Interviews
Ian Wong, Co-founder & CTO of Opendoor – Interview Series
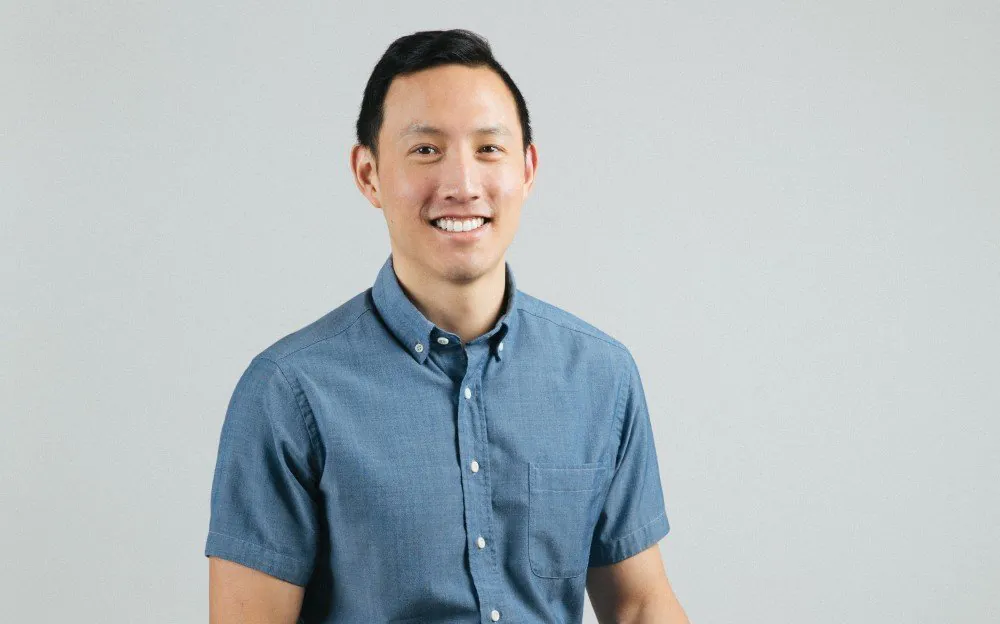
Can you summarize the concept behind Opendoor, and how does it differ from competitors such as Zillow?
Opendoor gives people a simple and convenient way to buy, sell and trade-in homes. We’re turning a fragmented, inflexible real estate model into an end-to-end, digital and on-demand experience. As the pioneer of “ibuyering”, Opendoor has served over 70,000 customers to date and expanded to 21 U.S. markets.
Opendoor is able to provide near-instant fair market values for homes using a proprietary valuation model that leverages first and third-party data, along with the use of machine learning, AI and human review. With just a few taps in the Opendoor app, sellers can receive an offer from Opendoor within 24 hours. Selling to Opendoor provides more choice and certainty as homeowners can choose their move out date and avoid the hassle and stress of home showings and repairs.
In addition, we’ve begun solving for other pain points in the home transaction with the launch of anew product that reimagines the home buying process, the launch of a home loans business and the acquisition of a title and escrow company. Our goal is to make moving seamless, on-demand and stress free.
What was it that attracted you to Opendoor?
We have the chance to reimagine the real-estate transaction, thereby redefining people's relationships with their largest asset. As opposed to a liability, what if homeowners can tap into liquidity afforded by their homes in the same way you and I can withdraw from our checking account? What if buyers and sellers can skip months of stress and uncertainty, and become more confident moving forward with the next chapter of their lives? The vision of enabling more geographic mobility and financial freedom is super exciting, and it feels like we're just embarking on that journey.
Opendoor analyzes a large collection of historical market transactions. What type of data points are you assembling?
Accurate real estate data with the level of granularity we need is not easy to come by. We use a combination of large propriety and third-party data sets to understand historical market transactions, including listing-level and home-level details. This means we look at common data points from a listing, like the sale date and price, when the home listed, as well as data points about individual homes, like the number of bedrooms and bathrooms, kitchen attributes or square footage. On top of this, we incorporate features that denote a home’s quality or uniqueness, allowing us to better select comparables and ultimately price the home as accurately as possible. We also take into account similar data from homes currently on the market. Ultimately, these data points help us predict the fair market value of a home and the amount of time it will likely take to resell the home.
Opendoor also analyzes homes that are taken off the market without transacting, how is this data used differently compared to homes that have sold?
We look at similar data for both active homes and homes that are taken off the market without transacting — homes we call “delistings.” Our data set looks at a variety of home-level and list-level details, including square feet and list price, for each transaction. We examine those insights for delistings, but do not get to observe our target variable of days-on-market. Additionally, we look at the market holistically to understand supply and demand. By incorporating non-transacted listings, we’re able to have a more comprehensive picture of the market.
Opendoor uses Ensembling as a factor in house pricing. Can you explain what ensembling is and how Opendoor uses this technology?
When a buyer wants to buy a house or a seller decides to list their home on the market, the way they determine the home’s value will depend on why they are buying or selling. And this can be very different depending on the buyer and seller type. We incorporate this in our model to understand how buyers and sellers view the market, which is where ensembling comes in. Ensembling allows us to use different pricing models together to compute a weighted average of home values. Some models may weigh certain variables differently than others. We’ve found that ensembling generally results in more accurate pricing than any single model.
Opendoor imports big data from various sources which can be a challenge due to how the data was originally labeled or formatted. Opendoor uses Markov Random Field to assist with this issue. Can you explain what this is?
The challenge stems from mutations in the text data, from abbreviations and misspellings to inconsistent ordering of words and numeric spellings. Poor quality data impacts our home valuation models, which is why we implemented a mathematical approach to help standardize text and improve the quality of labels. Markov Random Field enables us to score all labels jointly and more accurately interpret characteristics like subdivisions. The score of each labeling comes from two different components: 1) how well the final labels relate to the original text and 2) how spatially continuous the labels are among neighbors. With the mathematics of Markov chains, we make the data more than just the sum of its parts.
You use a technique called survival analysis to model the average holding time of a home that is listed for sale. What is survival analysis and does it apply in Opendoor’s case?
Fundamentally, we need to understand liquidity on a per home basis, and be able to update our view of the liquidity profile of a home as we get more information. Survival analysis is a statistical method that analyzes the anticipated amount of time it will take until one or more events happen. In our case, we use survival analysis to help us understand and predict how long a house will take to sell. Using this method, we dramatically improve our ability to respond to evolving market conditions, and more accurately predict our unit economics. This helps us determine a risk threshold for each home and make smarter investments, which is vital to our business.
There are often factors that affect the value of a home which are very location dependent, such as road noise. How do you use machine learning to program your valuation model for such an issue?
The Opendoor Valuation Model (OVM) combines machine intelligence with human expertise to provide accurate and competitive offers, taking less apparent factors, like road noise, into account. To do so, we rely on our human operators to identify variables and our machines to predict how much they matter in the pricing algorithm. OpenStreetMap (OSM) is a freely available data set for road geometries and helps us identify homes adjacent to roads. We also look for previous human adjustments on homes to compute the average adjustment value. We’re able to refine these values with scale, and as we collect more human adjustment data for markets, the data set grows and improves the OVM performance. Most importantly, we enrich readily available third-party data with our own proprietary data. As a result, the overall location dependent signals improve dramatically over time.
Is there anything else that you would like to share about Opendoor?
What makes working at Opendoor particularly special for me is that we’re using technology, data science and operational excellence to help solve real world pain points for millions of consumers. This marriage of the online and offline worlds has never been done and comes with lots of new and interesting challenges.
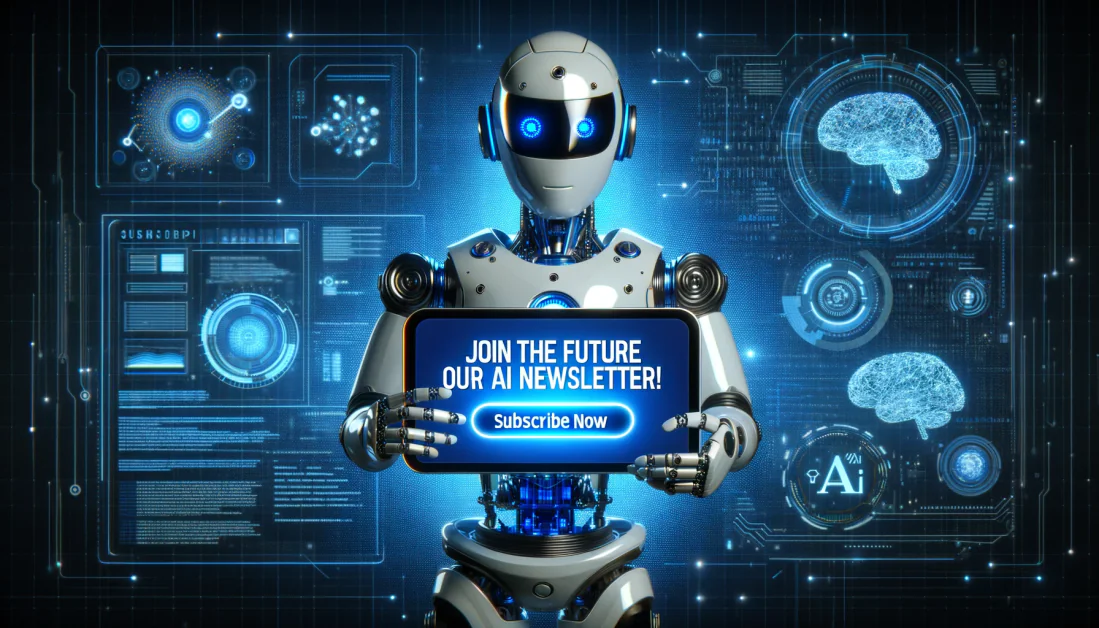
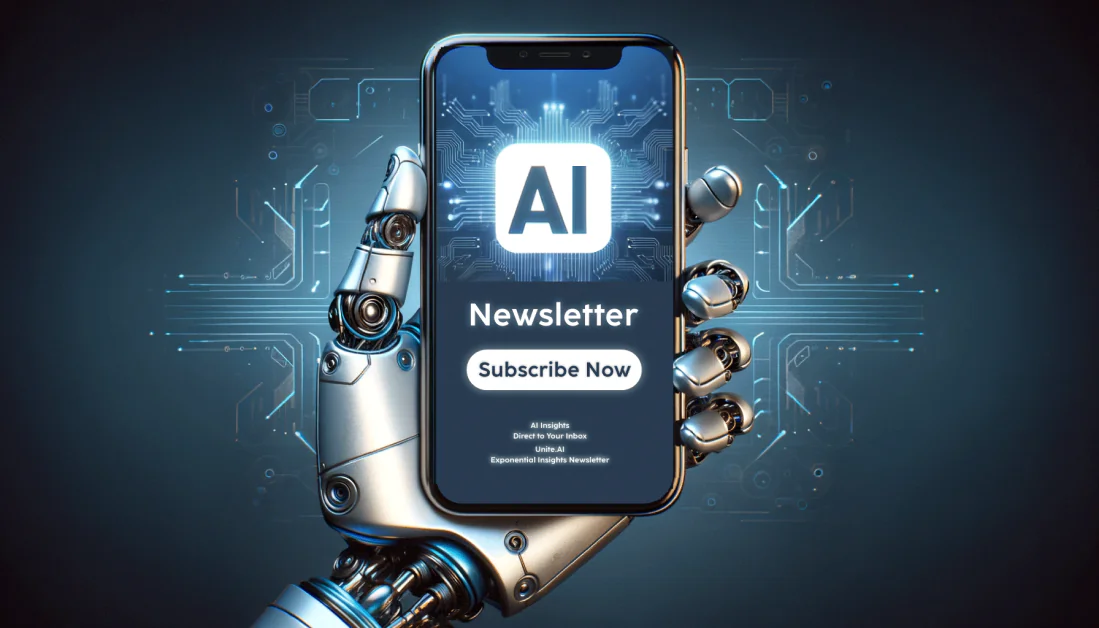