Artificial Intelligence
Experts Overcome Major Obstacle in AI Technology Using Brain Mechanism
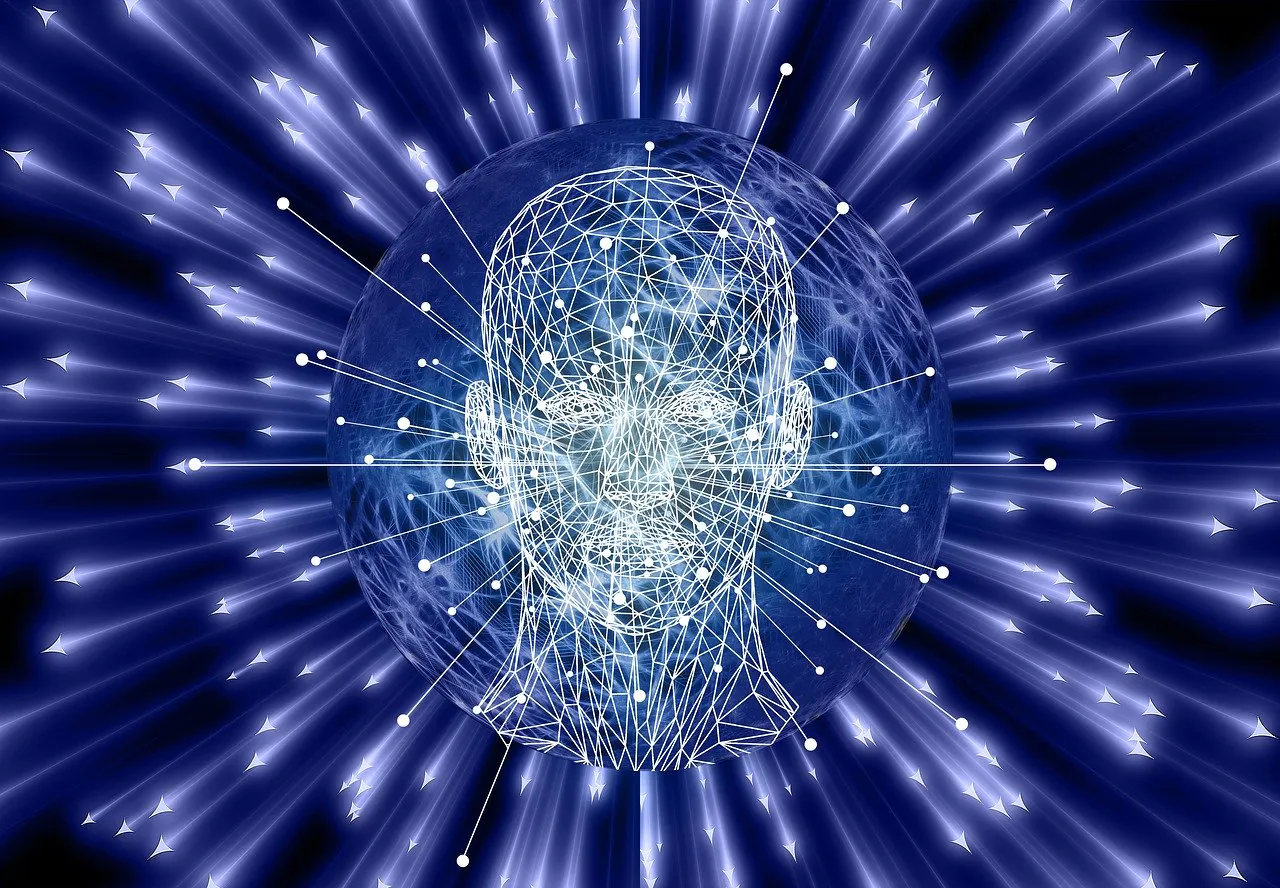
A group of artificial intelligence (AI) experts from various institutions have overcome a “major, long-standing obstacle to increasing AI capabilities.” The team looked toward the human brain, which is the case for many AI developments. Specifically, the team focused on the human brain memory mechanism known as “replay.”
Gido van de Ven is the first author and a postdoctoral researcher. He was joined by principal investigator Andreas Tolias at Baylor, as well as Hava Siegelmann at UMass Amherst.
The research was published in Nature Communications.
The New Method
According to the researchers, they have come up with a new method that efficiently protects deep neural networks from “catastrophic forgetting.” When a neural network takes on new learning, it can forget what was previously learned.
This obstacle is what stops many AI advancements from taking place.
“One solution would be to store previously encountered examples and revisit them when learning something new. Although such ‘replay’ or ‘rehearsal’ solves catastrophic forgetting, constantly retraining on all previously learned tasks is highly inefficient and the amount of data that would have to be stored becomes unmanageable quickly,” the researchers wrote.
The Human Brain
The researchers drew inspiration from the human brain, since it is able to build up information without forgetting, which is not the case for AI neural networks. The current development was built on previous work done by the researchers, including findings regarding a mechanism in the brain that is believed to be responsible for preventing memories from being forgotten. This mechanism is the replay of neural activity patterns.
According to Siegelmann, the major development comes from “recognizing that replay in the brain does not store data,” but “the brain generated representations of memories at a high, more abstract level with no need to generate detailed memories.”
Siegelmann took this information and joined her colleagues in order to develop a brain-like replay with artificial intelligence, where there was no data stored. As is the case for the human brain, the artificial network takes what it has seen before in order to generate high-level representations.
The method was highly efficient, with even just a few replayed generated representations resulting in older memories being remembered while new ones were learned. Generative replay is effective at preventing catastrophic forgetting, and one of the major benefits is that it allows the system to generalize from one situation to another.
According to van de Ven, “If our network with generative replay first learns to separate cats from dogs, and then to separate bears from foxes, it will also tell cats from foxes without specifically being trained to do so. And notably, the more the system learns, the better it becomes at learning new tasks.”
“We propose a new, brain-inspired variant of replay in which internal or hidden representations are replayed that are generated by the network’s own, context-modulated feedback connections,” the team writes. “Our method achieved state-of-the-art performance on challenging continual learnings benchmarks without storing data, and it provides a novel model for abstract replay in the brain.”
“Our method makes several interesting predictions about the way replay might contribute to memory consolidation in the brian,” Van de Ven continues. “We are already running an experiment to test some of these predictions.”
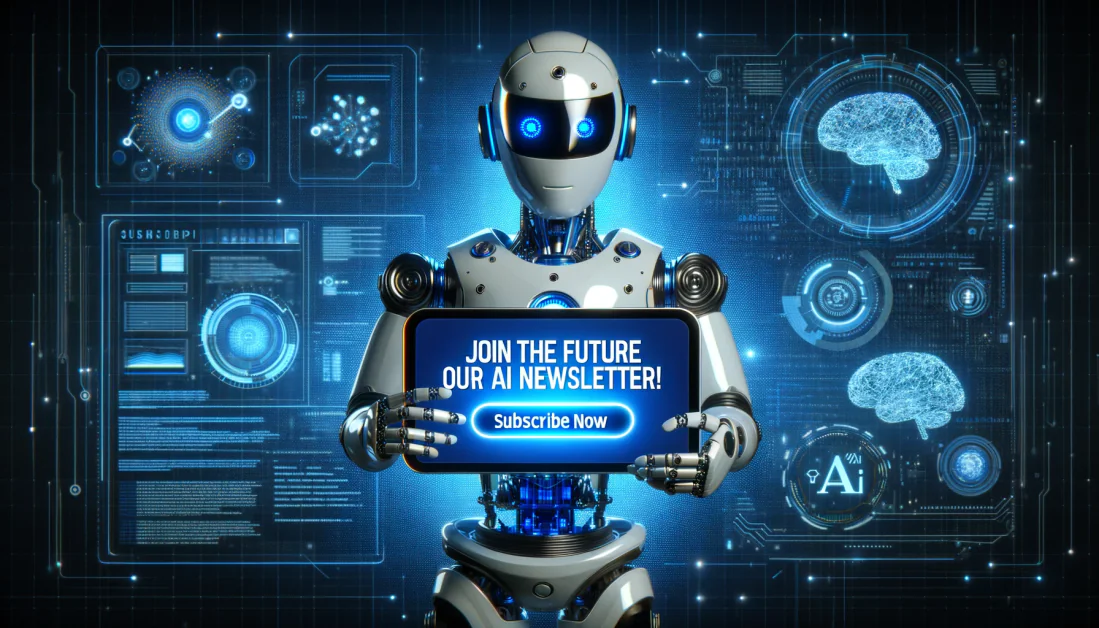
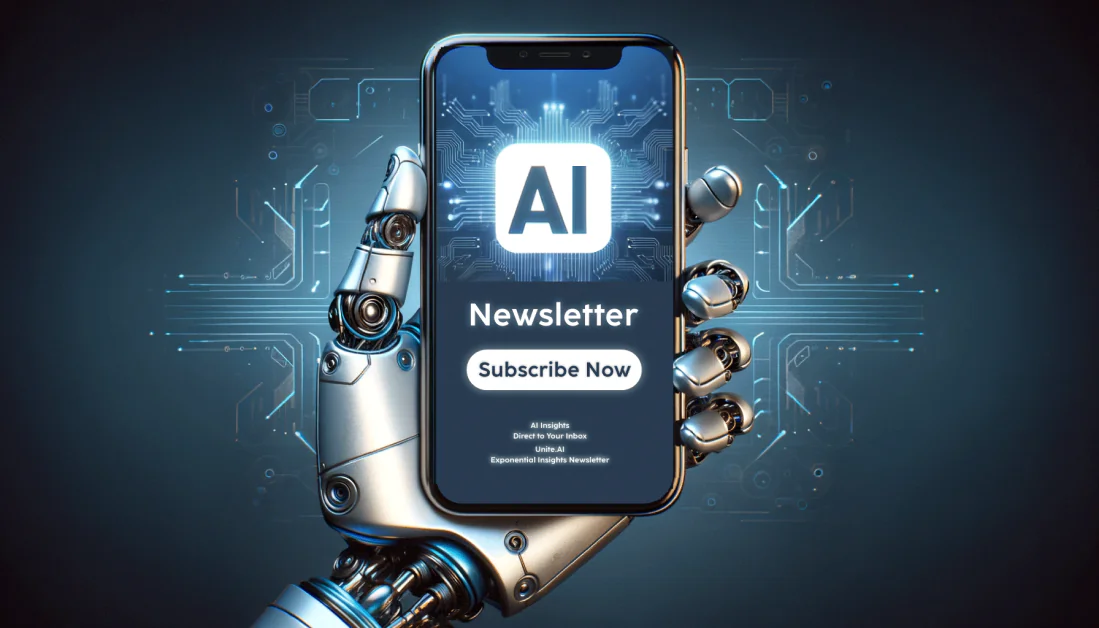