Interviews
Yasser Khan, CEO of ONE Tech – Interview Series
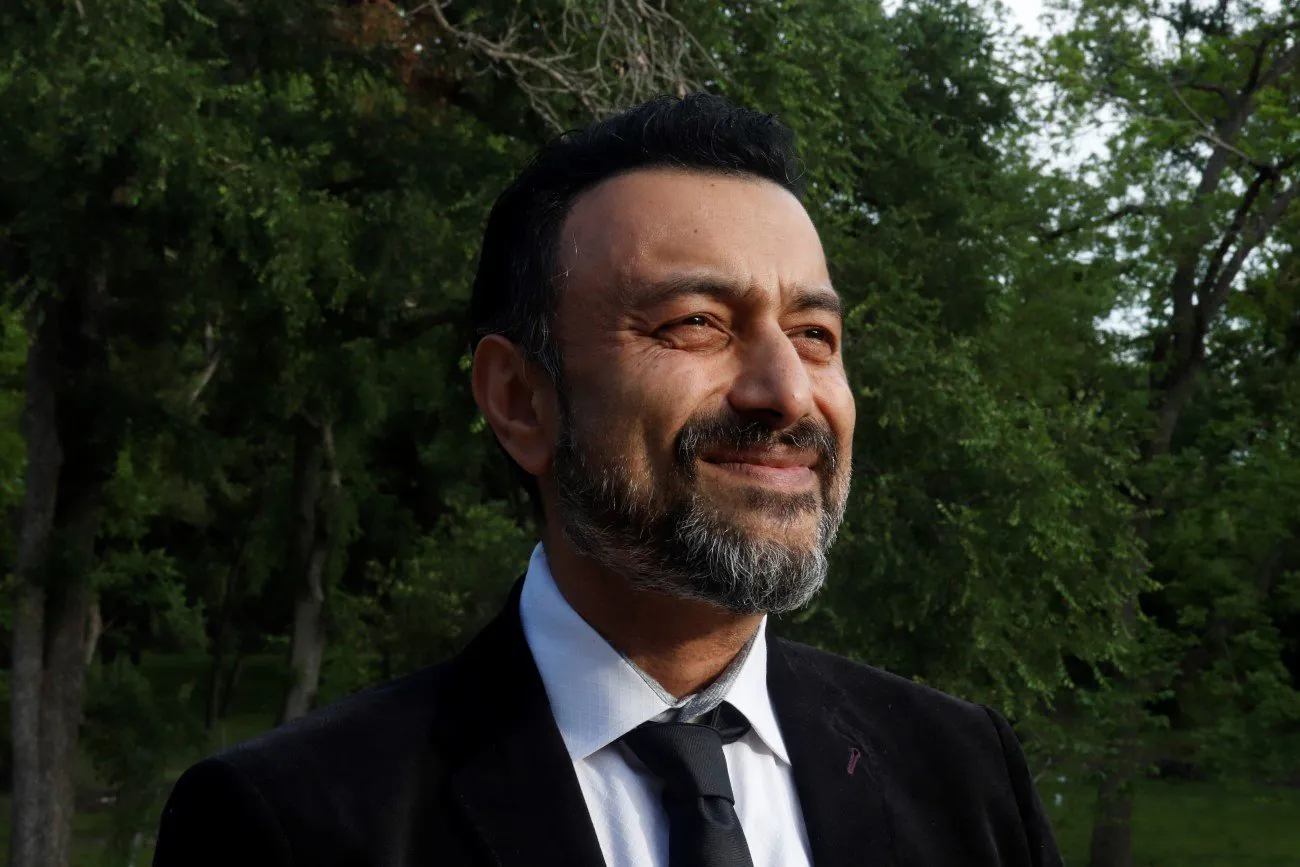
Yasser Khan, is the CEO of ONE Tech an AI-driven technology company that designs, develops and deploys next-generation IoT solutions for OEMs, network operators and enterprises.
What initially attracted you to Artificial Intelligence?
A few years ago, we deployed an Industrial Internet of Things (IIoT) solution that connected many assets across a wide geographic location. The amount of data that was generated was immense. We aggregated data from PLCs at sampling rates of 50 milliseconds and external sensor values a few times a second. Over the course of a single minute, we had thousands of datapoints being generated for each asset we were connecting to. We knew that the standard method of transmitting this data to a server and having a person evaluate the data was not realistic, nor beneficial for the business. So we set out to create a product that would process the data and generate consumable outputs, greatly reducing the amount of oversight that an organization needs in order to reap the benefits of a digital transformation deployment—heavily focused on asset performance management and predictive maintenance.
Can you discuss what ONE Tech’s MicroAI solution is?
MicroAI™ is a Machine Learning platform that provides a greater level of insight into asset (device or machine) performance, utilization and overall behavior. This benefit ranges from manufacturing plant managers who are looking for ways to improve overall equipment effectiveness to hardware OEMs who want to better understand how their devices are preforming out in the field. We accomplish this by deploying a small (as small as 70kb) packet onto the microcontroller (MCU) or microprocessor (MPU) of the asset. A key differentiator is that MicroAI’s process of training and forming a model is unique. We train the model directly on the asset itself. Not only does this allow for data to stay local, which reduces cost and time of deployment, but it also increases the accuracy and precision of the AI output. MicroAI has three primary layers:
- Data ingestion – MicroAI is agnostic to data input. We can consume any sensor value and the MicroAI Platform allows for feature engineering and weighting of the inputs within this first layer.
- Training – We train directly within the local environment. The training duration can be set by the user depending on what a normal cycle of the asset is. Typically, we like to capture 25-45 normal cycles, but this is heavily based on variation/volatility of each cycle captured.
- Output – Notifications and Alerts are generated by MicroAI based upon the severity of the anomaly that is detected. These thresholds can be adjusted by the user. Other outputs generated by MicroAI include Predicted Days to Next Maintenance (for optimizing service schedules), Health Score, and Asset Life Remaining. These outputs can be sent to existing IT systems that the clients have in place (Product Lifecycle Management tools, Support/Ticketing Management, Maintenance, etc.)
Can you discuss some of the machine learning technologies behind MicroAI?
MicroAI features a Multi-Dimensional Behavioral Analysis packed within a recursive algorithm. Each input that is fed into the AI engine impacts the thresholds (upper and lower bounds) that are set by the AI model. We do this by providing a one step ahead prediction. For example, if one input is RPMs and RPMs increase, the upper-bound threshold of bearing temperature may go up slightly due to the faster machine movement. This allows the model to continue to evolve and learn.
MicroAI is not reliant on accessing the cloud, what are the advantages of this?
We have a unique approach to forming models directly on the endpoint (where data is generated). This brings data privacy and security to deployments because data does not need to leave the local environment. This is especially important for deployments where data privacy is mandatory. Furthermore, the process of training data in a cloud is time consuming. This time consumption of how others are approaching this space is caused by the need of aggregating historical data, transmitting data to a cloud, forming a model, and eventually pushing that model down to the end assets. MicroAI can train and live 100% in the local environment.
One of the features of the MicroAI technology is its accelerated anomaly detection, could you elaborate on this functionality?
Due to our approach of behavioral analysis, we can deploy MicroAI and instantly start learning the behavior of the asset. We can start to see patterns within the behavior. Again, this is without the need of loading any historical data. Once we capture sufficient cycles of the asset, we can then begin to generate accurate output from the AI model. This is groundbreaking for the space. What used to take weeks or months to form an accurate model can happen within a matter of hours, and sometimes minutes.
What’s the difference between MicroAI™ Helio and MicroAI™ Atom?
MicroAI™ Helio Server:
Our Helio Server environment can be deployed in a local server (most common), or in a cloud instance. Helio provides the following functionality: (Workflow management, data analysis and management, and data visualization).
Workflows for managing assets – A hierarchy of where they are deployed and how they are used. (e.g., setup of all customer facilities globally, specific facilities and sections within each facility, individual stations, down to each asset in each station). Furthermore, the assets may be setup to perform different jobs with different cycle rates; this can be configured within these workflows. In addition is the ability for ticket/workorder management, which is also a part of the Helio Server environment.
Data analysis and management – Within this section of Helio, a user can run further analytics on the AI output, along with any raw data snapshots (i.e., Max, Min, and average data values on an hourly basis or data signatures that triggered an alert or an alarm). These can be queries that are configured in the Helio Analytics designer or more advanced analytics brought in from tools such as R, a programming language. The data management layer is where a user can utilize the API management gateway for 3rd-party connections that are consuming and/or sending data in coordination with the Helio environment.
Data Visualization – Helio provides templates for various industry specific reporting, which allows for users to consumer Enterprise Asset Management and Asset Performance Management views of their connected assets from both the Helio desktop and mobile applications.
MicroAI Atom:
MicroAI Atom is a Machine Learning platform designed for embedding into MCU environments. This includes training of the multi-dimensional behavior analysis recursive algorithm directly in local MCU architecture—not in a cloud and then pushed down to the MCU. This allows for accelerating the build and deployment of ML models through the auto-generation of the upper and lower thresholds based on multivariant model that is formed directly on the endpoint. We have created MicroAI to be a more efficient way of consuming and processing signal data to train models than other traditional methods. This not only brings a higher level of accuracy to the model that is formed but utilizes less resources on the host hardware (i.e., lower memory and CPU usage), which allows us to run in environments such as an MCU.
We have one other core offering called MicroAI™ Network.
MicroAI™ Network – Allows for a network of Atoms to be consolidated and mashed with external data sources for creating multiple models directly at the edge. This allows for horizontal and vertical analysis to be run on the various assets that are running Atom. MicroAI Network allows for an even deeper level of understanding to how a device/asset is performing in relation to similar assets that are deployed. Again, due to our unique approach to forming models directly at the edge, the machine learning models consume very little memory and CPU of the host hardware.
ONE Tech also offers IoT security consulting. What's the process for threat modeling and IoT penetration testing?
Due to our ability to understand how assets behave, we can consume data related to the internals of a connected device (e.g., CPU, Memory Usage, data pack size/frequency). IoT devices have, for the most part, a regular pattern of operation—how often it transmits data, where it sends the data, and the size of that data packet. We apply MicroAI to consume these internal data parameters to form a baseline of what is normal for that connected device. If an abnormal action occurs on the device, we can trigger a response. This can range from rebooting a device or opening a ticket within a workorder management tool, to completely cutting network traffic to a device. Our security team has developed testing hacks and we have successfully detected various Zero-Day attack attempts by using MicroAI in this capacity.
Is there anything else that you would like to share about ONE Tech, Inc?
Below is a diagram of how MicroAI Atom functions. Starting with acquiring raw data, training and processing in the local environment, inferencing the data and providing output.
Below is a diagram of how MicroAI Network functions. Many MicroAI Atoms feed into MicroAI Network. Along with the Atom data, additional data sources can be merged into the model for a more granular understanding of how the asset is performing. Furthermore, within MicroAI Network multiple models are formed allowing stakeholders to run horizontal analysis on how assets are performing in different regions, between customers, before and after updates, etc.
Thank you for the interview and your detailed responses, readers who wish to learn more should visit ONE Tech.
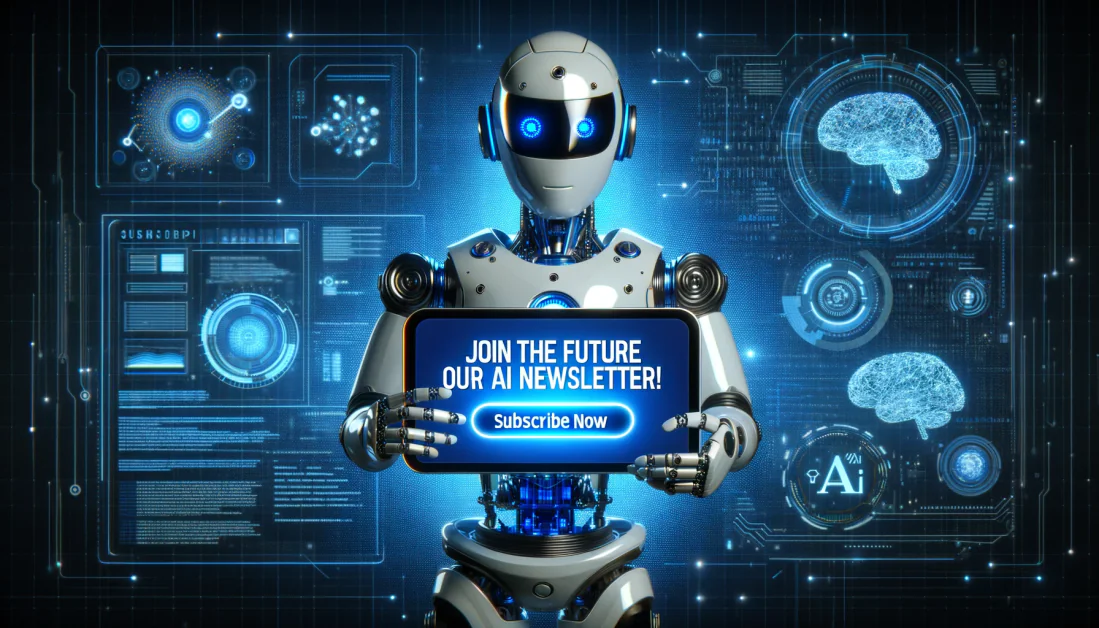
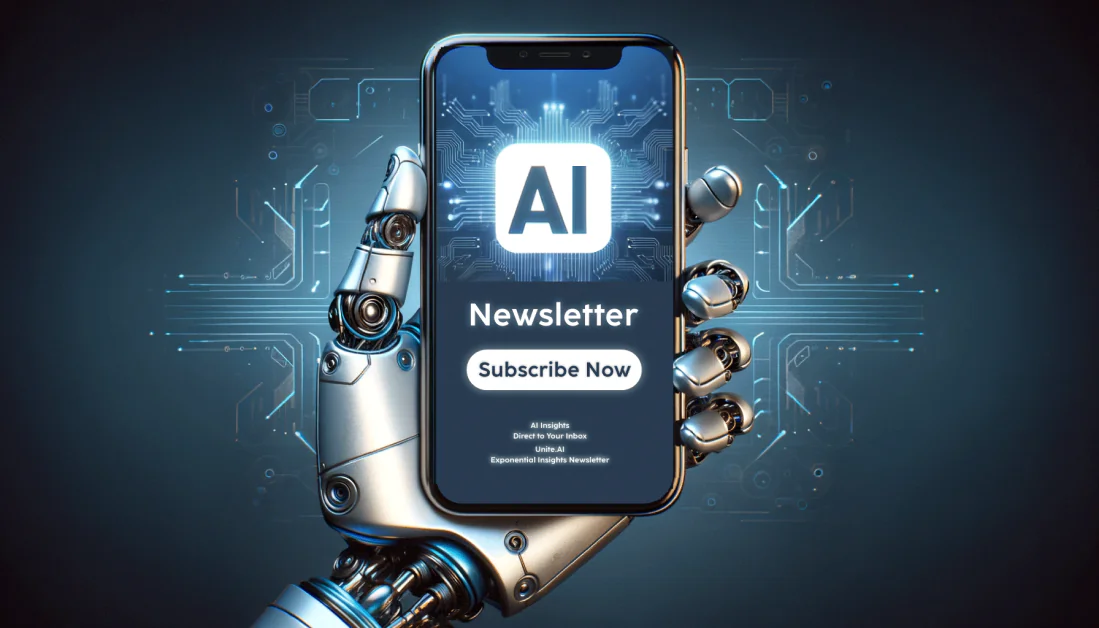