Interviews
Akilesh Bapu, Founder & CEO of DeepScribe – Interview Series
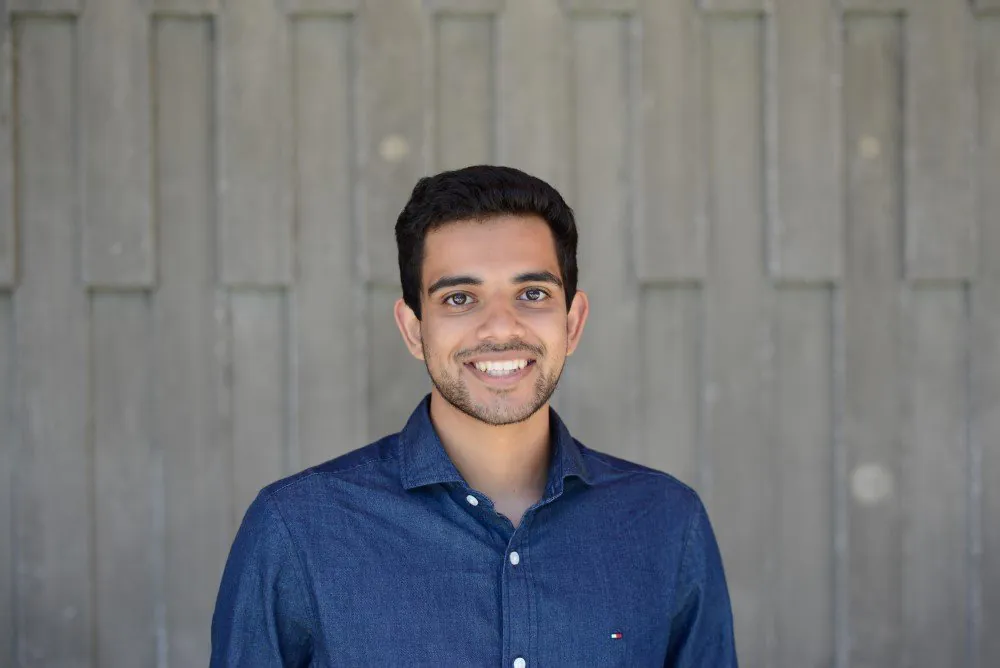
Akilesh Bapu is the Founder & CEO of DeepScribe, which uses natural language processing (NLP) and advanced deep learning to generate accurate, compliant, and secure notes of doctor-patient conversations.
What was it that introduced and attracted you to AI and natural language processing?
If I remember correctly, Jarvis from “Iron Man” was the first thing that really attracted me to the world of natural language processing and AI. Particularly, I found it fascinating how much faster a human was able to not only go through tasks but also go into an incredible level of depth into certain tasks and unveil certain information that they wouldn't have even known about if it weren't for this AI.
It was this concept of “AI by itself won't be as good as humans at most tasks but put a human and AI together and that combination will dominate.” Natural language processing is the most efficient way for this human/AI combination to happen.
From then on, I was obsessed with Siri, Google Now, Alexa, and the others. While they didn't work as seamlessly as Jarvis, I so badly wanted to make them work as Jarvis did. Particularly, what became apparent was, commands such as “Alexa do this,” “Alexa do that,” were pretty easy and accurate to do with the current state of technology. But when it comes to something like Jarvis, where it can actually learn and understand, filter, and pick up on important topics during another conversational exchange—that hadn't really been done before. This actually directly relates to one of my core motivations in founding DeepScribe. While we are solving the issue of documentation for physicians, we’re attempting a whole new wave of intelligence while doing it: ambient intelligence. AI that can dig through your day-to-day utterances, find useful information, and use that information to help you out.
You previously did some research using deep learning and NLP at UC Berkeley College of Engineering. What was your research on?
Back at the Berkeley AI Research Lab, I was working on a gene ontology annotator project where we were summarizing PubMed articles with specific output parameters.
The high-level overview: Take a task like the CNN news article summarization. In that task you're taking news articles and summarizing them into roughly a few sentences. In your favor you have data and the ability to train these models on over a million articles. However, the problem space is enormous since you have limited structure to the summaries. In addition, there is hardly any structure to the actual articles. While there have been quite a few improvements since 2.5 years ago when I was working on this project, this is still an unsolved problem.
In our research project, however, we were developing structured summaries of articles. A structured summary in this case is similar to a typical summary except we know the exact structure of the output summary. This is helpful since it dramatically reduces the output options for our machine learning model—the challenge was that there was not enough annotated training to run a data-hungry deep learning model and get usable results.
The core of the work I did on this project was to leverage the knowledge we have around the input data and develop an ensemble of shallow ML models to support it—a technique we invented called the 2-step annotator. The 2-step annotator benchmarked at nearly 20x the accuracy as the previous best (54 percent vs 3.6 percent).
While side by side, this project and DeepScribe may sound entirely different, they were highly similar in how they used the 2-step annotation method to vastly improve results on a limited dataset.
What was the inspiration behind launching DeepScribe?
It all started with my father, who was a medical oncologist. Before electronic health record systems took over health care, physicians would jot down things on paper and spend very little time on notes. However, once EHRs started becoming popular as part of the HITECH Act of 2009, I started noticing that my dad spent more and more time at the computer. He’d start coming home later. On the weekends, he’d be sitting on the couch dictating notes. Simple things like him picking me up from school or basketball practice became a thing of the past as he’d be spending most of his evening hours catching up on documentation.
As a nerdy kid growing up, I would try to find solutions for him by searching the web and having him try them out. Sadly, nothing worked well enough to save him from the long hours of documentation.
Fast forward several years to the summer of 2017—I’m a researcher working at the Berkeley AI Research Lab, working on projects in document summarization. One summer when I’m back at home, I notice that my dad is still spending copious amounts of time documenting. I ask, “What’s new in the world of documentation? Alexa is everywhere, Google Assistant is so good now. Tell me, what’s the latest in the medical space?” And his answer was, “Nothing has changed.” I thought that it was just him but when I went and surveyed several of his colleagues, it was the same issue: not what the latest is in cancer treatment or the novel problems their patients were having—it was documentation. “How can I get rid of documentation? How can I save time on documentation? It’s taking so much of my time.”
I also noticed several companies that had emerged to try to solve documentation. However, either they were too expensive (thousands of dollars per month) or they were too minimal in terms of technology. The physicians at that time had very few options. That was when the opportunity opened up that if we could create an artificially intelligent medical scribe, a technology that could follow physicians’ patient visits and summarize them, and offer it at a cost that could make it accessible for everyone, it could truly bring the joy of care back to medicine.
You were only 22 years old when you launched DeepScribe. Can you describe your journey as an entrepreneur?
My first exposure to entrepreneurship was back in high school. It started when a friend and I who happened to know some JavaScript basics met with the director of a center for children with learning disabilities. They told us how the simplest of tools could go a long way with dyslexic children. We ended up hacking together a dyslexia reader Chrome extension. It was really bare bones—it simply adjusted the font to meet the scientific guidelines for ease of reading by dyslexic people. While the concept was simple, we ended up getting over 5000 active users in a handful of months. I was blown away by how basic tech can have such a profound impact on people.
At Berkeley, I continued to delve into the world of entrepreneurship as much as possible, primarily with their wide array of classes. My favorites were:
- The Newton Lecture Series—people like Jessica Mah from InDinero or Diane Greene from VMWare who were Cal alums gave highly relatable talks about their time at Berkeley and how they started their own companies
- Challenge Lab—I actually met my co-founder Matt Ko through this class. We were placed in groups and went through a semester-long journey of creating a product and being mentored on what it takes during the early stages to get an idea going.
- Lean Launchpad—By far my favorite of the three; this was a grueling and rigorous process where we were guided by Steve Blank (acclaimed billionaire and the man behind the lean startup movement) to take an idea, validate it through 100 customer interviews, build a financial model, and more. This was the type of class where we pitched our “startup” only to get stopped on slide 1 or 2 and get grilled. If that wasn’t hard enough, we were also expected to interview 10 customers a week. Our idea at the time was to create a patent search that would give similar results to an expensive prior art search, which meant we were pitching to 10 enterprise customers a week. It was great because it taught us to think fast on our feet and be extra resourceful.
DeepScribe started when an investor group called The House Fund was writing checks for students who would turn down their summer internships and spend their summer building their company. We had just shut down Delphi (the patent search engine) and Matt and I had been constantly talking about medical documentation and everything fell in place since it was the perfect time to give it a shot.
With DeepScribe, we were lucky to have just come fresh out of Lean Launchpad since one of the most important factors in building a product for physicians was to iterate and refine the product around customer feedback. A historical issue with the medical industry has been that software has rarely had physicians in the design loop, therefore resulting in software that wasn’t optimized for the end user.
Since DeepScribe was happening at the same time as my final year at Berkeley, it was a heavy balancing act. I’d show up to class in a suit so I could be on time for a customer demo right after. I’d use all the EE facilities and professors not for anything to do with class but 100 percent for DeepScribe. My meetings with my research mentor even turned into DeepScribe brainstorming sessions.
Looking back, if I had to change one thing about my journey, it would’ve been to put college on hold so I could spend 150 percent of my time on DeepScribe.
Can you describe for a medical professional what the advantages of using DeepScribe are versus the more traditional method of voice dictation or even taking notes?
Using DeepScribe is meant to be very similar to using an actual human scribe. As you talk naturally to your patient, DeepScribe will listen in and pick up on the medically relevant speech that usually goes in your notes and puts it in there for you, using the same medical language that you yourself use. We like to think of it as a new AI-powered member of your medical staff that you can train as you’d like to help with documentation in your electronic health record system as you’d like. It’s very different from using voice dictation service as it eliminates the entire step of having to go back and document. While typical dictation services turn 10 minutes of documentation into 7-8 minutes, DeepScribe turns it into a few seconds. Our physicians report anywhere from 1.5 to 3 hours of time saved per day depending on how many patients they see.
DeepScribe is device-agnostic, operable from an iPhone, Apple Watch, browser (for telemedicine), or hardware device.
What are some of the speech recognition or NLP challenges that DeepScribe may encounter due to complex medical terminology?
Contrary to popular opinion, complex medical terminology is actually the easiest part for DeepScribe to pick up. The trickiest part for DeepScribe is to pick up on unique contextual statements a patient may give a physician. The more they stray from a typical conversation, the more we see the AI stumble. But as we collect more conversational data, we see it improve on this dramatically every day.
What are the other machine learning technologies that are used with DeepScribe?
The large umbrellas of speech recognition and NLP tend to cover most of the machine learning we’re doing at DeepScribe.
Can you name some of the hospitals, nonprofits, or academic institutions that are using DeepScribe?
DeepScribe started out through a pilot program with the UC Berkeley Health Center. Hartford Healthcare, Texas Medical Center, and Cedar Valley Medical Specialists are a handful of the larger systems DeepScribe is working with.
However, the larger percentage of DeepScribe users are 50 private practices from Alaska to Florida. Our most popular specialties are primary care, orthopedics, gastroenterology, cardiology, psychiatry, and oncology, but we do support a handful of other specialties.
DeepScribe has recently launched a program to assist with COVID-19. Could you walk us through this program?
COVID-19 has hit our doctors hard. Practices are only seeing 30-40 percent of their patient load, scribe staffing is being cut, and providers are being forced to rapidly switch all their patients on to telemedicine. All this ends up leading to more clerical work for providers—we at DeepScribe firmly believe that in order for this pandemic to come to a halt, physicians must devote 100 percent of their attention and time to taking care of their patients.
To help aid this cause, we are proud to launch a free telemedicine solution to health care professionals fighting this pandemic. Our telemedicine solution is fully integrated with our AI-powered medical scribe solution, eliminating the need for clinical documentation for encounters made on our platform.
We’re also offering our scribe service for free during the pandemic. This means that any physician can get access to a scribe for free to handle their documentation. Our hopes are that by doing this, physicians will be able to focus more of their attention on their patients and spend less time thinking about documentation, leading to a faster halting of the COVID-19 outbreak.
Thank you for the great interview, I really enjoyed learning about DeepScribe and your entrepreneurial journey. Anyone who wishes to learn more should visit DeepScribe.
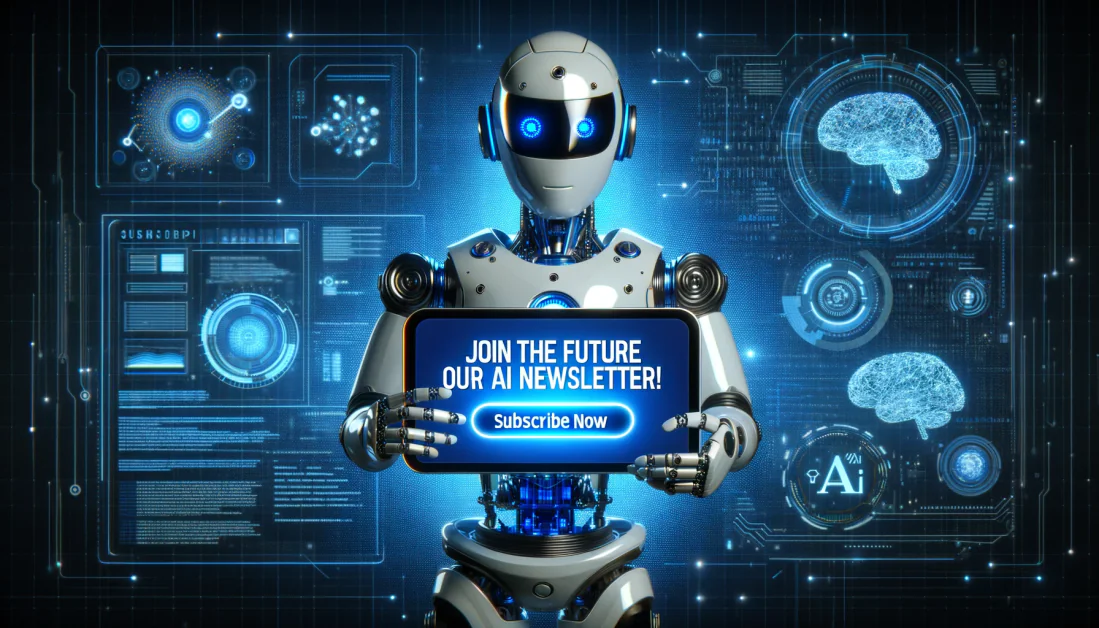
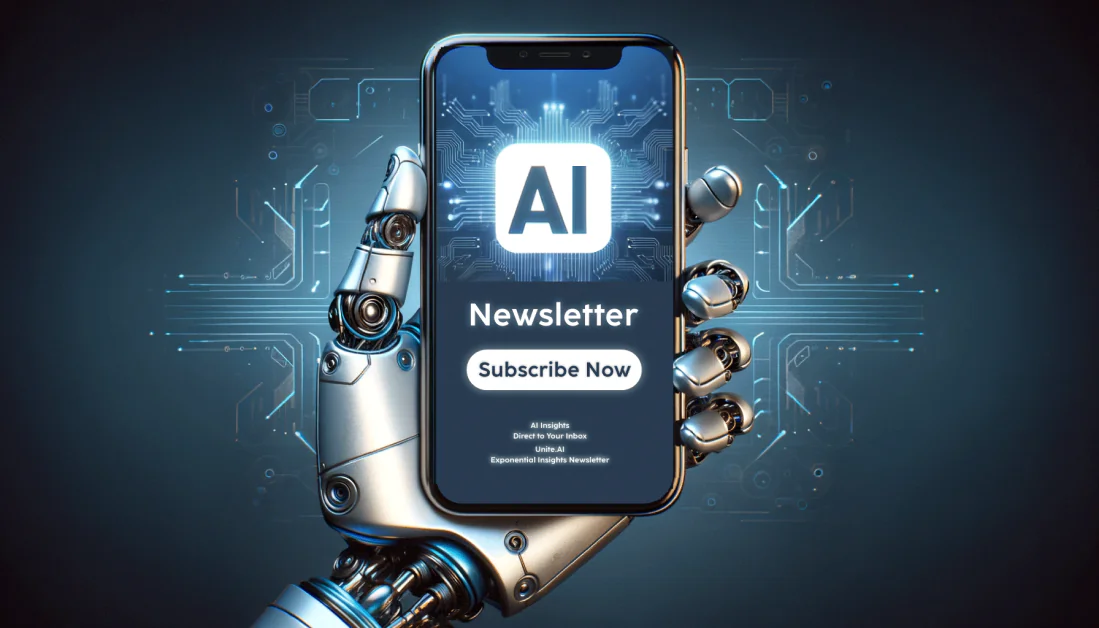