Artificial Intelligence
DeepMind Creates AI That Replays Memories Like The Hippocampus
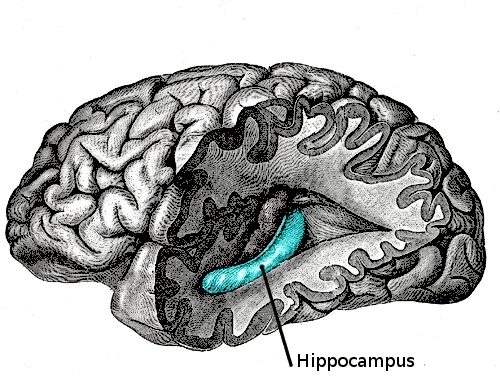
The human brain often recalls past memories (seemingly) unprompted. As we go throughout our day, we have spontaneous flashes of memory from our lives. While this spontaneous conjuration of memories has long been of interest to neuroscientists, AI research company DeepMind recently published a paper detailing how an AI of theirs replicated this strange pattern of recall.
The conjuration of memories in the brain, neural replay, is tightly linked with the hippocampus. The hippocampus is a seahorse-shaped formation in the brain that belongs to the limbic system, and it is associated with the formation of new memories, as well as the emotions that memories spark. Current theories on the role of the hippocampi (there is one in each hemisphere of the brain), state that different regions of the hippocampus are responsible for the handling of different types of memories. For instance, spatial memory is believed to be handled in the rear region of the hippocampus.
As reported by Jesus Rodriguez, Dr. John O’Keefe is responsible for many contributions to our understanding of the hippocampus, including the hippocampal “place” cells. The place cells in the hippocampus are triggered by stimuli in a specific environment. As an example, experiments on rats showed that specific neurons would fire when the rats ran through certain portions of a track. Researchers continued to monitor the rats even when they were resting, and they found that the same patterns of neurons denoting a portion of the maze would fire, although they fired at an accelerated speed. The rats seemed to be replaying the memories of the maze in their minds.
In humans, recalling memories is an important part of the learning process, but when trying to enable AI to learn, it is difficult to recreate the phenomenon.
The DeepMind team set about trying to recreate the phenomenon of recall using reinforcement learning. Reinforcement learning algorithms work by getting feedback from their interactions with the environment around them, getting rewarded whenever they take actions that bring them closer to the desired goal. In this context, the reinforcement learning agent records events and then plays them back at later times, with the system being reinforced to improve how efficiently it ends up recalling past experiences.
DeepMind added the replaying of experiences to a reinforcement learning algorithm using a replay buffer that would playback memories/recorded experiences to the system at specific times. Some versions of the system had the experiences played back in random orders while other models had pre-selected playback orders. While the researchers experimented with the order of playback for the reinforcement agents, they also experimented with different methods of replaying the experiences themselves.
There are two primary methods that are used to provide reinforcement algorithms with recalled experiences. These methods are the imagination replay method and the movie replay method. The DeepMind paper uses an analogy to describe both of the strategies:
“Suppose you come home and, to your surprise and dismay, discover water pooling on your beautiful wooden floors. Stepping into the dining room, you find a broken vase. Then you hear a whimper, and you glance out the patio door to see your dog looking very guilty.”
As reported by Rodriguez, the imagination replay method doesn’t record the events in the order that they were experienced. Rather, a probable cause between the events is inferred. The events are inferred based on the agent's understanding of the world. Meanwhile, the movie replay method stores memories in the order in which the events occurred, and replays the sequence of stimuli – “spilled water, broken vase, dog”. The chronological ordering of events is preserved.
Research from the field of neuroscience implies that the movie replay method is integral to the creation of associations between concepts and the connection of neurons between events. Yet the imagination replay method could help the agent create new sequences when it reasons by analogy. For instance, the agent could reason that if a barrel is to oil as a vase is to water, a barrel could be spilled by a factory robot instead of a dog. Indeed, when DeepMind probed further into the possibilities of the imagination replay method, they found that their learning agent was able to create impressive, innovative sequences by taking previous experiences into account.
Most of the current progress being made in the area of reinforcement learning memory is being made with the movie strategy, although researchers have recently begun to make progress with the imagination strategy. Research into both methods of AI memory can not only enable better performance from reinforcement learning agents, but they can also help us gain new insight into how the human mind might function.
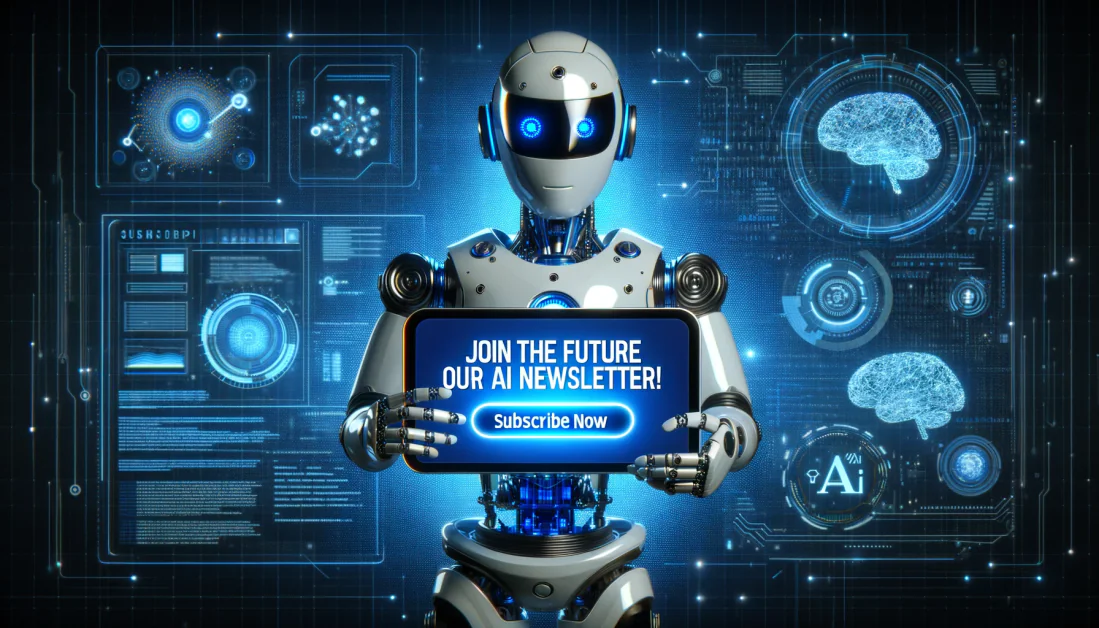
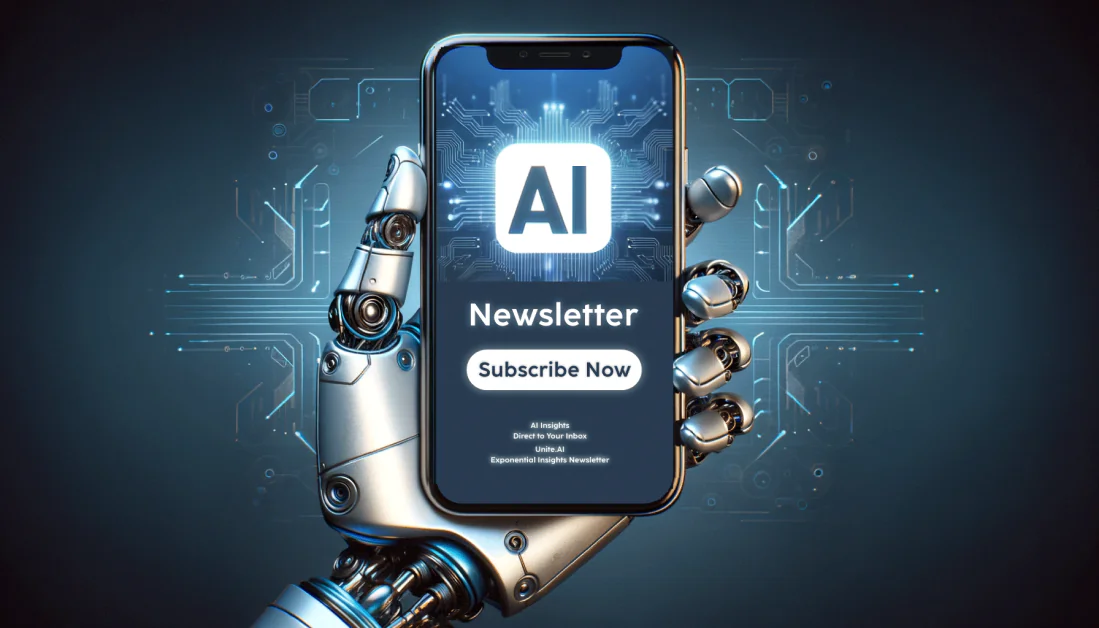