Artificial Intelligence
SofGAN: A GAN Face Generator That Offers Greater Control
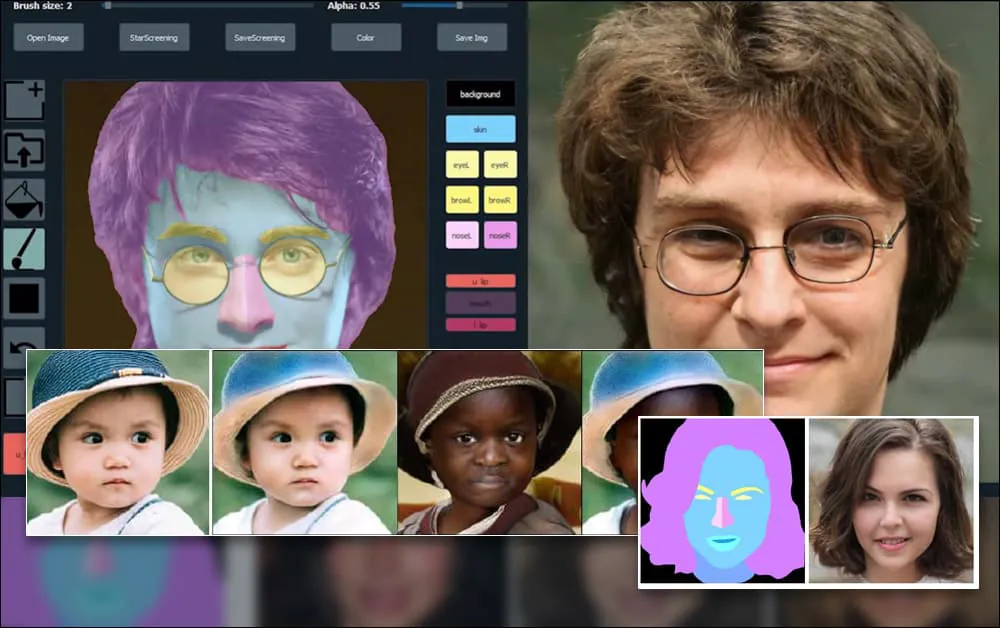
Researchers in Shanghai and the US have developed a GAN-based portrait generation system that allows users to create novel faces with a hitherto unavailable level of control over individual aspects such as hair, eyes, glasses, textures and color.
To demonstrate the versatility of the system, the creators have provided a Photoshop-style interface wherein a user can directly draw semantic segmentation elements that will be re-interpreted into realistic imagery, and which can even be obtained by drawing directly over existing photographs.
In the example below, a picture of actor Daniel Radcliffe is used as a tracing template (and the objective is not to produce a likeness of him, but rather a generally photorealistic image). As the user fills in various elements, including discrete facets such as glasses, they are identified and interpreted in the output drawing image:
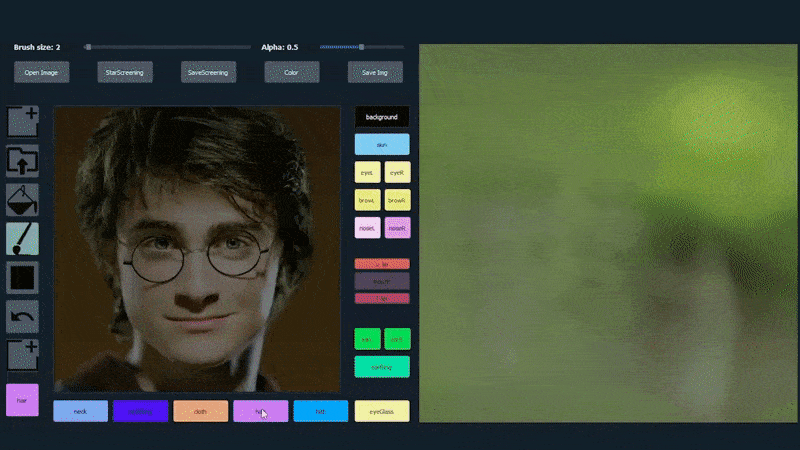
Using one image as tracing material for a SofGAN-generated portrait. Source: https://www.youtube.com/watch?v=xig8ZA3DVZ8
The paper is entitled SofGAN: A Portrait Image Generator with Dynamic Styling, and is led by Anpei Chen and Ruiyang Liu, along with two other researchers from ShanghaiTech University and another from the University of California at San Diego.
Disentangling Features
The primary contribution of the work is not so much in providing a user-friendly UX, but rather in ‘disentangling' characteristics of learned facial features, such as pose and texture, which allows SofGAN to also generate faces that are at indirect angles to the camera viewpoint.
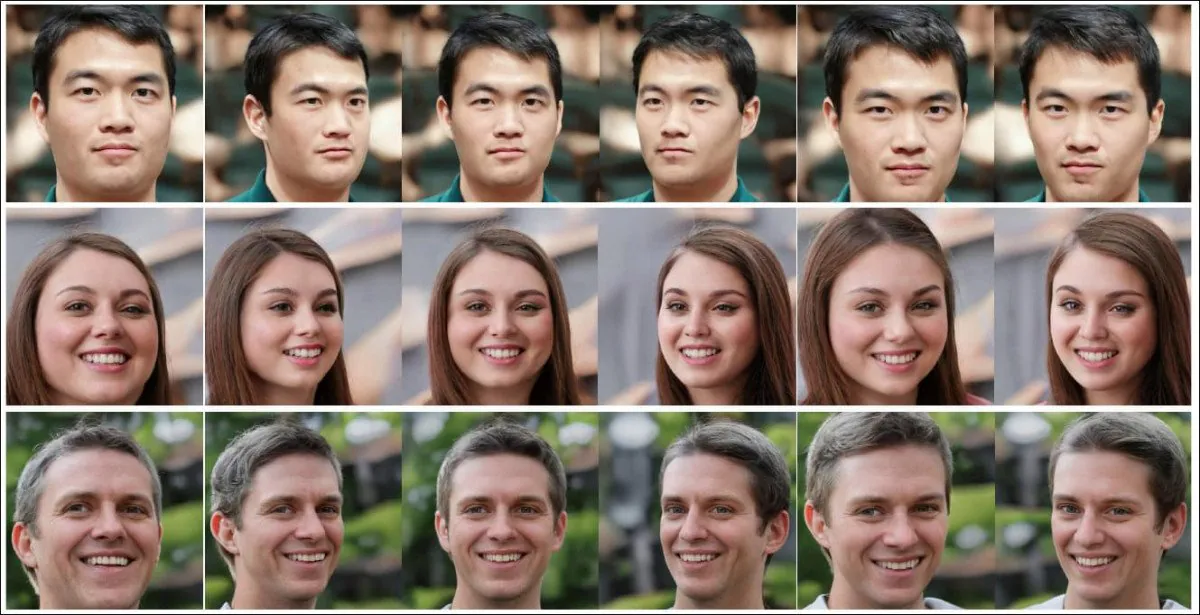
Unusual among facial generators based on Generative Adversarial Networks, SofGAN can change the angle of view at will, within the limits of the array of angles present in the training data. Source: https://arxiv.org/pdf/2007.03780.pdf
Since textures are now disentangled from geometry, face shape and texture can also be manipulated as separate entities. In effect, this allows race-changing of a source face, a scandalous practice that now has a potentially useful application, for the creation of racially-balanced machine learning datasets.
SofGAN also supports artificial ageing and attribute-consistent style adjustment at a granular level unseen in similar segmentation>image systems such as NVIDIA's GauGAN and Intel's game-based neural rendering system.
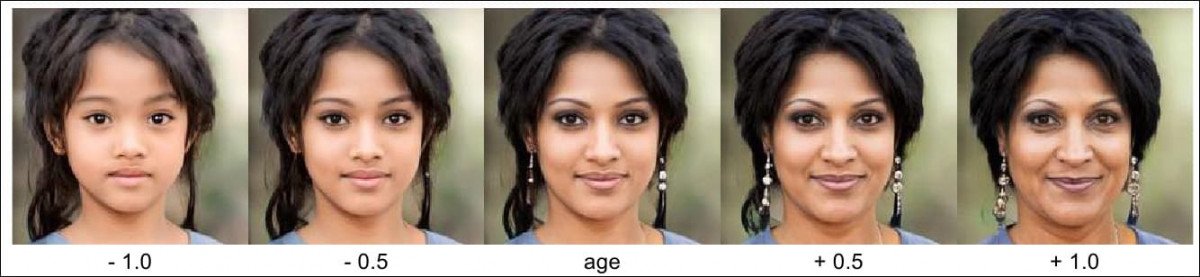
SofGAN is able to implement ageing as an iterative style.
Another breakthrough for SofGAN's methodology is that the training does not require paired segmentation/real images, but rather can be directly trained on unpaired real world images.
The researchers state that the ‘disentangling' architecture of SofGAN was inspired by traditional image rendering systems, which decompose the individual facets of an image. In visual effects workflows, the elements for a composite are routinely broken down to the most minute components, with specialists dedicated to each component.
Semantic Occupancy Field (SOF)
To achieve this in a machine learning image synthesis framework, the researchers developed a semantic occupancy field (SOF), an extension of the traditional occupancy field which individuates the component elements of facial portraits. The SOF was trained on calibrated multi-view semantic segmentation maps, but without any ground truth supervision.
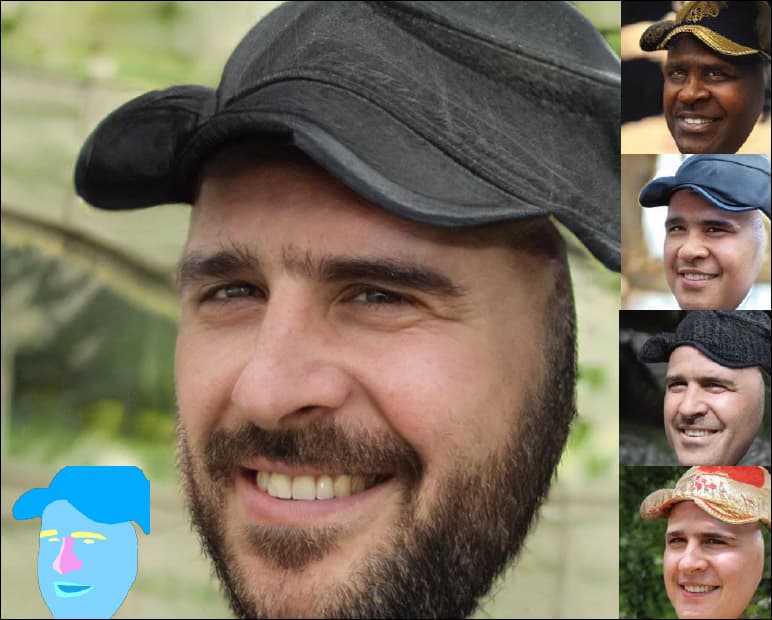
Multiple iterations from a single segmentation map (lower left).
Additionally, 2D segmentation maps are obtained by ray-tracing the output of the SOF, before being textured by a GAN generator. The ‘synthetic' semantic segmentation maps are also encoded in a low dimensional space via a three-layer encoder to ensure continuity of output when the viewpoint is changed.
The training scheme spatially mixes two random styles for each semantic region:
The researchers claim that SofGAN achieves a lower Frechet Inception Distance (FID) than the current alternative state of the art (SOTA) approaches, as well as a higher Learned Perceptual Image Patch Similarity (LPIPS) metric.
Previous StyleGAN approaches have frequently been hindered by feature entanglement, wherein the elements that compose an image are irretrievably bound up with each other, causing unwanted elements to appear alongside a desired element (i.e. ear-rings might appear when an ear shape is rendered that was informed at training time by a picture that featured ear-rings).
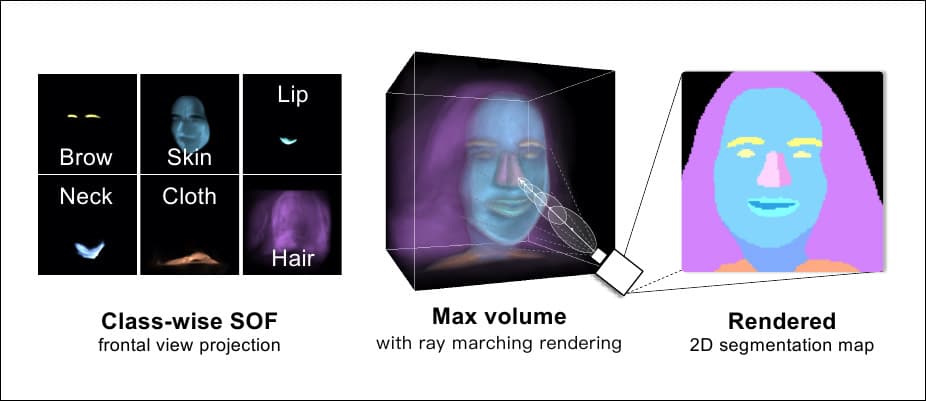
Ray marching is used to calculate the volume of semantic segmentation maps, enabling multiple viewpoints.
Datasets and Training
Three datasets were used in the development of various implementations of SofGAN: CelebAMask-HQ, a repository of 30,000 high resolution images taken from the CelebA-HQ dataset; NVIDIA's Flickr-Faces-HQ (FFHQ), which contains 70,000 images, where the researchers labelled the images with a pre-trained face parser; and a self-produced group of 122 portrait scans with manually labeled semantic regions.
The SOF is comprised of three trainable sub-modules – the hyper-net, a ray marcher (see image above), and a classifier. The project's Semantic Instance Wised (SIW) StyleGAN generator is configured similarly to StyleGAN2 in certain aspects. Data augmentation is applied through random scaling and cropping, and training features path regularization every four steps. The entire training procedure took 22 days to reach 800,000 iterations on four RTX 2080 Ti GPUs over CUDA 10.1.
The paper does not mention the configuration of the 2080 cards, which can accommodate between 11gb-22gb VRAM each, meaning that the total VRAM employed for the best part of a month to train SofGAN is somewhere between 44Gb and 88Gb.
The researchers observe that acceptable generalized, high-level results began to emerge quite early in the training, at 1500 iterations, three days into training. The remainder of the training was taken up with the predictable, slow crawl towards the obtaining of fine detail such as hair and eye facets.
SofGAN generally achieves more realistic results from a single segmentation map than rival methods such as NIVDIA's SPADE and Pix2PixHD, and SEAN.
Below is the video released by the researchers. Further self-hosted videos are available at the project page.
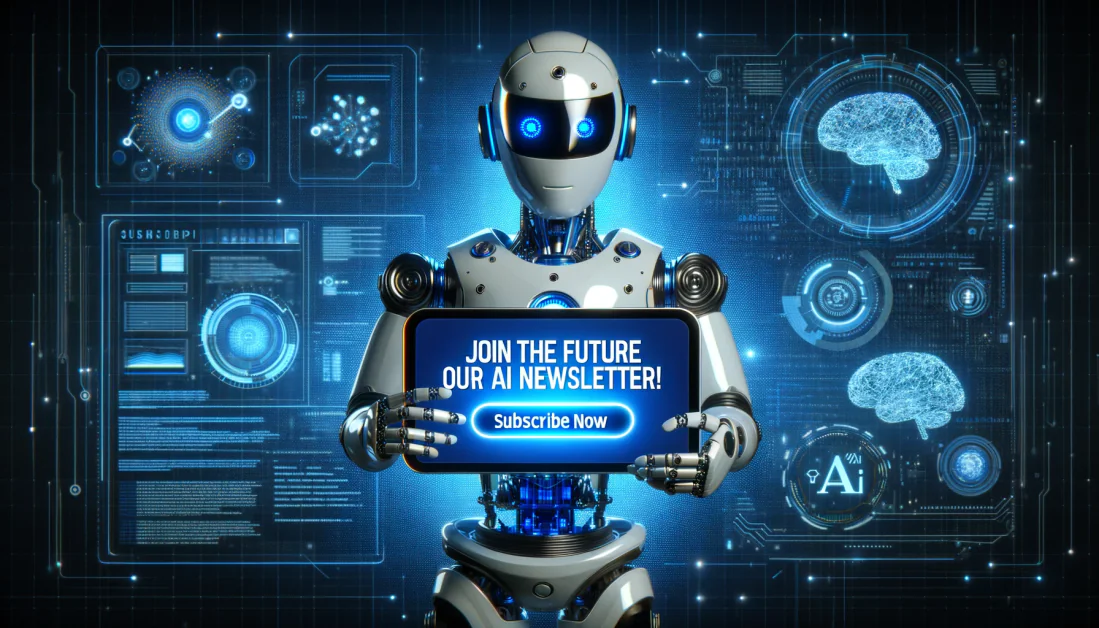
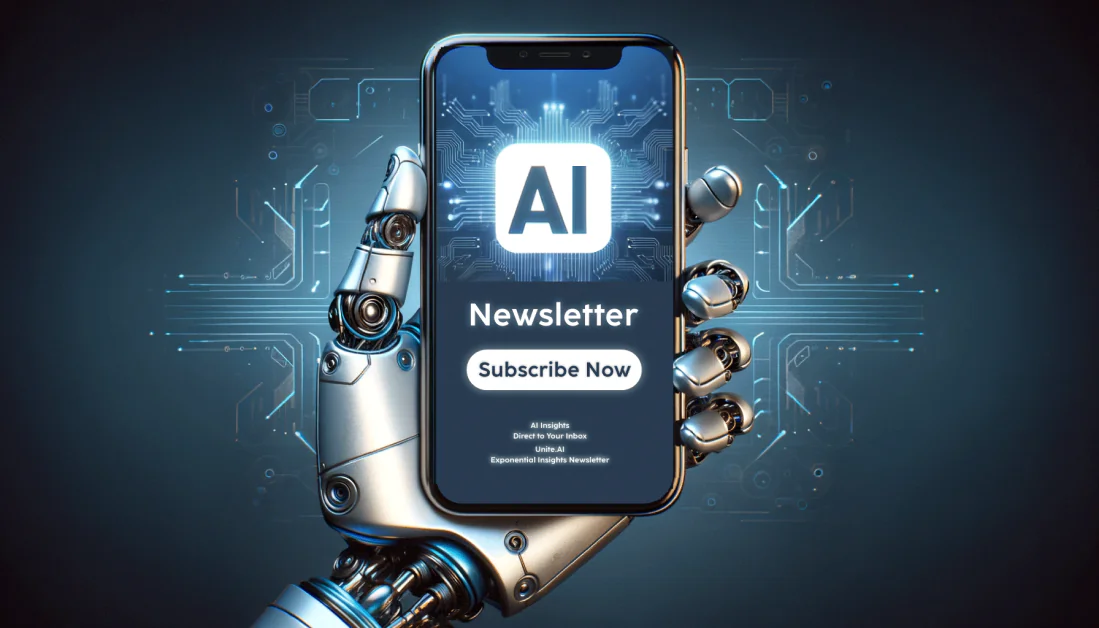