Healthcare
AI Can Improve Mental Health Care And Make Up For Lack Of Personnel
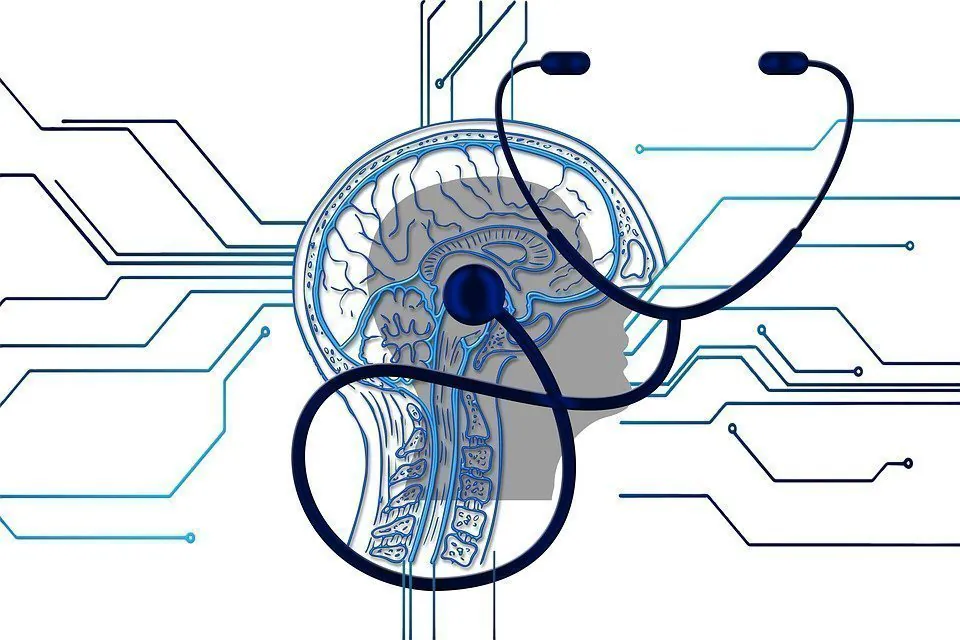
Artificial intelligence could help improve mental health care, making it more effective, along with addressing personnel issues that are poised to impact the mental health field over the course of the next decade. AI can potentially derive complex patterns from data, patterns which even trained psychiatrists and clinicians have difficulty seeing. Furthermore, as reported by Time, AI could help make up for critical shortages in mental health care workers, giving patients support outside of the time they spend with a clinician.
Over the course of the next five years, it is predicted that the US mental health system may come to lack around 15600 psychiatrists, according to a study published by the National Council for Behavioral Health. This means that the time and resources of clinicians and other mental health professionals will be spread thin. Even now, clinicians typically don't spend very much time with their patients, occasionally seeing a patient just once every few months.
Artificial Intelligence has recently made huge contributions to the medical field, improving accuracy of diagnosis, using computer vision to find obscure patterns in medical images, and engineering better treatment plans for patients. One of the ways mental healthcare differs from physical health care is that it requires a high level of perception and emotional intelligence in order to diagnose and treat patients, but AI could still have a beneficial effect on the field. The power of machine learning to analyze data and extract patterns, including those so confusing and subtle that humans find them hard to notice/interpret, can help mental health professionals treat and support their patients.
Advanced data analysis techniques can improve the diagnosis of certain mental health conditions like bipolar disease, and the quicker the diagnosis the faster patients can be put on the proper treatment course. Beyond this, artificial intelligence can help clinicians in other ways, such as letting doctors interact with their patients remotely or by automatically collecting and analyzing data that can be used to update treatment plans.
Currently, there are some applications that leverage artificial intelligence to support those with mental health conditions. Woebot, for instance, is a chatbot that employs principles derived from cognitive behavioral therapy to assist people in tracking their moods and managing thought patterns. The coming years could see much more sophisticated applications of artificial intelligence in the mental health field. As. Dr. Henry Nasrallah, a psychiatrist at the University of Cincinnati Medical Center explained to Time, there are methods that can be used to infer a patient’s mental health state, such as lack of speech affectation often correlating with depression, or with disjointed word use being linked to schizophrenia.
Clinicians often use speech patterns like this to diagnose patients, and AI algorithms can potentially pick up on patterns so subtle that people won’t discover them. Recently Peter Foltz, a research professor at the University of Colorado Boulder, and his collleagues created an app that has patients undergo various verbal exercises, collecting data about their tone and affections as they answer questions regarding their emotional state and relate stories. This data is then analyzed by an AI system that compares the clips against sound bites from a larger patient population in order to discover potential mental health issues. When tested on a population of 225 individuals across two different locations, the app performed at least as well as clinicians at detecting symptoms of mental health disorders or distress. Similar pattern recognition can also be done with written language, analyzing word choice and order of word use.
There are a few notable roadblocks to creating AI-based mental health diagnosis tools. One of the biggest issues is that clinicians and psychiatrists themselves often disagree about what criteria is needed to make a diagnosis, with illnesses like depression based on a variety of scales and criteria. Other problems like the questionable reliability of patient-reported data could also hamper efforts to design diagnostic AI tools. Even AI and mental health researchers themselves emphasize that their tools aren’t meant to replace human psychiatrists and acknowledge their limitations. However, as data collection gets better and models become more sophisticated, the reliability of mental health diagnostic AIs may increase. Finally, by automating many of the time-consuming processes that clinicians must deal with, AIs can allow mental healthcare providers to spend more time with patients, a worthy goal all on its own.
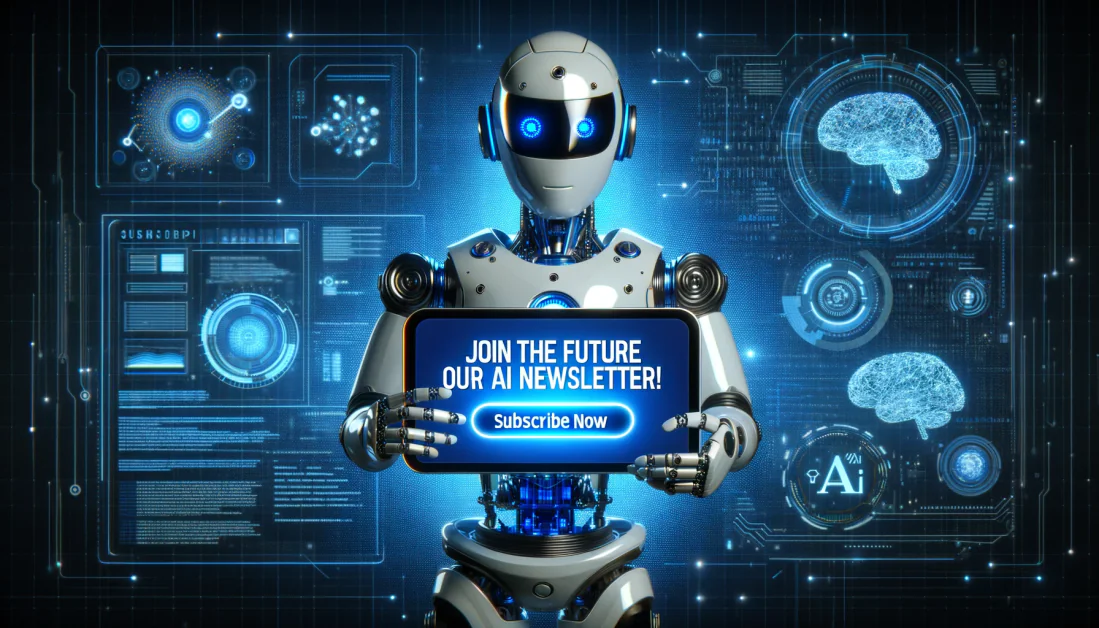
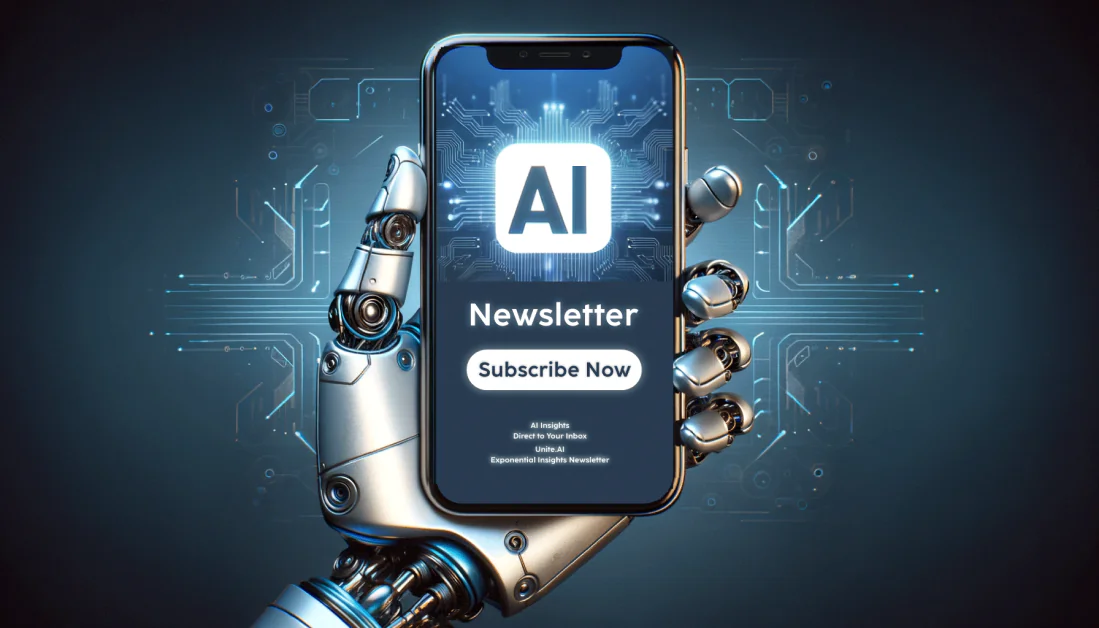