Artificial Intelligence
Disney Combines CGI With Neural Rendering to tackle the ‘Uncanny Valley’
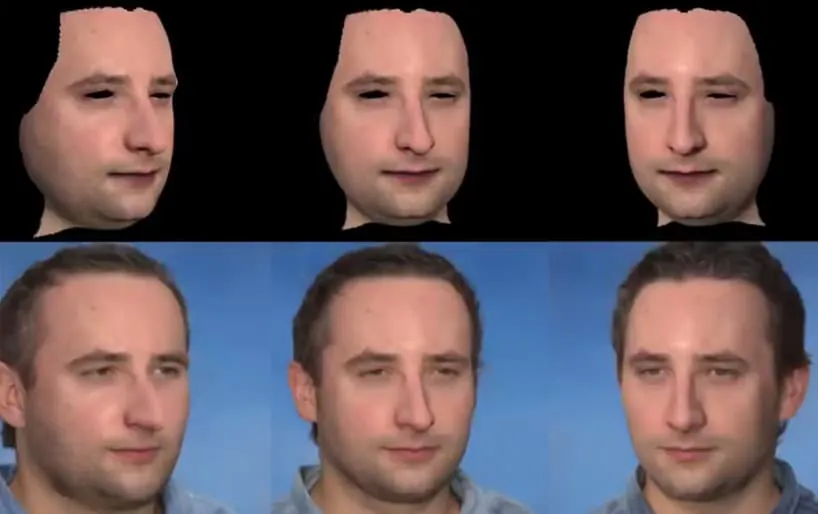
Disney's AI research division has developed a hybrid method for movie-quality facial simulation, combining the strengths of facial neural rendering with the consistency of a CGI-based approach.
The pending paper is titled Rendering with Style: Combining Traditional and Neural Approaches for High Quality Face Rendering, and is previewed in a new 10-minute video at the Disney Research YouTube channel (embedded at end of this article*).
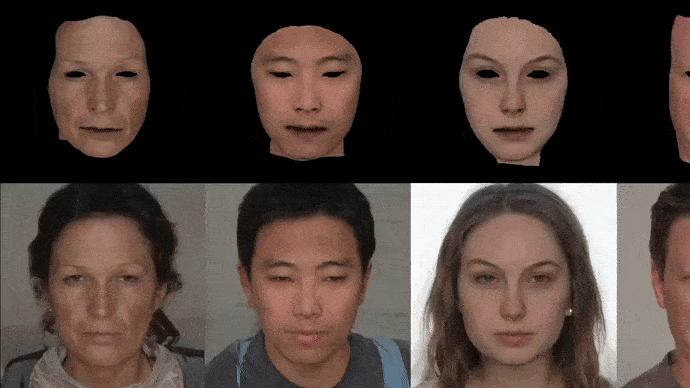
Meshes combined with neural facial renders. See video embed at end of article for better detail and quality. Source: https://www.youtube.com/watch?v=k-RKSGbWLng (since replaced by https://www.youtube.com/watch?v=TwpLqTmvqVk)
As the video notes, neural rendering of faces (including deepfakes) can produce far more realistic eyes and mouth interiors than CGI is capable of, while CGI-driven facial textures are more consistent and suitable for cinema-level VFX output.
Therefore Disney is experimenting with letting NVIDIA's StyleGan2 neural generator handle the surrounding features of a face and the ‘life-critical' elements such as eyes, while superimposing consistent CGI facial skin and related elements into the output.
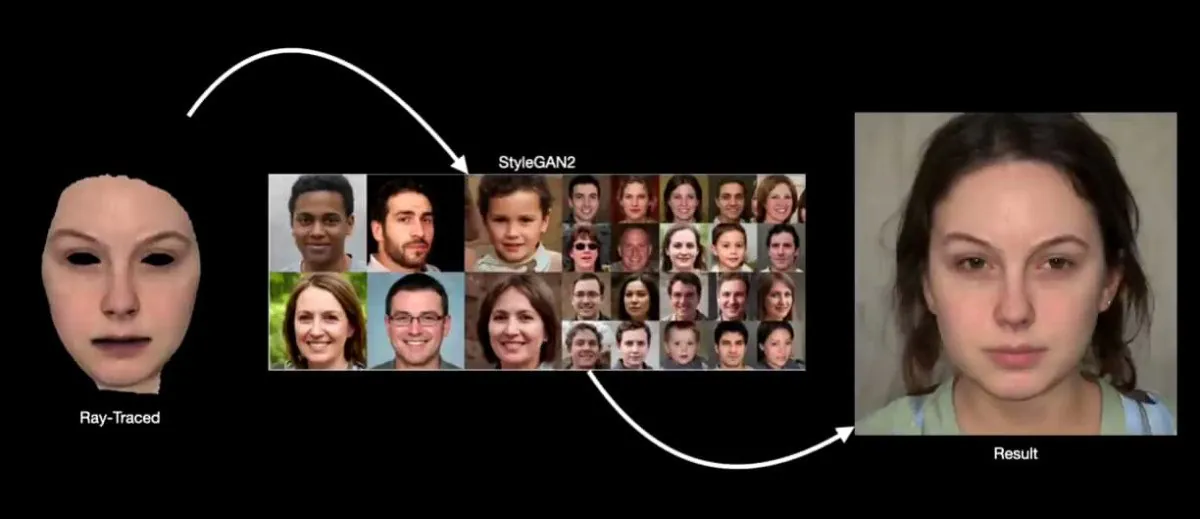
From the video (see end of article), the architectural concept behind Disney's hybrid approach, where an old-school CGI mesh, of the type used to recreate ‘young' Carrie Fisher and the late Peter Cushing for Rogue One (2016), is integrated into neurally-rendered face environments.
The video makes a tacit reference to frequent criticism of the inauthenticity and ‘uncanny valley' effect of the CGI recreation of late British Star Wars actor Peter Cushing in Rogue One (2016), conceding:
‘[There's] still a huge gap between what people can easily capture and render versus final photorealistic digital doubles, complete with hair, eyes and inner mouth. To close this gap, it usually takes a lot of manual work from skilled artists.'
In truth, even the most modern facial capture systems do not even attempt to recreate eyes, mouth interiors or hair, which either have issues of authenticity in such techniques (eyes) or else of temporal consistency (hair).
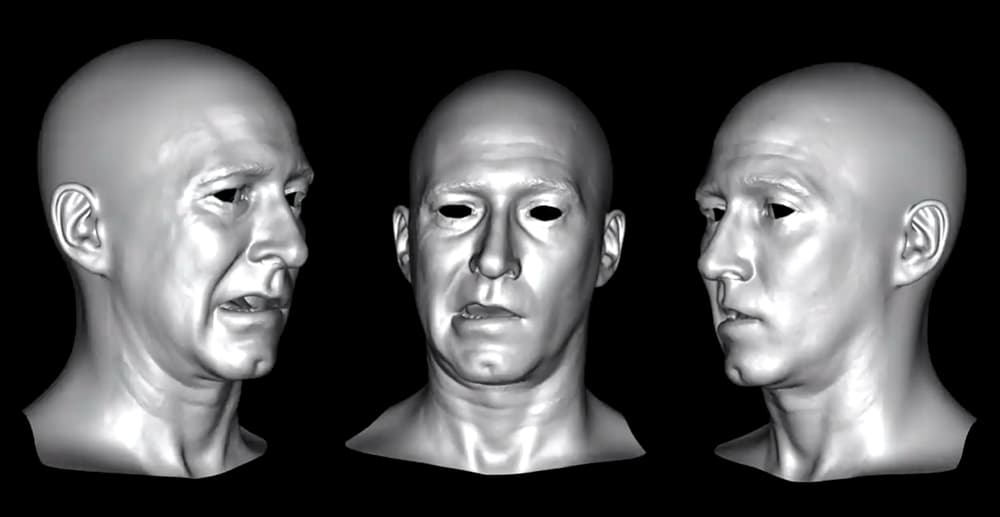
The video illustrates what VFX artists will get after a typical modern facial capture session. Eyes, hair, facial hair, and mouth interiors will all have to be handled by separate teams in the production pipeline, in addition to texturing and lighting.
Illumination Control
The hybrid approach is also a benefit with relighting – a notable challenge for neural rendering of faces, since CGI skin superimpositions can be more easily relit.
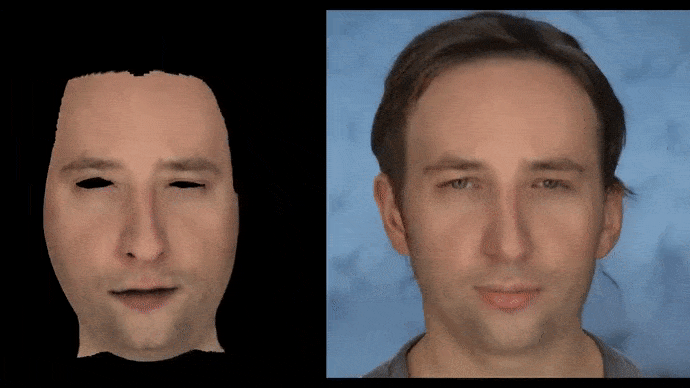
An animated version of the CGI/Neural approach.
In more challenging environments, such as exterior shoots, the researchers have developed a method of inpainting around a kind of demilitarized zone surrounding the person being ‘created'.
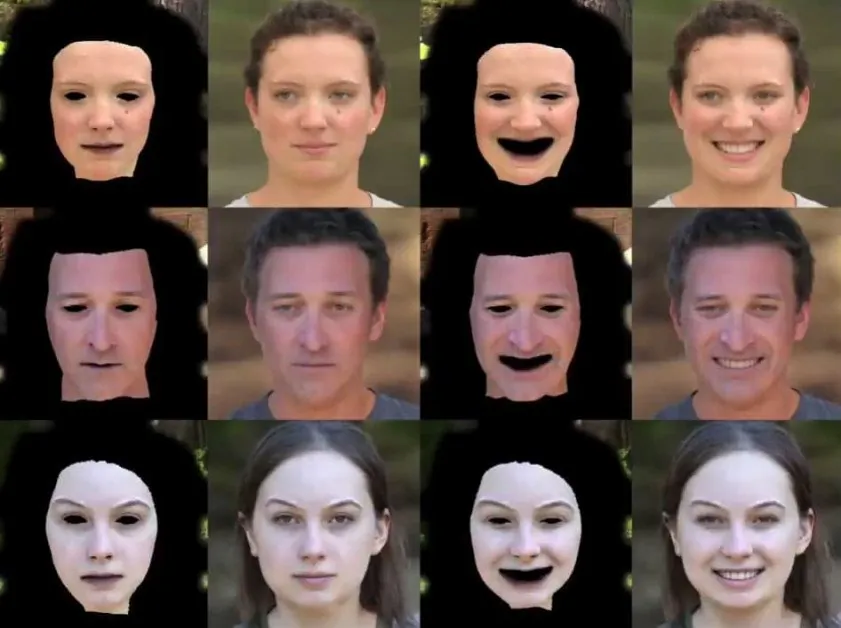
A black margin is generated to allow a ‘canvas' for inpainting the outer parts of the identity and integrating the CGI skin into the combined CGI/neural output.
The video notes:
‘[The] neural render does not match the background constraint perfectly. – it's only meant as a guide, since optimizing for realistic human components like the hair, eyes and teeth is the main goal. More challenging is to try and maintain a consistent identity, while changing the environment lighting.'
Creating CGI Meshes From Neural Renders
The research team have also developed a variational autoencoder trained on a (unspecified) large database of 3D face images, and claims that it can produce ‘random but plausible' 3D face meshes from ground truth data.
There are limitations for this research to overcome, including the difficulty in getting hair to stay temporally consistent in the neural renderings, and the video (see below) shows several examples of rapidly mutating hair in an otherwise consistent pan around a CGI/neural face.
Temporal consistency in neural video rendering is a far wider problem than just Disney's, and it seems likely that later iterations of this system may resort to adding hair ‘in post', or various other possible approaches to hair generation than hoping a novel neural approach will eventually solve it.
Uses for Dataset Generation
The method is proposed also as a potential method of generating synthetic data, and enriching the facial image set landscape, which has in recent years become dangerously monotonous.
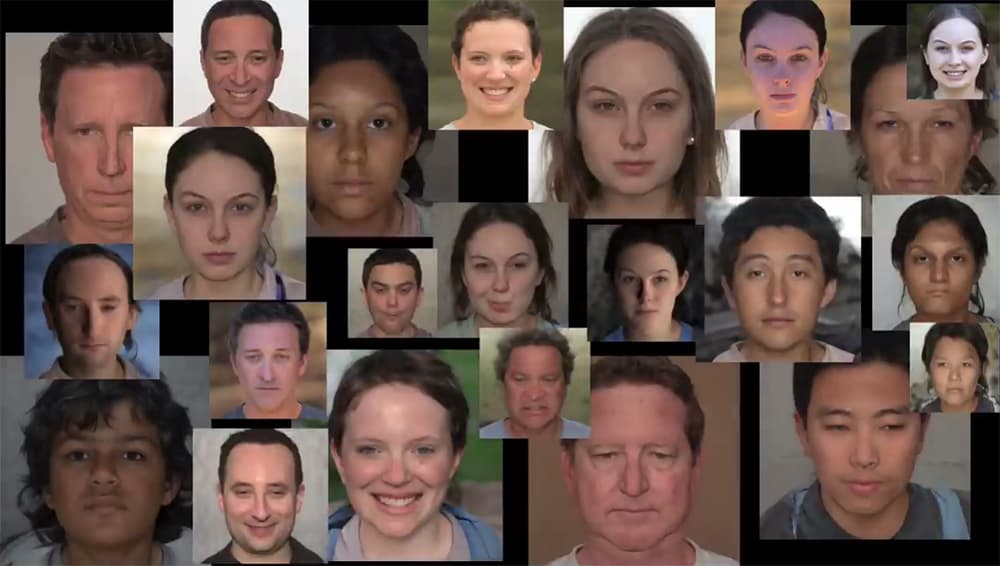
Disney envisages the new technique populating facial image datasets.
‘[Every] photorealistic result we generate has an underlying corresponding geometry, and appearance maps, rendered from unknown camera viewpoints with known illumination. This ‘ground truth' information can be vital for training downstream applications, such as monocular, 3D face reconstruction, facial recognition, or scene understanding. And so every results render could be considered a data sample, and we can generate many variations of many different individuals.
‘Furthermore, even for a single person rendered in a single expression with a single viewpoint and illumination, we can generate random variations of the photo-real render by varying the randomization seed during optimization.'
The researchers note that this diversity of configurable output could be useful in training facial recognition applications, concluding:
‘[Our] method is able to leverage current technology for facial skin capture, modeling and rendering, and automatically create complete photorealistic face renders that match the desired identity, expression and scene configuration. This approach has applications and facial rendering for film and entertainment, saving manual artists labor and also for data generation in different fields of deep learning.'
For a deeper look at the new approach, check out the 10-minute video released today:
* The original video link was substituted for another apparently identical one 8 hours after this article was published. I changed all relevant links, as there is no trace of the original video.
8:24 GMT+2 – Replaced video, as it was switched out by the Disney Research YouTube channel for some reason.
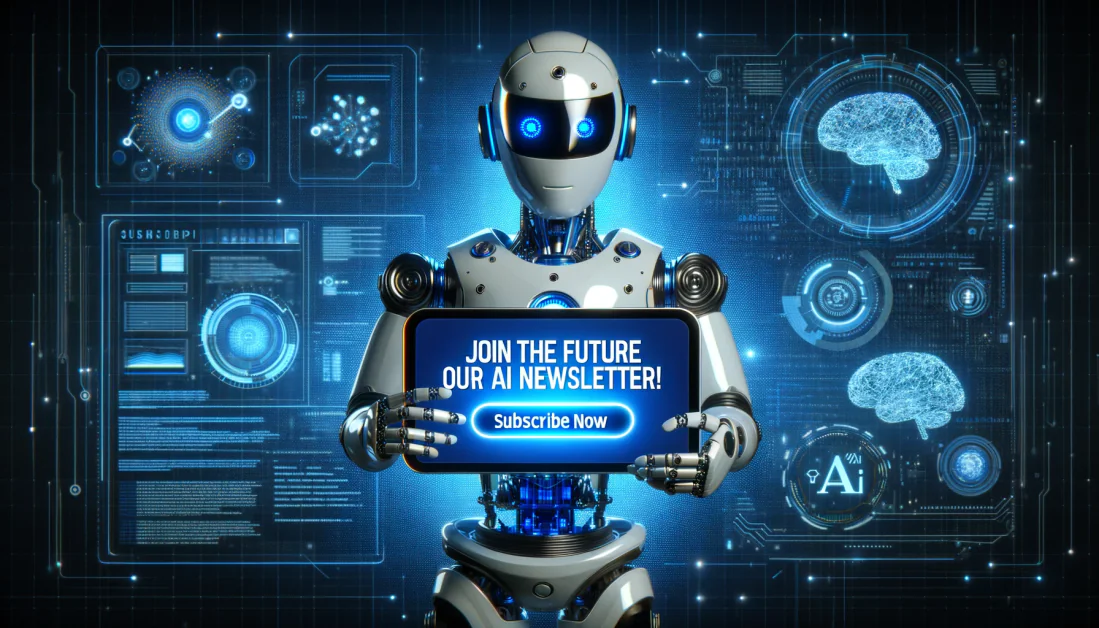
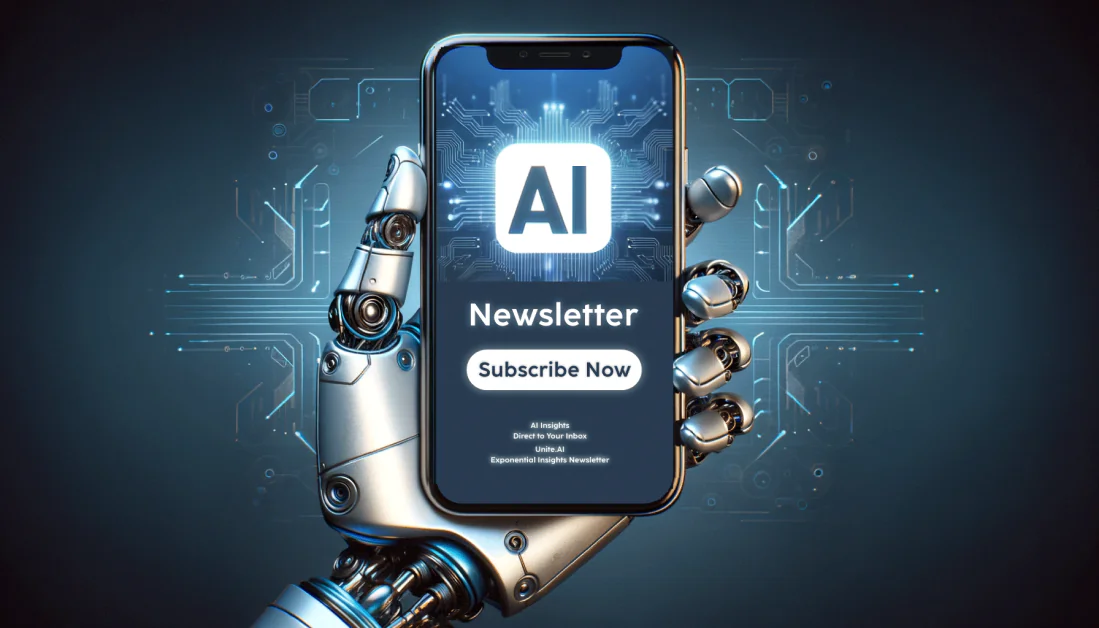