Thought Leaders
Will GPT-4 Bring Us Closer to a True AI Revolution?
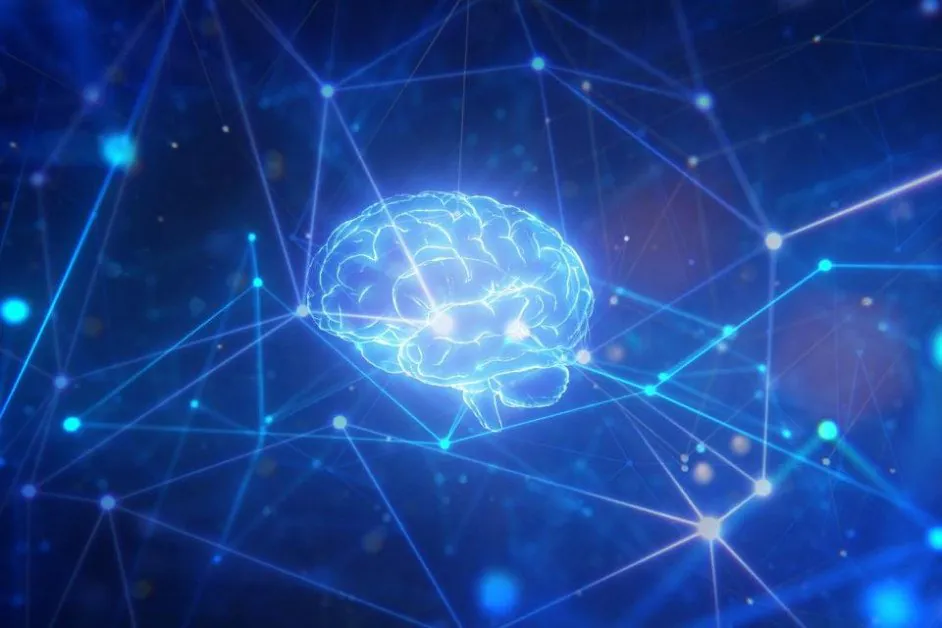
It’s been almost three years since GPT-3 was introduced, back in May 2020. Since then, the AI text-generation model has garnered a lot of interest for its ability to create text that looks and sounds like it was written by a human. Now it’s looking like the next iteration of the software, GPT-4, is just around the corner, with an estimated release date of sometime in early 2023.
Despite the highly anticipated nature of this AI news, the exact details on GPT-4 have been pretty sketchy. OpenAI, the company behind GPT-4, has not publicly disclosed much information on the new model, such as its features or its abilities. Nevertheless, recent advances in the field of AI, particularly regarding Natural Language Processing (NLP), may offer some clues on what we can expect from GPT-4.
What is GPT?
Before getting into the specifics, it’s helpful to first establish a baseline on what GPT is. GPT stands for Generative Pre-trained Transformer and refers to a deep-learning neural network model that is trained on data available from the internet to create large volumes of machine-generated text. GPT-3 is the third generation of this technology and is one of the most advanced AI text-generation models currently available.
Think of GPT-3 as operating a little like voice assistants, such as Siri or Alexa, only on a much larger scale. Instead of asking Alexa to play your favorite song or having Siri type out your text, you can ask GPT-3 to write an entire eBook in just a few minutes or generate 100 social media post ideas in less than a minute. All that the user needs to do is provide a prompt, such as, “Write me a 500-word article on the importance of creativity.” As long as the prompt is clear and specific, GPT-3 can write just about anything you ask it to.
Since its release to the general public, GPT-3 has found many business applications. Companies are using it for text summarization, language translation, code generation, and large-scale automation of almost any writing task.
That said, while GPT-3 is undoubtedly very impressive in its ability to create highly readable human-like text, it's far from perfect. Problems tend to crop up when prompted to write longer pieces, especially when it comes to complex topics that require insight. For example, a prompt to generate computer code for a website may return correct but suboptimal code, so a human coder still has to go in and make improvements. It's a similar issue with large text documents: the larger the volume of text, the more likely it is that errors – sometimes hilarious ones – will crop up that need fixing by a human writer.
Simply put, GPT-3 is not a complete replacement for human writers or coders, and it shouldn’t be thought of as one. Instead, GPT-3 should be viewed as a writing assistant, one that can save people a lot of time when they need to generate blog post ideas or rough outlines for advertising copy or press releases.
More parameters = better?
One thing to understand about AI models is how they use parameters to make predictions. The parameters of an AI model define the learning process and provide structure for the output. The number of parameters in an AI model has generally been used as a measure of performance. The more parameters, the more powerful, smooth, and predictable the model is, at least according to the scaling hypothesis.
For example, when GPT-1 was released in 2018, it had 117 million parameters. GPT-2, released a year later, had 1.2 billion parameters, while GPT-3 raised the number even higher to 175 billion parameters. According to an August 2021 interview with Wired, Andrew Feldman, founder and CEO of Cerebras, a company that partners with OpenAI, mentioned that GPT-4 would have about 100 trillion parameters. This would make GPT-4 100 times more powerful than GPT-3, a quantum leap in parameter size that, understandably, has made a lot of people very excited.
However, despite Feldman's lofty claim, there are good reasons for thinking that GPT-4 will not in fact have 100 trillion parameters. The larger the number of parameters, the more expensive a model becomes to train and fine-tune due to the vast amounts of computational power required.
Plus, there are more factors than just the number of parameters that determine a model’s effectiveness. Take for example Megatron-Turing NLG, a text-generation model built by Nvidia and Microsoft, which has more than 500 billion parameters. Despite its size, MT-NLG does not come close to GPT-3 in terms of performance. In short, bigger does not necessarily mean better.
Chances are, GPT-4 will indeed have more parameters than GPT-3, but it remains to be seen whether that number will be an order of magnitude higher. Instead, there are other intriguing possibilities that OpenAI is likely pursuing, such as a leaner model that focuses on qualitative improvements in algorithmic design and alignment. The exact impact of such improvements is hard to predict, but what is known is that a sparse model can reduce computing costs through what's called conditional computation, i.e., not all parameters in the AI model will be firing all the time, which is similar to how neurons in the human brain operate.
So, what will GPT-4 be able to do?
Until OpenAI comes out with a new statement or even releases GPT-4, we're left to speculate on how it will differ from GPT-3. Regardless, we can make some predictions
Although the future of AI deep-learning development is multimodal, GPT-4 will likely remain text-only. As humans, we live in a multisensory world that is filled with different audio, visual, and textual inputs. Therefore, it’s inevitable that AI development will eventually produce a multimodal model that can incorporate a variety of inputs.
However, a good multimodal model is significantly more difficult to design than a text-only model. The tech simply isn’t there yet and based on what we know about the limits on parameter size, it’s likely that OpenAI is focusing on expanding and improving upon a text-only model.
It’s also likely that GPT-4 will be less dependent on precise prompting. One of the drawbacks of GPT-3 is that text prompts need to be carefully written to get the result you want. When prompts are not carefully written, you can end up with outputs that are untruthful, toxic, or even reflecting extremist views. This is part of what’s known as the “alignment problem” and it refers to challenges in creating an AI model that fully understands the user’s intentions. In other words, the AI model is not aligned with the user’s goals or intentions. Since AI models are trained using text datasets from the internet, it’s very easy for human biases, falsehoods, and prejudices to find their way into the text outputs.
That said, there are good reasons for believing that developers are making progress on the alignment problem. This optimism comes from some breakthroughs in the development of InstructGPT, a more advanced version of GPT-3 that is trained on human feedback to follow instructions and user intentions more closely. Human judges found that InstructGPT was far less reliant than GPT-3 on good prompting.
However, it should be noted that these tests were only conducted with OpenAI employees, a fairly homogeneous group that may not differ a lot in gender, religious, or political views. It's likely a safe bet that GPT-4 will undergo more diverse training that will improve alignment for different groups, though to what extent remains to be seen.
Will GPT-4 replace humans?
Despite the promise of GPT-4, it’s unlikely that it will completely replace the need for human writers and coders. There is still much work to be done on everything from parameter optimization to multimodality to alignment. It may well be many years before we see a text generator that can achieve a truly human understanding of the complexities and nuances of real-life experience.
Even so, there are still good reasons to be excited about the coming of GPT-4. Parameter optimization – rather than mere parameter growth – will likely lead to an AI model that has far more computing power than its predecessor. And improved alignment will likely make GPT-4 far more user-friendly.
In addition, we’re still only at the beginning of the development and adoption of AI tools. More use cases for the technology are constantly being found, and as people gain more trust and comfort with using AI in the workplace, it’s near certain that we will see widespread adoption of AI tools across almost every business sector in the coming years.
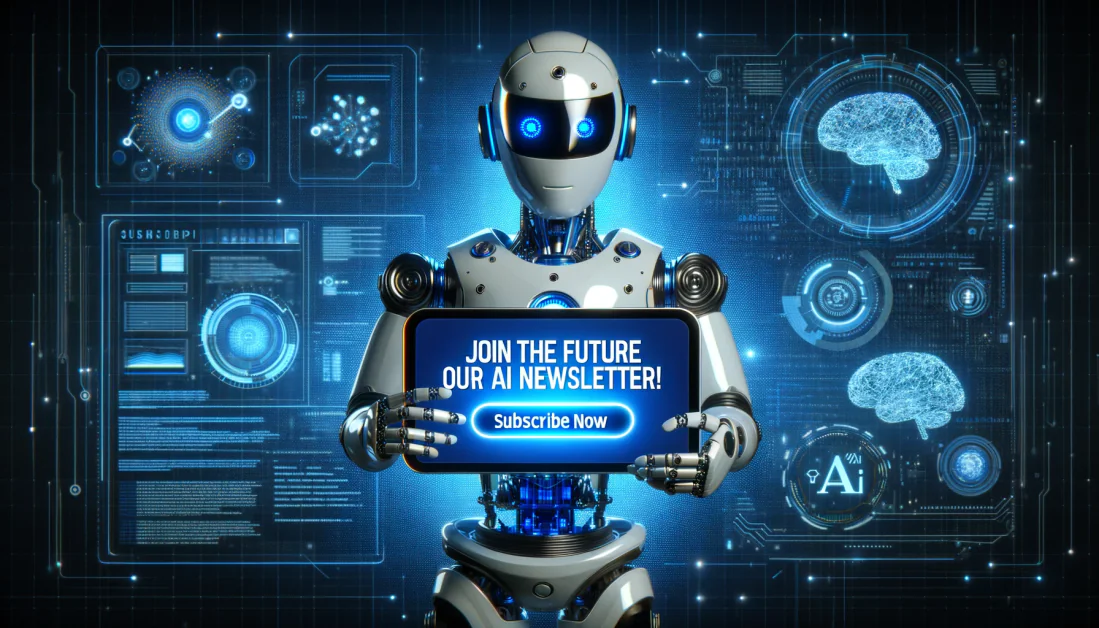
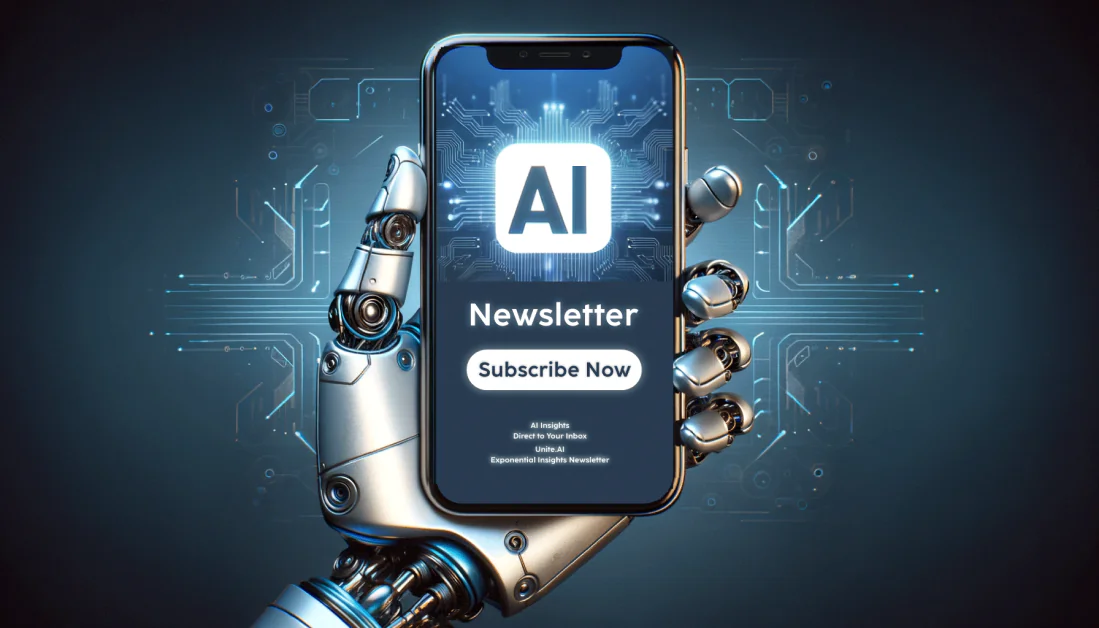