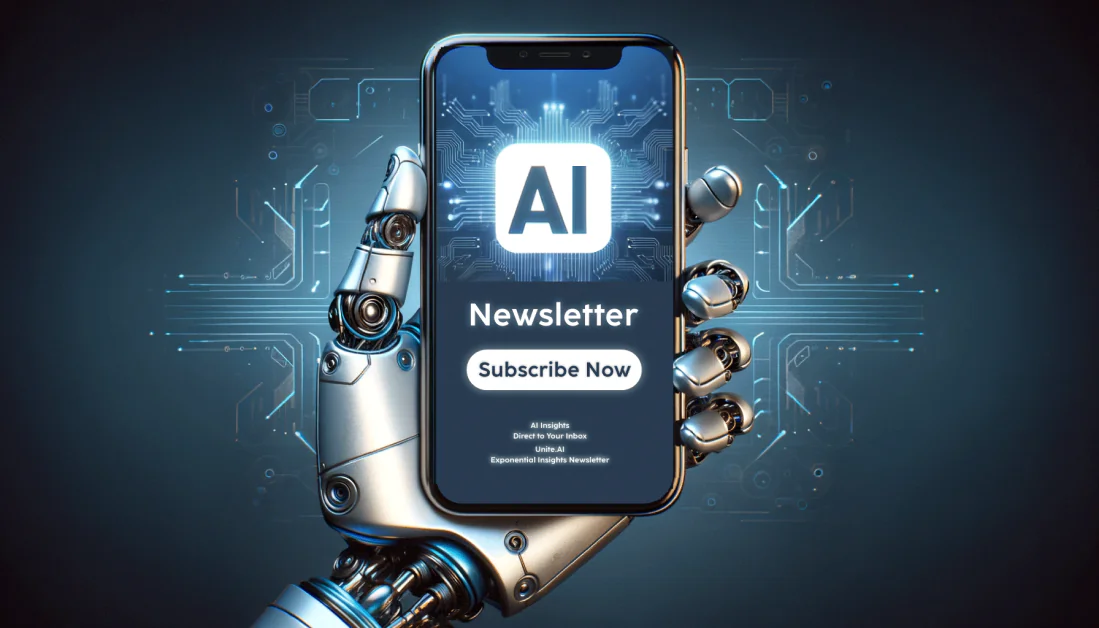