Healthcare
Determining Intoxication With Machine Learning Analysis of Eyes
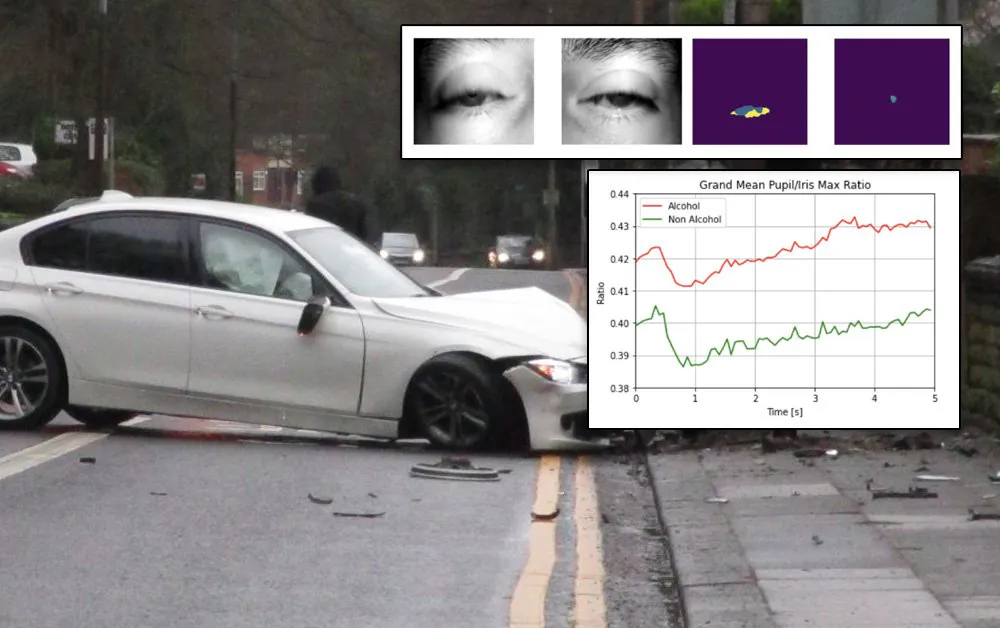
Researchers from Germany and Chile have developed a new machine learning framework capable of evaluating whether a person is intoxicated, based on near infra-red images of their eyes.
The research is aimed at the development of ‘fitness for duty' real-time systems capable of assessing the readiness of an individual to perform critical tasks such as driving, or operating machinery, and uses a novel and scratch-trained object detector that can individuate a subject's eye components from a single image and evaluate them against a database that includes intoxicated and non-intoxicated eye images.
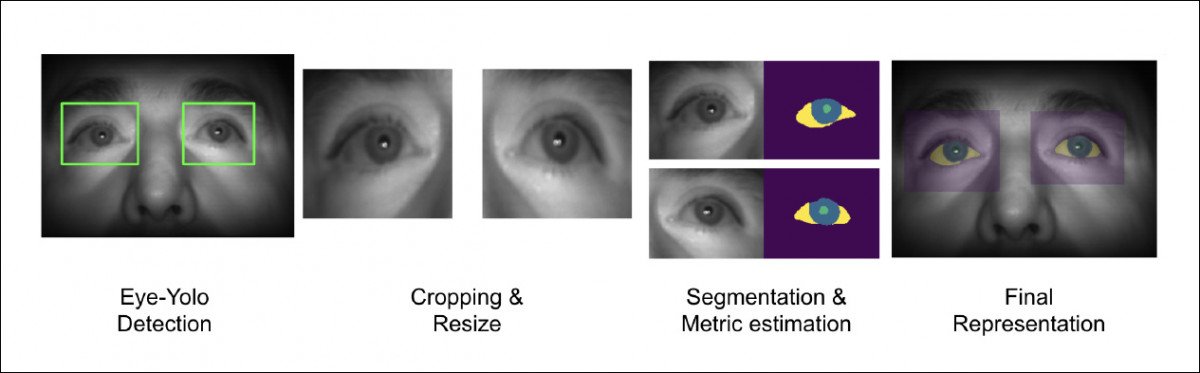
You Only Look Once (YOLO) individuates the subject's eyes, after which the framework separates the instances and performs segmentation to break the eye image down into its constituent parts. Source: https://arxiv.org/pdf/2106.15828.pdf
Initially the system captures and individuates an image of each eye with the You-Only-Look-Once (YOLO) object detection framework. After this, two optimized networks are used to break down the eye images into semantic regions – the Criss Cross attention network (CCNet) released in 2020 by the Huazhong University of Science and Technology, and the DenseNet10 segmentation algorithm, also developed by several of the new paper's researchers at Chile.
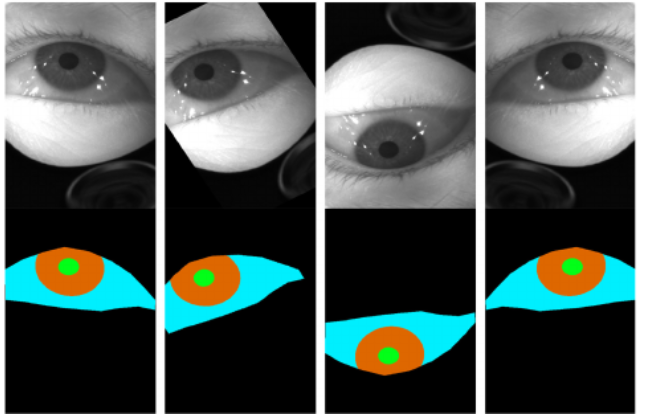
Segmentation obtained from near infra-red eye images. Source: https://www.researchgate.net/publication/346903035_Towards_an_Efficient_Segmentation_Algorithm_for_Near-Infrared_Eyes_Images#pf6
The two algorithms employ only 122,514 and 210,732 parameters respectively – a frugal outlay, in comparison with some of the larger feature-sets in similar models, and bucking the general trend towards higher volumes of data in ML frameworks.
Database of the Drunk
To inform the machine learning framework, the researchers developed an original database featuring 266 intoxicated subjects and 765 sober subjects.
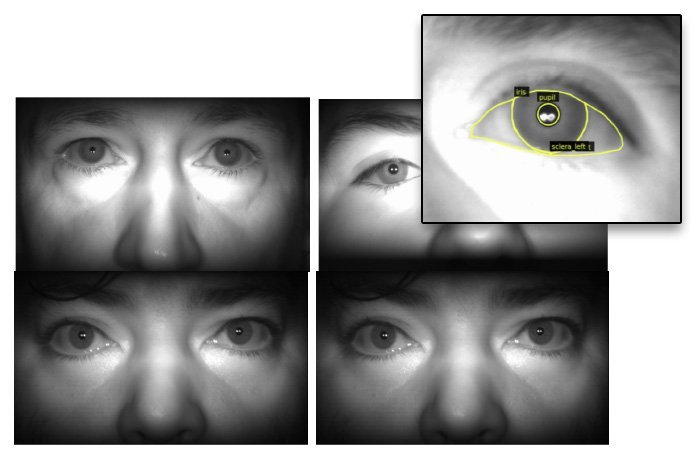
Samples from the obtained database of intoxicated and non-intoxicated subjects.
The subjects were required to stand in front of two originating Iritech cameras, the Gemini/Venus range, to face the device, and to be recorded sober. After this they consumed 200ml of alcohol, and were re-captured at 15-minute intervals as their levels of blood-alcohol rose, up to the final session at 60 minutes since consumption of the alcohol.
This produced 21,309 images, which were then annotated using the Python library imgaug.
Preparing the Data for the Real World
It was not a highly automated workflow, despite the advanced tools used – manual labeling of the eye images was described by the researchers as ‘a very demanding and time-consuming process', and took more than a year.
The data was aggressively augmented with a series of methods designed to degrade and challenge the system, replicating possible real world conditions, including snowflakes, Poisson noise (to simulate low-light sensor degrading), blur, spatter and rain effects. Besides this, the use of infra-red capture obviates the need for ideal lighting conditions, which can't be guaranteed in economical and practical deployments.
This grueling work eventually paid off with a 98,60% level of accuracy for eye capture and segmentation.
Testing
The segmentation framework was tested with five platforms: Osiris, DeepVOG, DenseNet10 (see above), CCNet (see above), and Grand-Mean. In all cases, the analysis demonstrated successful results for correlating pupil dilation to level of drunkenness, though a hybrid approach using DenseNet and CCNet proved the most effective.
The researchers anticipate that their work can eventually be incorporated into a standard NIR iris sensor, and note that the Herculean effort of producing the contributing intoxicated eye database is a likely benefit to this sector of biometric research.
Consumer and Industrial Intoxication Testing Through Eye Evaluation
The new research builds on some notable prior literature, including a seminal 2015 paper from researchers in Brazil and the US, which proposed a systematic and rationalized method of evaluating intoxication from pupillary response. Researchers for that paper observed that alcohol reduces brain efficiency and impairs night vision by a factor of 25%, and reaction time by 30%, with varying levels of severity according to the individual's tolerance levels.
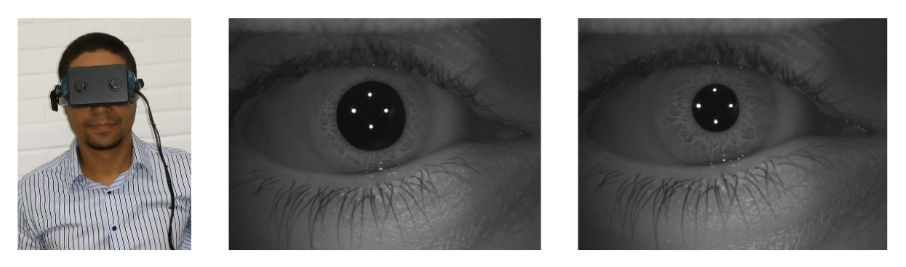
Source: https://pixellab.group/publication/2015/pinheiro2015/pinheiro2015.pdf
The primary issue for diffusion of such technologies is portability. As early as 2003, UK research company Hampton Knight offered a system for intoxication evaluation through eye analysis – though it cost £10,000 sterling at the time.
A preliminary 2012 study out of New Delhi and the USA also explored the possibility of using systematic AI techniques to derive an intoxication score from ocular images, though with less success than the current research. That study also contributed a valuable dataset (IITD Iris Under Alcohol Influence) to the body of work in this field.
However, recent innovations in edge computing and optimized mobile machine learning hardware resources open up the field for far more mobile applications of pre-activity checks for intoxication, including in-car sensors that could potentially add iris checks to the current methods that are of interest to the Driver Alcohol Detection System for Safety (DADSS) framework being developed in the US – which to date has relied on skin-alcohol sensors and the evaluation of in-vehicle air for alcohol vapor.
A 2020 report estimated that the adoption of technologies of this type could save 11,000 lives a year in the US alone.
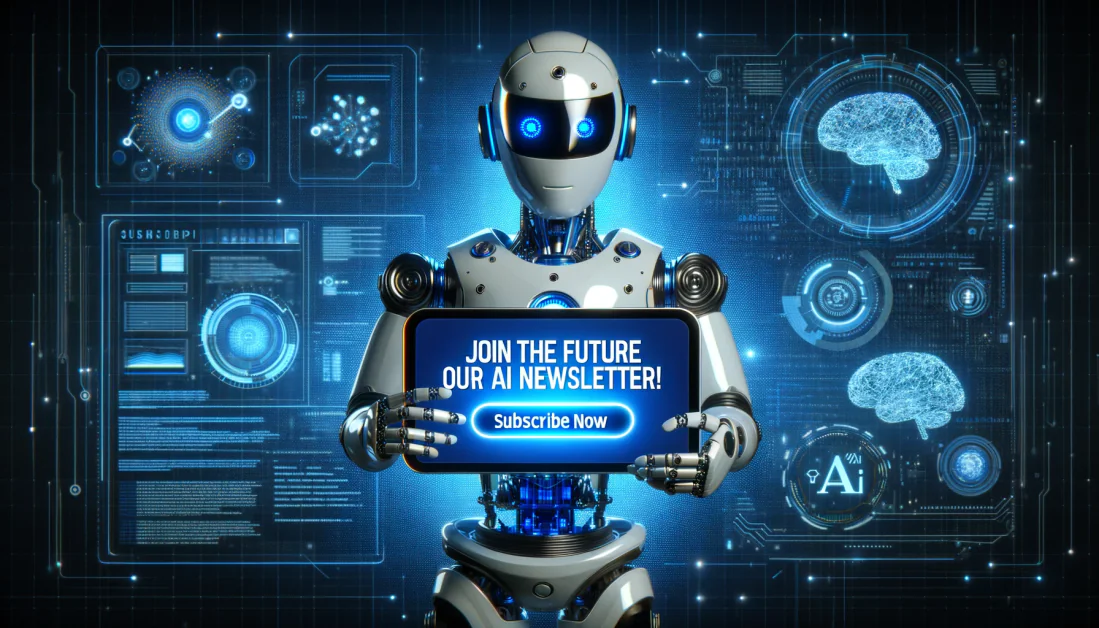
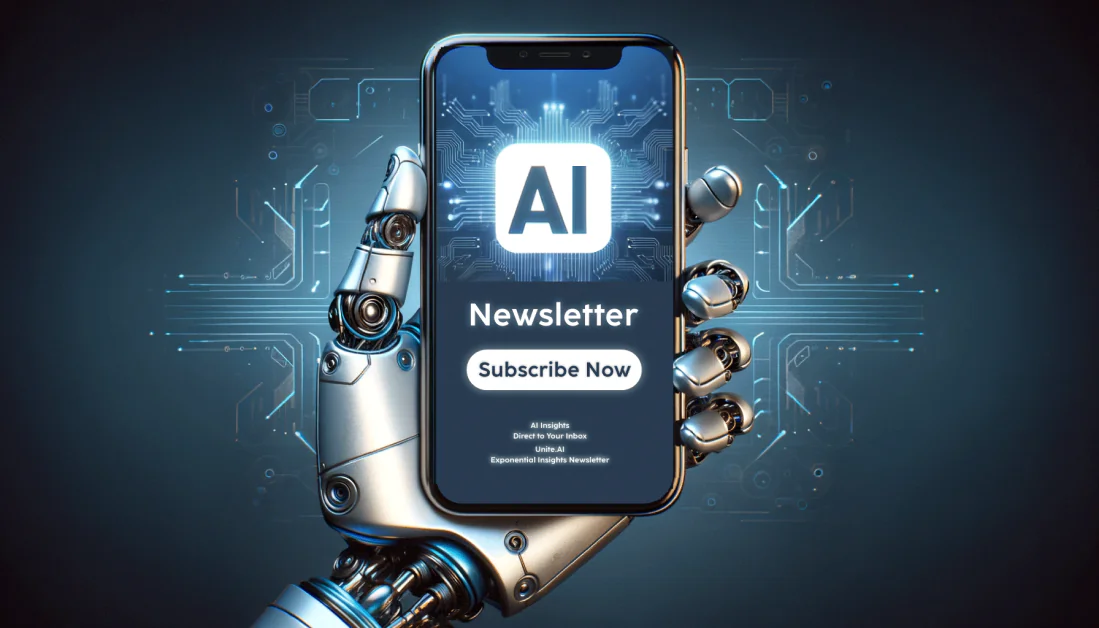