Artificial Intelligence
DeepMind Discovers AI Training Technique That May Also Work In Our Brains
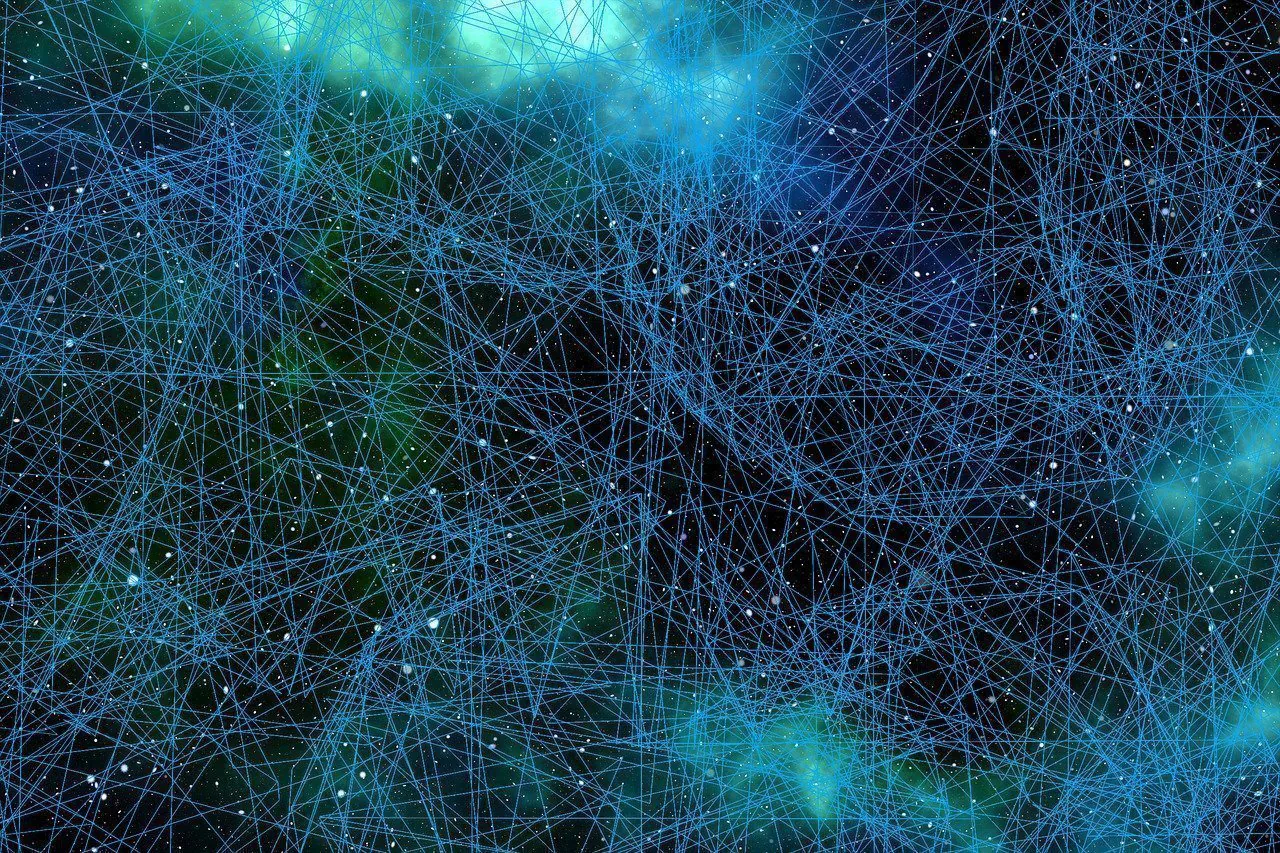
DeepMind just recently published a paper detailing how a newly developed type of reinforcement learning could potentially explain how reward pathways within the human brain operate. As reported by NewScientist, the machine learning training method is called distributional reinforcement learning and the mechanisms behind it seem to plausibly explain how dopamine is released by neurons within the brain.
Neuroscience and computer science have a long history together. As far back as 1951, Marvin Minksy used a system of rewards and punishments to create a computer program capable of solving a maze. Minksy was inspired by the work of Ivan Pavlov, a physiologist who demonstrated that dogs could learn through a series of rewards and punishments. Deepmind’s new paper adds to the intertwining history of neuroscience and computer science by applying a type of reinforcement learning to gain insight into how dopamine neurons might function.
Whenever a person, or animal, is about to carry out an action, the collections of neurons in their brain responsible for the release of dopamine make a prediction about how rewarding the action will be. Once the action has been carried out and the consequences (rewards) of that action made apparent, the brain releases dopamine. However, this dopamine release is scaled in accordance with the size of the error in prediction. If the reward is larger/better than expected, a stronger surge of dopamine is triggered. In contrast, a worse reward leads to less dopamine being released. The dopamine serves as a corrective function that makes the neurons tune their predictions until they converge on the actual rewards being earned. This is very similar to how reinforcement learning algorithms operate.
The year 2017 saw DeepMind researchers release an enhanced version of a commonly used reinforcement learning algorithm, and this superior learning method was able to boost performance on many reinforcement learning tasks. The DeepMind team thought that the mechanisms behind the new algorithm could be used to better explain how dopamine neurons operate within the human brain.
In contrast to older reinforcement learning algorithms, DeepMind’s newer algorithm represents rewards as a distribution. Older reinforcement learning approaches represented estimated rewards as just a single number that stood for the average expected result. This change allowed the model to more accurately represent possible rewards and perform better as a result. The superior performance of the new training method prompted the DeepMind researchers to investigate if dopamine neurons in the human brain operate in a similar fashion.
In order to investigate the workings of dopamine neurons, DeepMind worked alongside Harvard to research the activity of dopamine neurons in mice. The researchers had the mice perform various tasks and gave them rewards based on the roll of dice, recording how their dopamine neurons fired. Different neurons seemed to predict different potential results, releasing different amounts of dopamine. Some neurons predicted lower than the actual reward while some predicted rewards higher than the actual reward. After graphing out the distribution of the reward predictions, the researchers found that the distribution of predictions was fairly close to the genuine reward distribution. This suggests that the brain does make use of a distributional system when making predictions and adjusting predictions to better match reality.
The study could inform both neuroscience nad computer science. The study supports the use of distributional reinforcement learning as a method of creating more advanced AI models. Beyond that, it could have implications for our theories of how the brain operates regarding reward systems. If dopamine neurons are distributed and some are more pessimistic or optimistic than others, understanding these distributions could alter how we approach aspects of psychology like mental health and motivation.
As MIT Technology View reported, Matt Botvinik, the director of neuroscience research at DeepMind, explained the importance of the findings at a press briefing. Botvinik said:
“If the brain is using it, it’s probably a good idea. It tells us that this is a computational technique that can scale in real-world situations. It’s going to fit well with other computational processes. It gives us a new perspective on what's going on in our brains during everyday life”
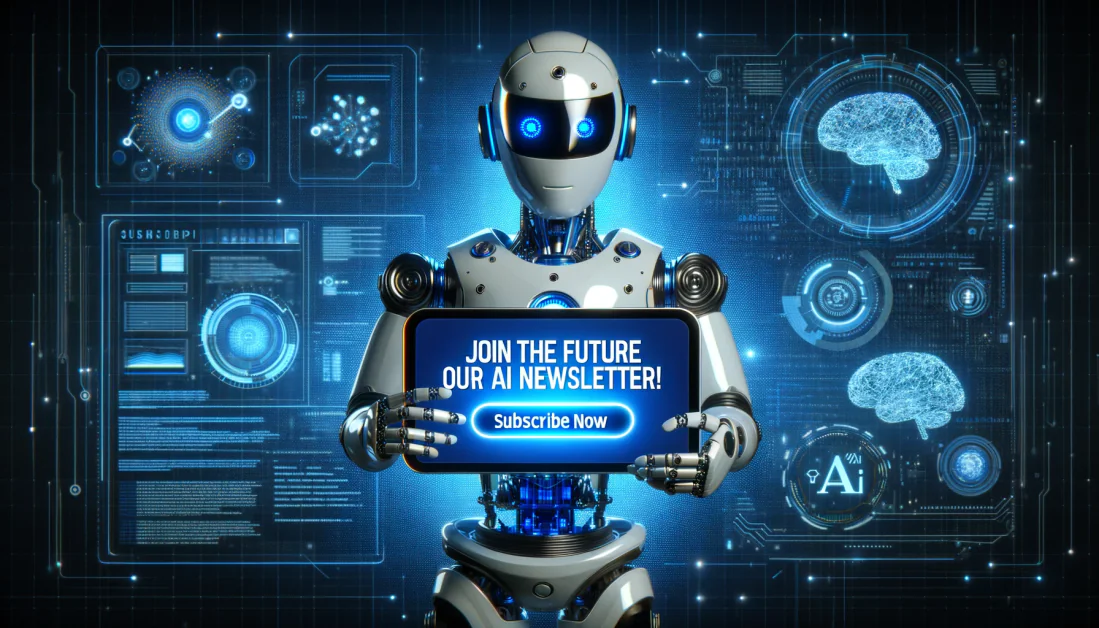
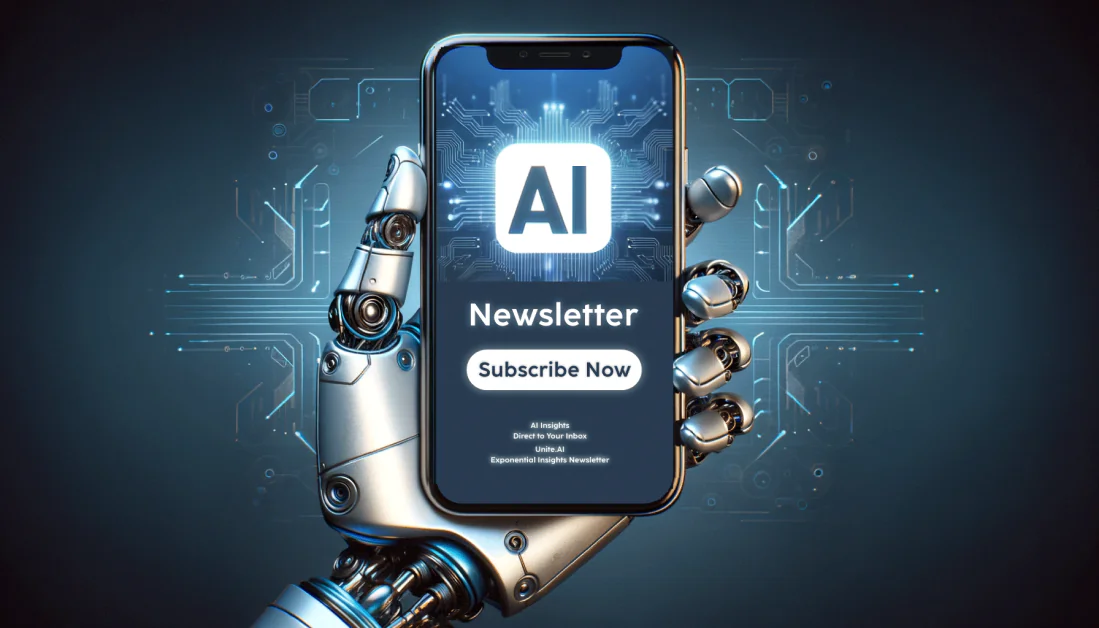