Thought Leaders
How we can use Deep Learning with Small Data? – Thought Leaders
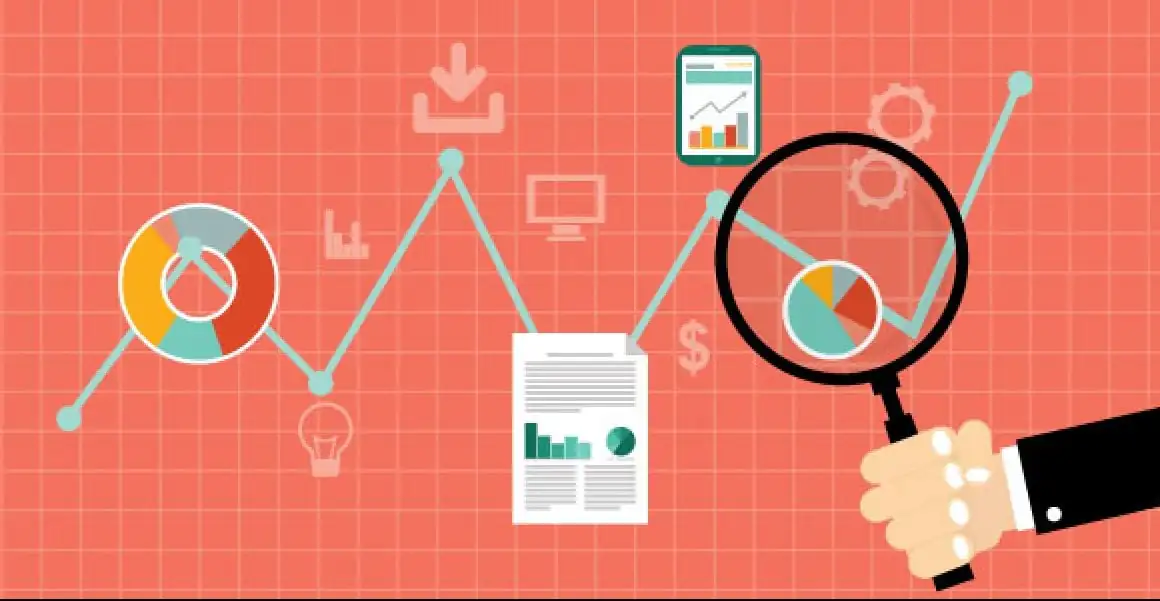
When it comes to keeping up with emerging cybersecurity trends, the process of staying on top of any recent developments can get quite tedious since there’s a lot of news to keep up with. These days, however, the situation has changed dramatically, since the cybersecurity realms seem to be revolving around two words- deep learning.
Although we were initially taken aback by the massive coverage that deep learning was receiving, it quickly became apparent that the buzz generated by deep learning was well-earned. In a fashion similar to the human brain, deep learning enables an AI model to achieve highly accurate results, by performing tasks directly from the text, images, and audio cues.
Up till this point, it was widely believed that deep learning relies on a huge set of data, quite similar to the magnitude of data housed by Silicon Valley giants Google and Facebook to meet the aim of solving the most complicated problems within an organization. Contrary to popular belief, however, enterprises can harness the power of deep learning, even with access to a limited data pool.
In an attempt to aid our readers with the necessary knowledge to equip their organization with deep learning, we’ve compiled an article that dives deep (no pun intended) into some of the ways in which enterprises can utilize the benefits of deep learning in spite of having access to limited, or ‘small’ data.
But before we can get into the meat of the article, we’d like to make a small, but highly essential suggestion- start simple. However, before you start formulating neural networks complex enough to feature in a sci-fi movie, start by experimenting with a few simple and conventional models, (e.g. random forest) to get the hang of the software.
With that out of the way, let’s get straight into some of the ways in which enterprises can amalgamate the deep learning technology while having access to limited data.
#1- Fine-lining the baseline model:
As we’ve already mentioned above, the first step that enterprises need to take after they’ve formulated a simple baseline deep learning model is to fine-tune them for the particular problem at hand.
However, fine-tuning a baseline model sounds much difficult on paper, then it actually is. The fundamental idea behind fine-tuning a large data set to cater to the specific needs of an enterprise is simple- you take a large data, that bears some resemblance to the domain you function in, and then fine-tune the details of the original data set, with your limited data.
As far as obtaining the large data set is concerned, enterprise owners can rely on ImageNet, which subsequently also provides an easy to fix to any problems of image classification as well. The dataset hosted by ImageNet allows organizations access to millions of images, which are divided across multiple classes of images, which can be useful to enterprises hailing from a wide variety of domains, including, but certainly not limited to images of animals, etc.
If the process of fine-tuning a pre-trained model to suit the specific needs of your organization still seems like too much work for you, we’d recommend getting help from the internet, since a simple Google search will provide you with hundreds of tutorials on how to fine-tune a dataset.
#2- Collect more data:
Although the second point on our list might seem redundant to some of our more cynical readers, the fact of the matter remains- when it comes to deep learning, the larger your data set is, the more likely you are to achieve more accurate results.
Although the very essence of this article lies in providing enterprises with a limited data set, we’ve often had the displeasure of encountering too many “higher-ups,” who treat investing in the collection of data equivalent to committing a cardinal sin.
It is all too often that businesses tend to overlook the benefits offered by deep learning, simply because they are reluctant to invest time and effort in the gathering of data. If your enterprise is unsure about the amount of data that needs to be collected, we’d suggest to plot learning curves, as the additional data is integrated into the model, and observe the change in model performance.
Contrary to the popular belief held by most CSO’s and CISO’s, sometimes the best way to solve problems is through the collection of more relevant, data. The role of CSO and CISO is extremely important in this case because there is always a threat of cyber-attacks. It is found that in 2019, the total global spending on cybersecurity takes up to $103.1 billion, and the number continues to rise. To put this into perspective, let’s consider a simple example- imagine that you were trying to classify rare diamonds, but have access to a very limited data set. As the most obvious solution to the problem dictates, instead of having a field day with the baseline model, just collect more data!
#3- Data Augmentation:
Although the first two points we’ve discussed above are both highly efficient in providing an easy solution to most problems surrounding the implementation of deep learning into enterprises with a small data set, they rely heavily on a certain level of luck to get the job done.
If you’re unable to have any success with fine-tuning a pre-existing data set either, we’d recommend trying data augmentation. The way that data augmentation is simple. Through the process of data augmentation, the input data set is altered, or augmented, in such a way that it gives a new output, without actually changing the label value.
To put the idea of data augmentation into perspective for our readers, let’s consider a picture of a dog. When rotated, the viewer of the image will still be able to tell that it’s an image of a dog. This is exactly what good data augmentation hopes to achieve, as compared to a rotated image of a road, which changes the angle of elevation and leaves plenty of space for the deep learning algorithm to come to an incorrect conclusion, and defeats the purpose of implementing deep-learning in the first place.
When it comes to solving problems related to image classification, data augmentation serves as a key player in the field and hosts a variety of data augmentation techniques that help the deep learning model to gain an in-depth understanding of the different classifications of images.
Moreover, when it comes to augmenting data- the possibilities are virtually endless. Enterprises can implement data augmentation in a variety of ways, which include NLP, and experimentation of GANs, which enables the algorithm to generate new data.
#4- Implementing an ensemble effect:
The technology behind deep learning dictates that the network is built upon multiple layers. However, contrary to popular belief maintained by many, rather than viewing each layer as an “ever-increasing” hierarchy of features, the final layer serves the purpose of offering an ensemble mechanism.
The belief that enterprises with access to a limited, or smaller data set should opt to build their networks deep was also shared in a NIPs paper, which mirrored the belief we’ve expressed above. Enterprises with small data can easily manipulate the ensemble effect to their advantage, simply by building their deep learning networks deep, through fine-tuning or some other alternative.
#5- Incorporating autoencoders:
Although the fifth point we’ve taken into consideration for has received only a relative level of success- we’re still on board with the use of autoencoders in order to pre-train a network and initialize the network properly.
One of the biggest reasons apart from cyber-attacks as to why enterprises fail to get over the initial hurdles of integrating deep learning is because of bad initialization, and it’s many pitfalls. Unsupervised pre-training often leads to poor, or incorrect execution of the deep learning technology, which is where autoencoders can shine.
The fundamental notion behind a neural network dictates the creation of a neural network that predicts the nature of the dataset being input. If you are unsure of how to use an autoencoder, there are several tutorials online that give clear cut instructions.
To conclude:
At the end of the article, we’d like to reimburse what we’ve said throughout the article, with one addition- incorporating domain-specific knowledge into the learning process! Not only does the incorporation of valuable insight to speed up the learning process, but it also allows for the deep learning technology to produce better, and more accurate results.
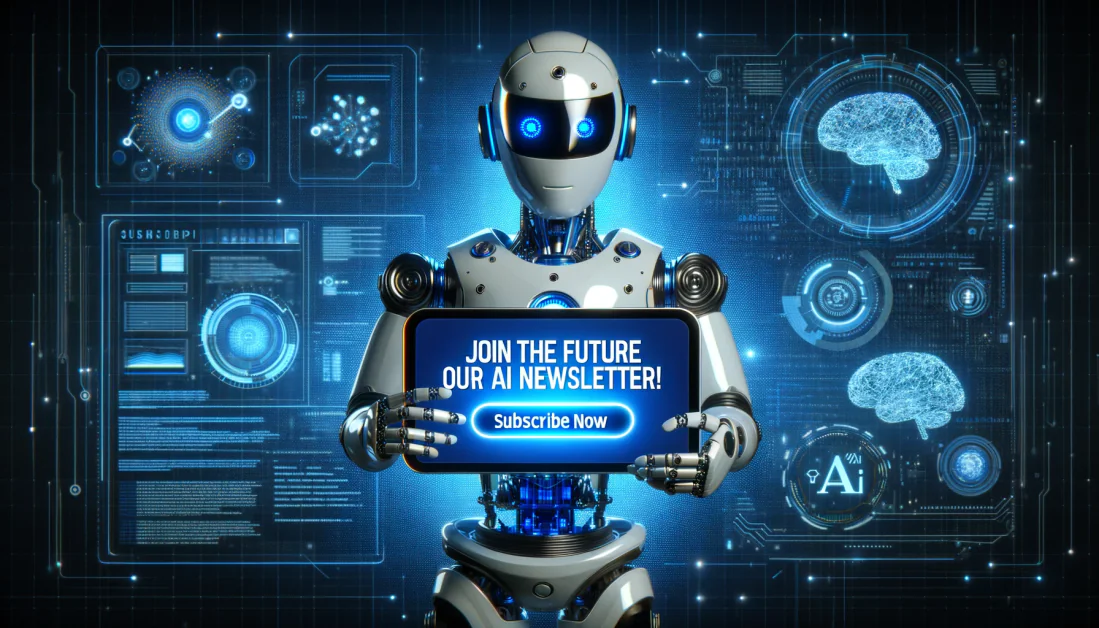
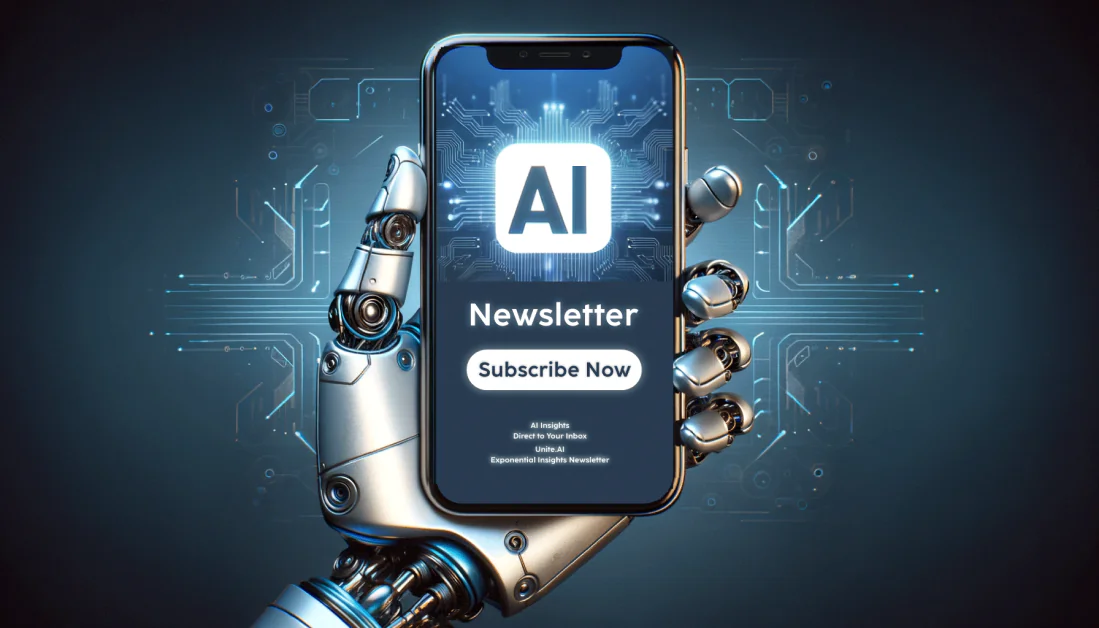