Artificial Intelligence
Using Deep Learning Autonomous Research Vessel ‘Mayflower’ Sets Sail In September 2020
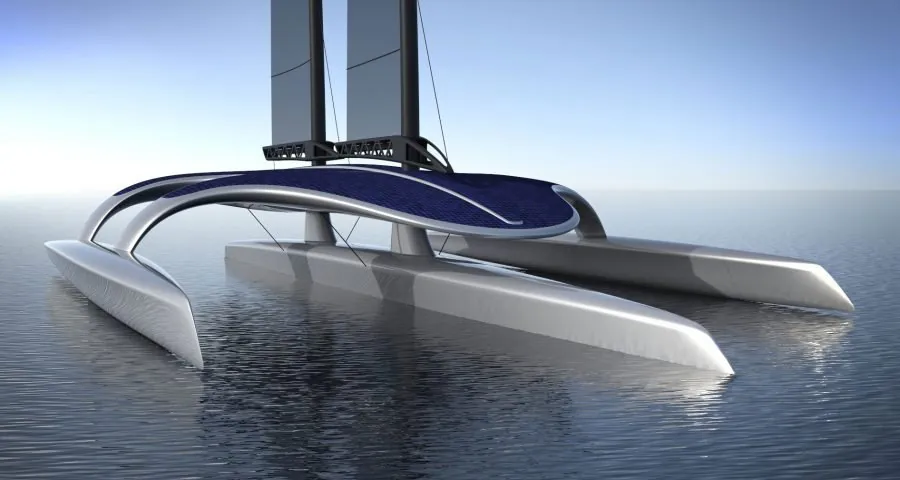
Next year marks the 400-year anniversary when the original ‘Mayflower ‘ ship set sail across the Atlantic Ocean. It is thought that it took 30 crew members to make sure that ship safely crosses the ocean, but as TechCrunch, CbrOnline, and other sources report, this version of the ‘Mayflower’ will be autonomous, powered by artificial intelligence, and will conduct extensive research.
The ship represents a result of global joint work that involves the University of Plymouth, marine research firm Promare, and technology giant IBM, which will along with technical support, supply its PowerAI vision technology backed by its Power Systems servers.
The new, autonomous Mayflower, as is reported, will be decked out with solar panels, as well as diesel and wind turbines to provide it with its propulsion power, as it attempts the 3,220-mile journey from Plymouth in England, to Plymouth in Massachusetts in the U.S. For its part, Promare is hoping that a successful crossing and mission, which would be “ the first for full-size seafaring vessels navigating the Atlantic on their own,” will make possible the use of other research-focused applications of autonomous seagoing ships.
The researchers and academics from the University of Plymouth will develop research pods that are supposed to tackle various experiments in areas such as “maritime cybersecurity, sea mammal monitoring and even addressing the challenges of ocean-borne microplastics.”
The IBM technology, based on the deep learning models that were developed in partnership with Promare and using radars, LIDAR sensors, automated identification systems, and optical cameras, is designed to “help with the avoidance of obstacles and hazards at sea.” On-board servers are then supposed to process the data to allow the ship “to determine the best course forward at an optimal speed.”
CBR adds that the “AI model is being trained in Plymouth Sound, a bay on the English Channel, where it is being fed real-world data and images to help it determine risks and the best avoidance measures.”
It is envisioned that the system will use “both local and remote processing, meaning devices on the ship will be able to operate without connection at the edge, and then check back in periodically with HQ when conditions allow for processing via nodes located at either shore.”
During its voyage, “IoT and edge devices will be constantly collecting data and storing it on-board till the ship encounters edge nodes that are located onshore. Once in range, the ship will transmit its data to these nodes, which will then upload the data to the IBM cloud.”
Brett Phaneuf, Founding Board Member of ProMare, wrote in a blog that: “Putting a research ship to sea can cost tens of thousands of dollars or pounds a day and is limited by how much time people can spend onboard – a prohibitive factor for many of today’s marine scientific missions. With this project, we are pioneering a cost-effective and flexible platform for gathering data that will help safeguard the health of the ocean and the industries it supports.”
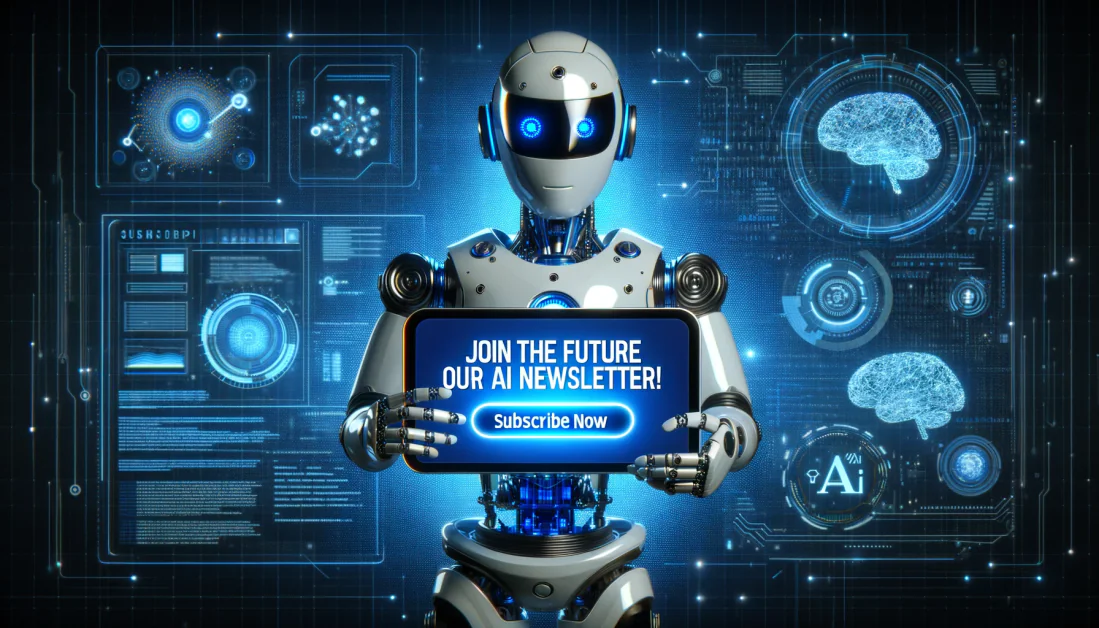
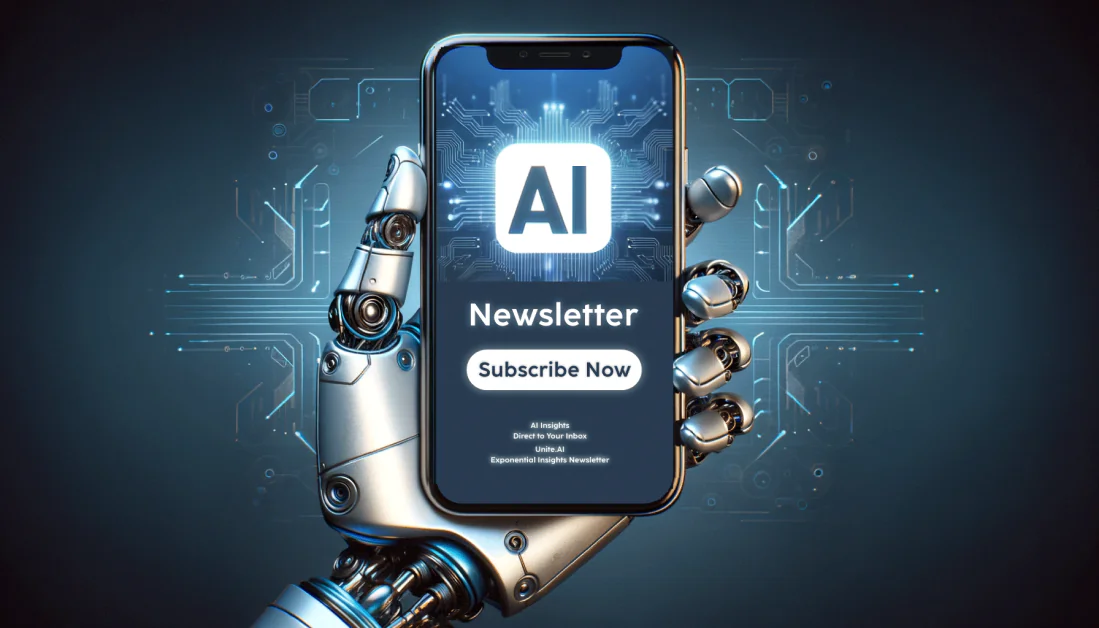