Interviews
Yaron Singer, CEO at Robust Intelligence & Professor of Computer Science at Harvard University – Interview Series
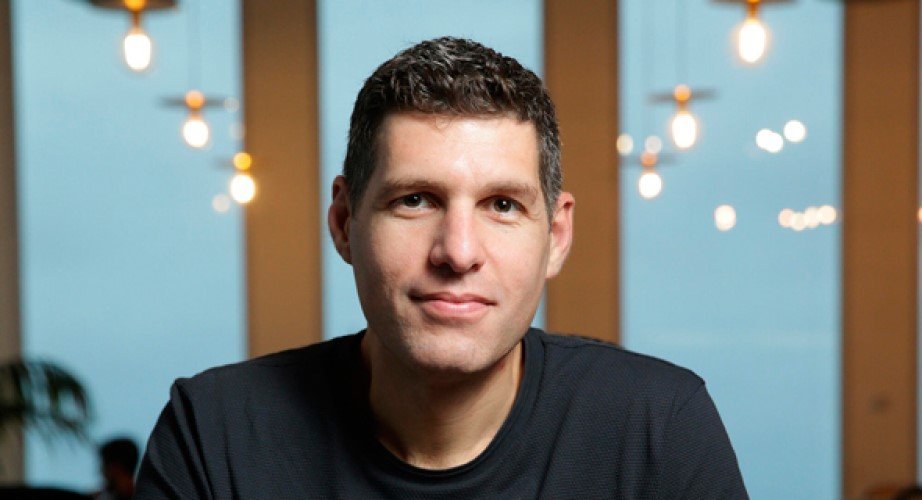
Yaron Singer is the CEO of Robust Intelligence and Professor of Computer Science and Applied Math at Harvard. Yaron is known for breakthrough results in machine learning, algorithms, and optimization. Previously, Yaron worked at Google Research and obtained his PhD from UC Berkeley.
What initially attracted you to the field of computer science and machine learning?
My journey began with math, which led me to computer science, which set me on the path to machine learning. Math initially drew my interest because its axiomatic system gave me the ability to create new worlds. With computer science, I learned about existential proofs, but also the algorithms behind them. From a creative perspective, computer science is the drawing of boundaries between what we can and cannot do.
My interest in machine learning has always been rooted in an interest in real data, almost the physical aspect of it. Taking things from the real world and modeling them to make something meaningful. We could literally engineer a better world through meaningful modeling. So math gave me a foundation to prove things, computer science helps me see what can and cannot be done, and machine learning enables me to model these concepts in the world.
Until recently you were a Professor of Computer Science and Applied Mathematics at Harvard University, what were some of your key takeaways from this experience?
My biggest takeaway from being a faculty member at Harvard is that it develops one’s appetite for doing big things. Harvard traditionally has a small faculty, and the expectation from tenure track faculty is to tackle big problems and create new fields. You have to be audacious. This ends up being great preparation for launching a category-creating startup defining a new space. I don’t necessarily recommend going through the Harvard tenure track first—but if you survive that, building a startup is easier.
Could you describe your ‘aha’ moment when you realized that sophisticated AI systems are vulnerable to bad data, with some potentially far-reaching implications?
When I was a graduate student at UC Berkeley, I took some time off to do a startup that built machine learning models for marketing in social networks. This was back in 2010. We had massive amounts of data from social media, and we coded all models from scratch. The financial implications for retailers were quite significant so we followed the models’ performance closely. Since we used data from social media, there were many errors in the input, as well as drift. We saw that very small errors resulted in big changes in the model output and could result in bad financial outcomes for retailers using the product.
When I transitioned into working on Google+ (for those of us who remember), I saw the exact same effects. More dramatically, in systems like AdWords that made predictions about the likelihood of people clicking on an advertisement for keywords, we noticed that small errors in input to the model lead to very poor predictions. When you witness this problem at Google scale, you realize the problem is universal.
These experiences heavily shaped my research focus, and I spent my time at Harvard investigating why AI models make mistakes and, importantly, how to design algorithms that can prevent models from making mistakes. This, of course, led to more ‘aha’ moments and, eventually, to the creation of Robust Intelligence.
Could you share the genesis story behind Robust Intelligence?
Robust Intelligence started with research on what was initially a theoretical problem: what are the guarantees we can have for decisions made using AI models. Kojin was a student at Harvard, and we worked together, initially writing research papers. So, it starts with writing papers that outline what is fundamentally possible and impossible, theoretically. These results later continued to a program for designing algorithms and models that are robust to AI failures. We then build systems that can run these algorithms in practice. After that, starting a company where organizations could use a system like this was a natural next step.
Many of the issues that Robust Intelligence tackles are silent errors, what are these and what makes them so dangerous?
Before giving a technical definition of silent errors, it’s worth taking a step back and understanding why we should care about AI making errors in the first place. The reason we care about AI models making mistakes is the consequences of these mistakes. Our world is using AI to automate critical decisions: who gets a business loan and at what interest rate, who gets health insurance coverage and at what rate, which neighborhoods should police patrol, who is most likely to be a top candidate for a job, how should we organize airport security, and so on. The fact that AI models are extremely error-prone means that in automating these critical decisions we inherit a great deal of risk. At Robust Intelligence we call this “AI Risk” and our mission in the company is to eliminate AI Risk.
Silent errors are AI models errors where the AI model receives input and produces a prediction or decision that is wrong or biased as an output. So, on the surface, everything to the system looks OK, in that the AI model is doing what it’s supposed to do from a functional perspective. But the prediction or decision is erroneous. These errors are silent because the system doesn’t know that there’s an error. This can be far worse than the case in which an AI model is not producing an output, because it can take a long time for organizations to realize that their AI system is faulty. Then, AI risk becomes AI failures which can have dire consequences.
Robust Intelligence has essentially designed an AI Firewall, an idea that was previously considered impossible. Why is this such a technical challenge?
One reason the AI Firewall is such a challenge is because it goes against the paradigm the ML community had. The ML community’s previous paradigm has been that in order to eradicate errors, one needs to feed more data, including bad data to models. By doing that, the models will train themselves and learn how to self-correct the mistakes. The problem with that approach is that it causes the accuracy of the model to drop dramatically. The best-known results for images, for example, cause AI model accuracy to drop from 98.5% to about 37%.
The AI Firewall offers a different solution. We decouple the problem of identifying an error from the role of creating a prediction, meaning the firewall can focus on one specific task: determine whether a datapoint will produce an erroneous prediction.
This was a challenge in itself due to the difficulty of giving a prediction on a single data point. There are a lot of reasons why models make errors, so building a technology that can predict these errors was not an easy task. We are very fortunate to have the engineers we do.
How can the system help to prevent AI bias?
Model bias comes from a discrepancy between the data the model was trained on and the data it is using to make predictions. Going back to AI risk, bias is a major issue attributed to silent errors. For example, this is often an issue with underrepresented populations. A model may have bias because it has seen less data from that population, which will dramatically affect the performance of that model and the accuracy of its predictions. The AI Firewall can alert organizations to these data discrepancies and help the model make correct decisions.
What are some of the other risks to organizations that an AI firewall helps prevent?
Any company using AI to automate decisions, especially critical decisions, automatically introduces risk. Bad data could be as minor as inputting a zero instead of a one and still result in significant consequences. Whether the risk is incorrect medical predictions or false predictions about lending, the AI Firewall helps organizations to prevent risk altogether.
Is there anything else that you would like to share about Robust Intelligence?
Robust Intelligence is growing rapidly and we’re getting a lot of great candidates applying for positions. But something I really want to emphasize for people who are considering applying is that the most important quality we seek in candidates is their passion for the mission. We get to meet a lot of candidates who are strong technically, so it really comes down to understanding whether they are truly passionate about eliminating AI risk to make the world a safer and better place.
In the world we are going towards, many decisions that are currently being made by humans will be automated. Whether we like it or not, that's a fact. Given that, all of us at Robust Intelligence want automated decisions to be done responsibly. So, anyone who is excited about making an impact, who understands the way that this can affect people’s lives, is a candidate we are looking for to join Robust Intelligence. We are looking for that passion. We are looking for the people who will create this technology that the whole world will use.
Thank you for the great interview, I enjoyed learning about your views on preventing AI bias and on the need for an AI firewall, readers who wish to learn more should visit Robust Intelligence.
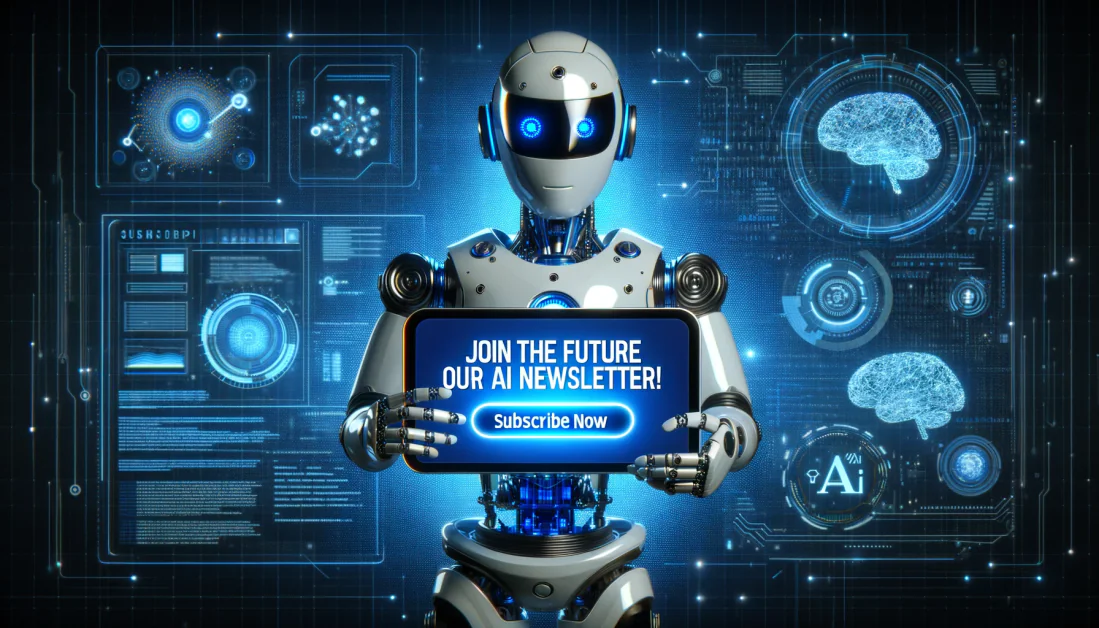
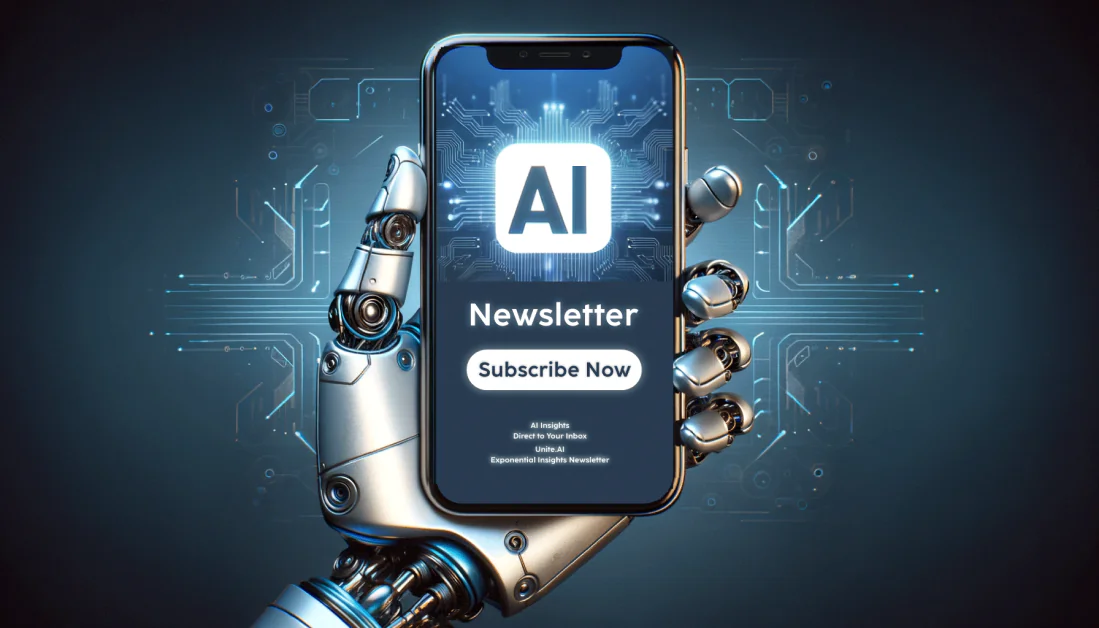