Artificial Intelligence
AI Could Help Keep Coffee Affordable and Accessible In The Face Of Climate Change
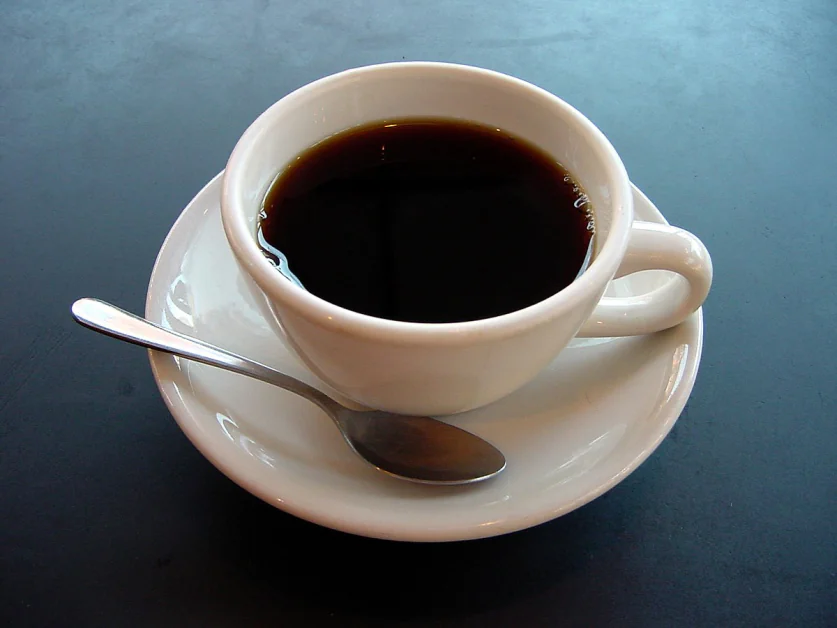
If you’re a lover of coffee, it will come as unpleasant news that the price of coffee could potentially spike in the near future. Climate change and deforestation are threatening some of the biggest coffee species in the world, but AI could potentially help keep coffee relatively affordable.
The combined forces of deforestation and climate change are threatening the production of many species of coffee, including the common Arabica species, which can be found in many of the most prolific blends and brews. Coffee farmers around the globe are having to deal with rising temperatures and the problems that are associated with them, such as periods of drought. One recent study published in the journals Global Change Biology and Science Advances found that there were substantial risks to many wild coffee species, with around 60% of 124 different wild coffee species being vulnerable to extinction.
As reported by Inside Climate News, Aaron P. Davis, one of the authors on the study and a senior research leader at England's Royal Botanic Gardens, explained that domesticated coffee is adapted from these wild species.
“We should be concerned about the loss of any species for lots of reasons,” explained Davis, “but for coffee specifically, I think we should remember that the cup in front of us originally came from a wild source.”
Domesticated coffee is composed of primarily two bean varieties: arabica and robusta. Wild plants are bred with these species to increase their quality, serving as a genetic library that allows scientists to create hardier plants. Biologists look to wild coffee varieties to find species with resistance to these threats, but as the climate continues to warm, this becomes more difficult.
Much as the wild strains of coffee are under pressure, cultivate coffee crops are also experiencing strain. Severe droughts and longer, more intense outbreaks of pests and disease are threatening cultivated crops. A fungal disease is taking advantage of the warmer conditions and higher humidity to proliferate amongst coffee crops, and the coffee borer beetle is possibly spreading faster thanks to climate change. Climate change also makes weather patterns more extreme, with more severe droughts and rainstorms. Either too much or too little rain can degrade coffee production. Further, it is estimated that around half of all wild coffee plants will disappear over the next 70 years.
Despite the recent problems climate change has brought to coffee farmers, demand for coffee is only likely to increase. The overall demand for food across the globe is expected to increase by around 60% by 2050, and small farmers produce most of the globe’s food supply, around 70%.
Amidst the growing threat of climate change, AI could help coffee farmers compensate for things like drought and pests. Researchers associated with the Financial and Agricultural Recommendation Models project, or FARM, intend to assist coffee farmers by providing them with techniques that can boost yields. Project FARM is initially going to be tested on coffee farmers throughout Kenya, where it will apply data science techniques to big datasets gathered from coffee farms. The FARM platform aims to bring automated farming systems and data science-backed techniques to small farms across Kenya, which should help boost yield. Project FARM is driven by the decreasing price of sensors and the accompanying availability of large datasets gathered by these sensors.
The AI-based farming methods can provide farmers with valuable insights that can help them optimize production. Machine learning algorithms can be used to predict weather patterns and take precautions against inclement weather, while computer vision systems can recognize crop damage and possible signs of spreading fungus or parasites. Gaining this valuable info can help farmers guard against these damaging forces. Farmers can even be alerted via SMS if a storm is expected the next day.
One company, Agrics, is able to use data gathered by sensors to predict risks that may impact individual farmers. Violanda de Man, Innovation Manager at Agrics East Africa, explained that the data can be used to give farmers location-specific services and products that can reduce farm risks and improve both the income and security of rural populations
As crops and farmers around the globe contend with the challenges of climate change, AI seems poised to play a major role in the struggle to contend with these challenges.
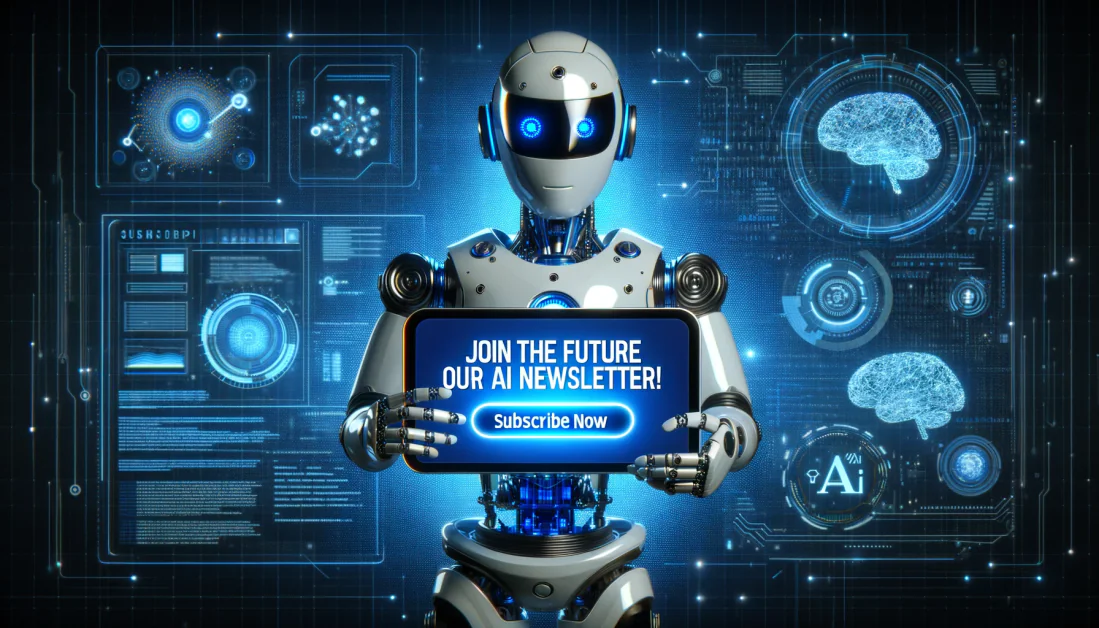
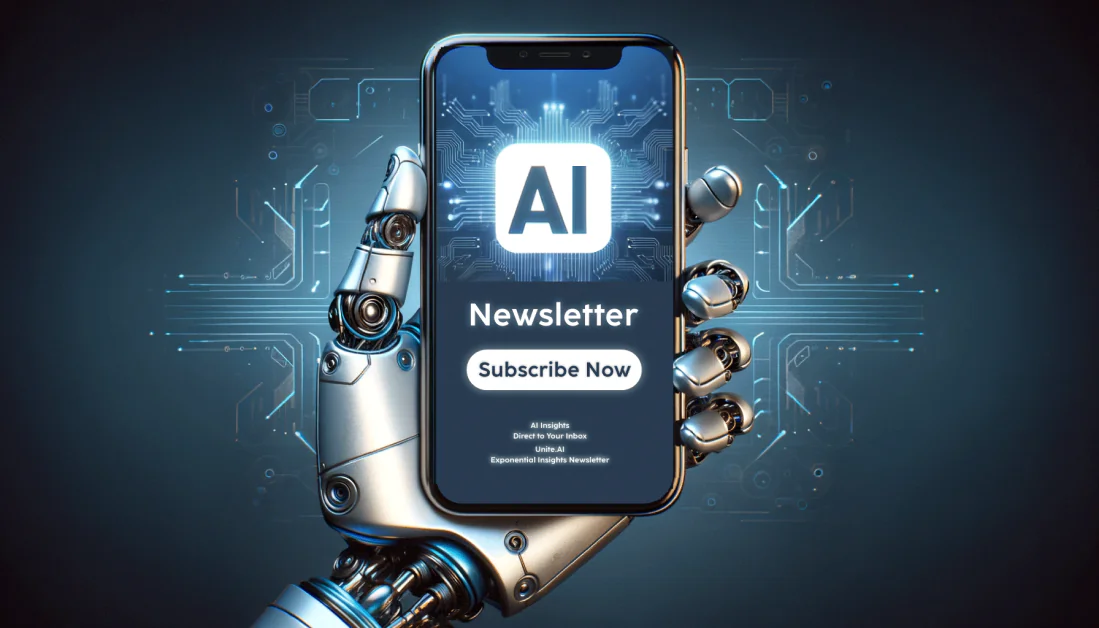