Interviews
Wilson Pang, Co-Author of Real World AI – Interview Series
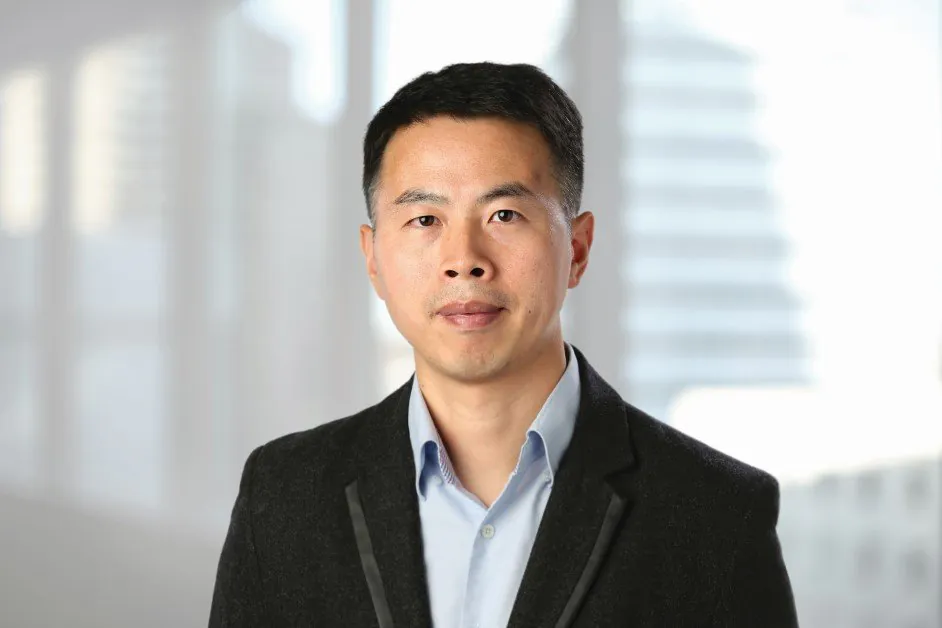
Wilson Pang joined Appen in November 2018 as CTO and is responsible for the company’s products and technology. Wilson has over nineteen years’ experience in software engineering and data science. Prior to joining Appen, Wilson was chief data officer of Ctrip in China, the second-largest online travel agency company in the world, where he led data engineers, analysts, data product managers, and scientists to improve user experience and increase operational efficiency that grew the business. Before that, he was senior director of engineering at eBay in California and provided leadership in various domains, including data service and solutions, search science, marketing technology, and billing systems. He worked as an architect at IBM prior to eBay, building technology solutions for various clients. Wilson obtained his master’s and bachelor’s degrees in electrical engineering from Zhejiang University in China.
We discuss his new book: The Real World of AI: A Practical Guide for Responsible Machine Learning
You describe how when you led eBay’s search science teams, one of your first lessons with machine learning was understanding the importance of knowing what metrics to measure. The example given was how the metric “purchases per session” failed to account for the monetary value of an item. How can companies best understand what metrics need measuring in order to avoid similar issues?
Start with the goals your team attributes to the AI model – in our case, we wanted to drive more revenue with machine learning. When you attach metrics to the goals, think about what mechanics those metrics will produce, once you release the model and people start interacting with it, but also make note of your assumptions. In our case, we assumed the model would optimize for revenue, but the number of purchases per session did not translate to that, because the model was optimizing for high number of low-ticket value sales, and at the end of the day we weren’t making more money. Once we realized that, we were able to change the metrics and point the model in the right direction. So determining the granular metrics, as well as noting assumptions are critical to the success of a project.
What did you personally learn from researching and writing this book?
We have a lot of different problems that can be solved by AI from different companies and different industries. The use cases can be very different, the AI solution might be different, the data to train that AI solution might be different. However, regardless all those differences, the mistakes people made during their AI journey are quite similar. Those mistakes happened again and again in all kinds of companies from all kinds of industries.
We shared some common best practices when implementing AI projects with hopes to help more people and companies avoid those mistakes and gain them the confidence to deploy responsible AI.
What are some of the most important lessons that you hope people will take from reading this?
We believe fiercely that thoughtful, responsible, and ethical uses of machine learning technology can make the world a more just, fair, and inclusive place. Machine learning technology promises to reshape everything throughout the business world, but it doesn’t have to be hard. There are tried and tested methods and processes teams can follow and get the confidence to deploy to production.
Another key lesson is that line-of-business owners (like product managers) and team members on the more technical side (like engineers and data scientists) need to speak a common language. To successfully deploy AI, leaders must bridge the gap between teams, providing business specialists and the C-level enough context to converse efficiently with technical implementers.
A lot of people first think about code when they think of AI. One of the key lessons in the book is that data is critical to the success of an AI model. There is a lot that goes with data from collecting to labelling to storage and every step will influence the success of the model. The most successful AI deployments are the ones who put high emphasis on data and strive to continuously improve this aspect of their ML model.
All real-world AI requires is a cross-functional team and an innovative spirit.
Discussed is determining when an AI model’s accuracy is high enough to support using AI. What’s the easiest way to assess the type of accuracy that is needed?
It depends on your use cases and risk tolerance. Teams developing AI should always have a testing phase where they determine accuracy levels and acceptable thresholds for their organizations and stakeholders. For life-or-death use cases – where there is potential harm if the AI goes wrong, like in the case of sentencing software, self-driving cars, medical use cases, the bar is very, very high – and teams must put in place contingencies in case the models are wrong. For more fault tolerant use cases, where there’s a lot of subjectivity at play – like content, search or ads relevance, teams can rely on user feedback to continue to adjust their models even while in production. Of course, there are some high-risk use cases here, as well, where illegal or immoral material might be show to users, so safeguards and feedback mechanisms must be in place here, too.
Can you define the importance of defining success for a project up-front?
It’s equally as important to start with a business problem as it is to define success up-front as the two go hand-in-hand. Following the example in the book about the automotive dealer using AI to label images, they did not determine what success looked like because they had not defined a business problem to solve. Success to them could have been a number of different things which makes it difficult to solve for a problem, even for teams of people, let alone a machine learning model with a fixed scope. If they had set out to label all vehicle with dents to create a list of vehicles that needed repair and defined success as accurately labelling 80% of all vehicle dents in the used car inventory, then when they would have accurately labelled 85%, the team would have called it a success. But if that success is not tied to the business problem, and to direct business impact, it’s hard to evaluate the project outside the focused definition of labeling accuracy in this example. Here, the business problem was more complex, and labeling dents is just a component of it. In their case, they could have been better of by defining success as saving time/money on the claims process or optimizing the repairs process by X% and then translate the labeling impact into real business outcomes.
How important is ensuring that training data examples cover all the use cases that will happen in the production deployment?
It is extremely important that the model be trained on all use cases to avoid bias. But it’s also important to note that, while it’s impossible to cover absolutely all the use cases in production, teams building AI need to understand their production data, as well as their training data so that they train the AI for what it will encounter in production. Accessing training data that comes from large diverse groups with various use cases will be critical to model success. For example, a model that is trained to recognize people’s pet in an uploaded image needs to be trained on all types of pets; dogs, cats, birds, small mammals, lizards, etc. If the model is only train on dogs, cats, and birds, then when someone uploads an image with their guinea pig, the model will not be able to identify it. While this is a very simple example, it shows how training on as many likely use cases as possible is critical to the success of a model.
Discussed in the book is the need to develop good data hygiene habits from the top down, what are some common first steps to nurture this habit?
Good data hygiene habits will increase the usability of internal data and prime it for ML use cases. The entire company has to become good at organizing and keeping track of its datasets. One sure way of achieving this is making it a business requirement and tracking implementation so that there are very few reports that end up being custom jobs, and teams work more and more with data pipelines funneled to a central repository, with a clear ontology. Another good practice is keeping a record of when and where the data was collected and what happened it to before it was placed in the database, as well as establishing processes for cleaning out unused or stale data periodically.
Thank you for the great interview, for readers who are interested in learning more, I recommend that they read the book The Real World of AI: A Practical Guide for Responsible Machine Learning.
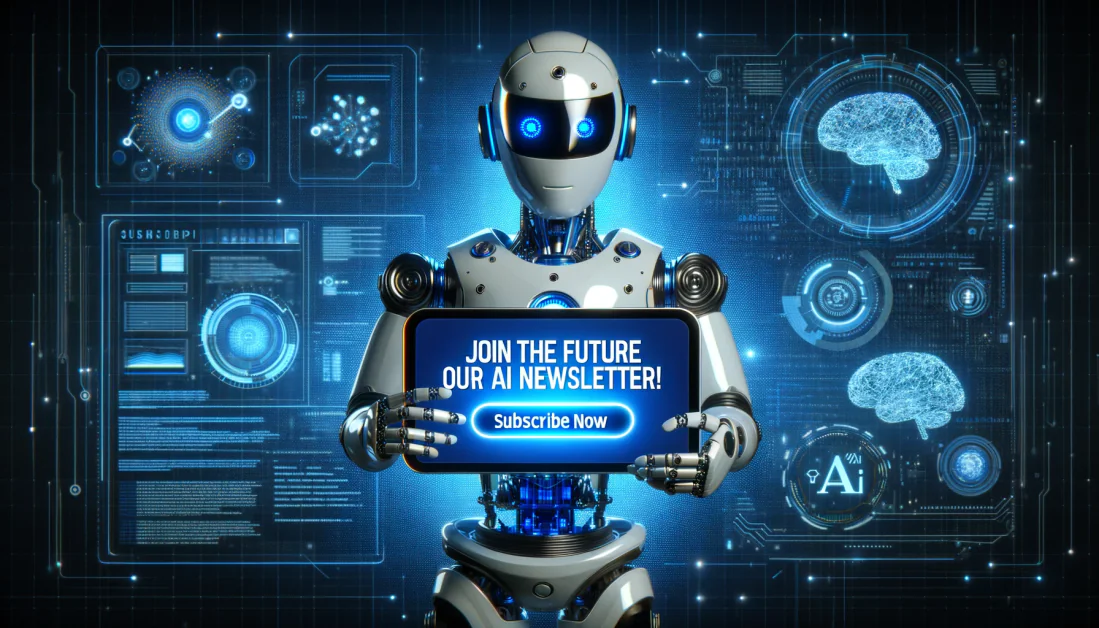
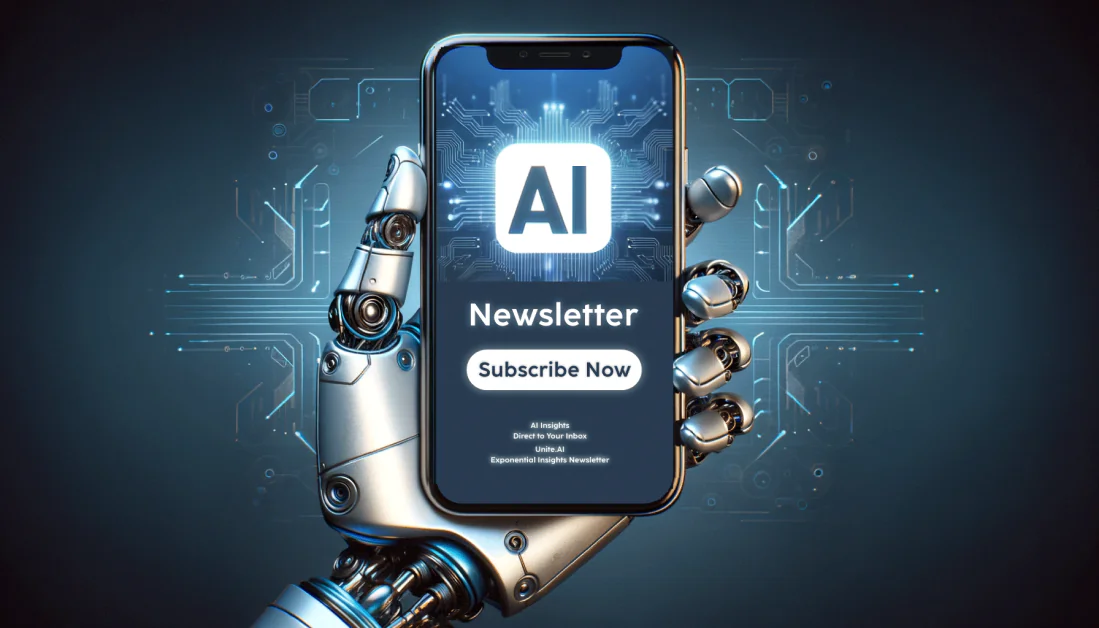