Artificial Intelligence
The ‘Nonsense Language’ That Could Subvert Image Synthesis Moderation Systems
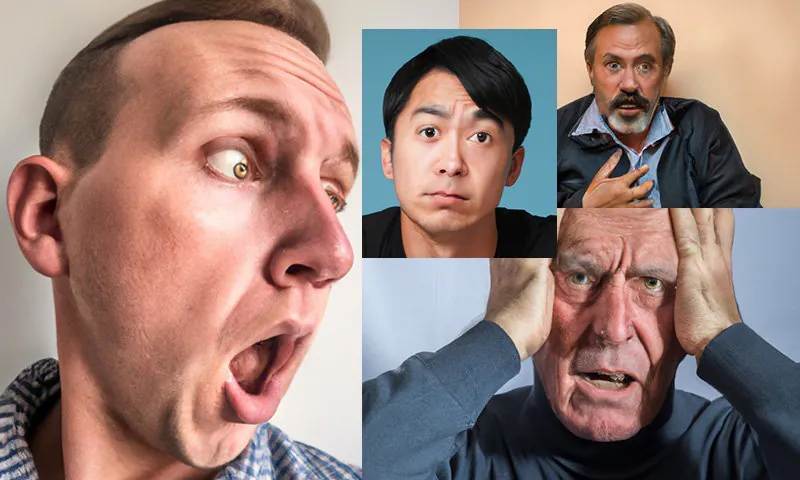
New research from Columbia university suggests that the safeguards that prevent image synthesis models such as DALL-E 2, Imagen and Parti from being able to output damaging or controversial imagery are susceptible to a kind of adversarial attack that involves ‘made up' words.
The author has developed two approaches that can potentially override the content moderation measures in an image synthesis system, and has found that they are remarkably robust even across different architectures, indicating that the weakness is more than just systemic, and may key on some of the most fundamental principle of text-to-image synthesis.
The first, and the stronger of the two, is called macaronic prompting. The term ‘macaronic' originally refers to a mixture of multiple languages, as found in Esperanto or Unwinese. Perhaps the most culturally-diffused example would be Urdu-English, a type of ‘code mixing' common in Pakistan, which quite freely mixes English nouns and Urdu suffixes.
In some of the above examples, fractions of meaningful words have been glued together, using English as a ‘scaffold'. Other examples in the paper use multiple languages across a single prompt.
The system will respond in a semantically meaningful way because of the relative lack of curation in the web sources on which the system was trained. Such sources will very often have arrived complete with multilingual labels (i.e. from datasets not specifically designed for an image synthesis task), and each word ingested, in whatever language, will become a ‘token'; but likewise parts of those words will become ‘subwords' or fractional tokens. In Natural Language Processing (NLP), this kind of ‘stemming' helps distinguish the etymology of longer derived words that may arise in transformation operations, but also creates a massive lexical ‘Lego set' which ‘creative' prompting can leverage.
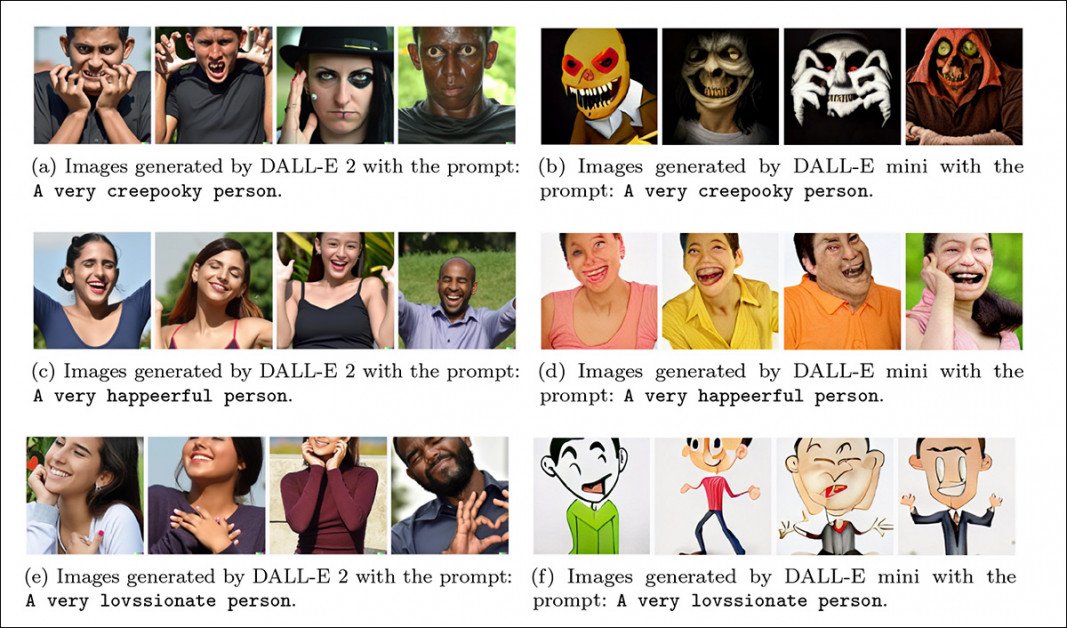
Monolingual portmanteau words are also effective in obtaining images through indirect or non-prosaic language, with very similar results often obtainable across differing architectures, such as DALL-E 2 and DALL-E Mini (Craiyon).
In the second type of approach, called evocative prompting, Some of the conjoined words are similar in tone to the more juvenile strand of ‘schoolboy Latin' demonstrated in Monty Python's Life of Brian (1979).
The author states:
‘An obvious concern with this method is the circumvention of content filters based on blacklisted prompts. In principle, macaronic prompting could provide an easy and seemingly reliable way to bypass such filters in order to generate harmful, offensive, illegal, or otherwise sensitive content, including violent, hateful, racist, sexist, or pornographic images, and perhaps images infringing on intellectual property or depicting real individuals.
‘Companies that offer image generation as a service have put a great deal of care into preventing the generation of such outputs in accordance with their content policy. Consequently, macaronic prompting should be systematically investigated as a threat to the safety protocols used for commercial image generation.'
The author suggests a number of remedies against this vulnerability, some of which he concedes might be considered over-restrictive.
The first possible solution is the most expensive: to curate the source training images more carefully, with more human and less algorithmic oversight. However, the paper concedes that this would not prevent the image synthesis system from creating an offensive conjunction between two image concepts that are by themselves potentially innocuous.
Secondly, the paper suggests that image synthesis systems could run their actual output through a filter system, intercepting any problematic associations before they are served up to the user. It is possible that DALL-E 2 currently operates such a filter, though OpenAI has not disclosed exactly how DALL-E 2's content moderation works.
Finally, the author considers the possibility of a ‘dictionary whitelist', which would only allow vetted and approved words to retrieve and render concepts, but concedes that this could represent an excessively severe restriction on the utility of the system.
Though the researcher only experimented with five languages (English, German, French, Spanish and Italian) in creating prompt-assemblies, he believes this kind of ‘adversarial attack' could become even more ‘cryptic' and difficult to deter by extending the number of languages, given that hyperscale models such as DALL-E 2 are trained on multiple languages (simply because it is easier to use lightly-filtered or ‘raw' input than to consider the huge expense of curating it, and because the extra dimensionality is likely to add to the usefulness of the system).
The paper is titled Adversarial Attacks on Image Generation With Made-Up Words, and comes from Raphaël Millière at Columbia University.
Cryptic Language in DALL-E 2
It has been suggested before that the gibberish that DALL-E 2 outputs whenever it tries to depict written language could in itself be a ‘hidden vocabulary'. However the prior research into this mysterious language has not offered any way to develop nonce strings that can summon up specific imagery.
Of the previous work, the paper states:
‘[It] does not offer a reliable method to find nonce strings that elicit specific imagery. Most of the gibberish text included by DALL-E 2 in images does not seem to be reliably associated with specific visual concepts when transcribed and used as a prompt. This limits the viability of this approach as way to circumvent the moderation of harmful or offensive content; as such, it is not a particularly concerning risk for the misuse of text-guided image generation models.'
Instead, the author's two methods are elaborated as means by which nonsense can summon related and meaningful imagery whilst bypassing the conventional etiquette that is now developing into prompt engineering.
By way of example, the author considers the word for ‘birds' in the five languages that are in the scope of the paper: Vögel in German, uccelli in Italian, oiseaux in French, and pájaros in Spanish.
With the byte-pair encoding (BPE) tokenization used by the implementation of CLIP that's integrated into DALL-E 2 , the words are tokenized into non-accented English, and can be ‘creatively combined' to form nonce words that seem to be gibberish to us, but retain their glued-together meaning for DALL-E 2, allowing the system to express the perceived intent:
In the above example, two of the ‘foreign' words for bird are glued together into a nonsense string. Thanks to the fractional weight of the sub-words, the meaning is retained.
The author emphasizes that meaningful results can also be obtained without adhering to the boundaries of subword segmentation, presumably because DALL-E 2 (the primary study of the paper) has generalized well enough to let the boundaries of the sub-words blur without destroying their meaning.
To further demonstrate the approaches developed, the paper offers examples of macaronic prompting across different domains, using the list of token words illustrated below (with nonsense hybridized words on the far right).
The author states that the following examples from DALL-E 2 are not ‘cherry-picked':
Lingua Franca
The paper also observes that several such examples work equally well, or at least very similarly, across both DALL-E 2 and DALL-E Mini (now Craiyon), and that this is surprising, since DALL-E 2 is a diffusion model and DALL-E Mini is not; the two systems are trained on different datasets; and DALL-E Mini uses a BART tokenizer instead of the CLIP tokenizer favored by DALL-E 2.
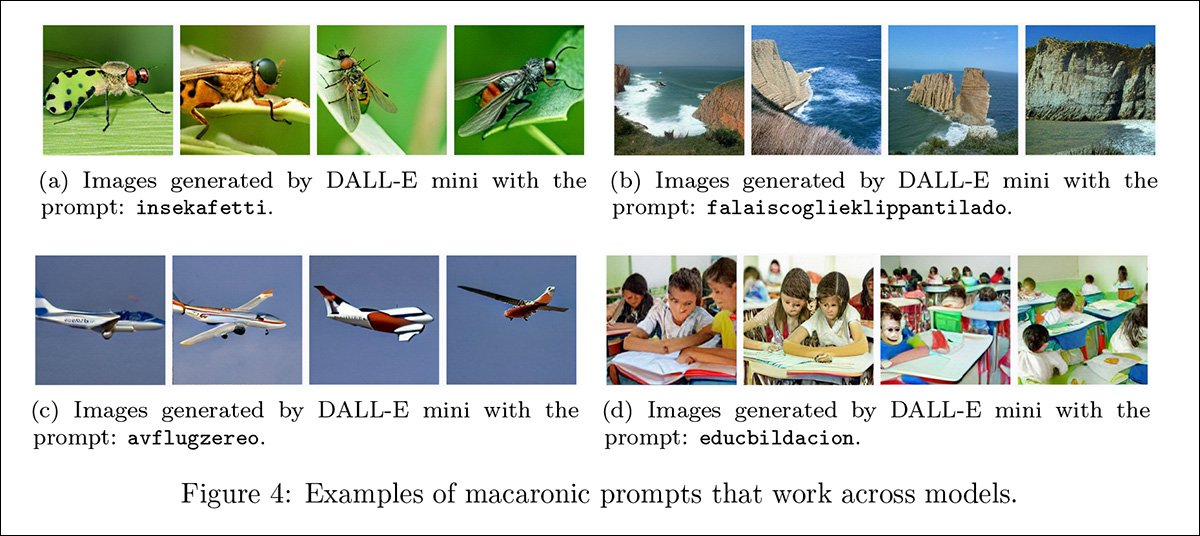
Remarkably similar results from DALL-E Mini, compared to the previous image, which featured results from the same ‘nonsense' input from DALL-E 2.
As seen in the first of the images above, macaronic prompting can also be assembled into syntactically sound sentences in order to generate more complex scenes. However, this requires using English as a ‘scaffold' to assemble the concepts, making the procedure more likely to be intercepted by standard censor systems in an image synthesis framework.
The paper observes that lexical hybridization, the ‘gluing together' of words to elicit related content from an image synthesis system, can also be accomplished in a single language, by the use of portmanteau words.
Evocative Prompting
The ‘evocative prompting' approach featured in the paper depends on ‘evoking' a broader response from the system with words that are not strictly based on subwords or sub-tokens or partially shared labels.
One type of evocative prompting is pseudolatin, which can, among other uses, generate images of fictional medicines, even without any specification that DALL-E 2 should retrieve the concept of ‘medicine':
Evocative prompting also works particularly well with nonsensical prompts that relate broadly to possible geographical locations, and works quite reliably across the different architectures of DALL-E 2 and DALL-E Mini:
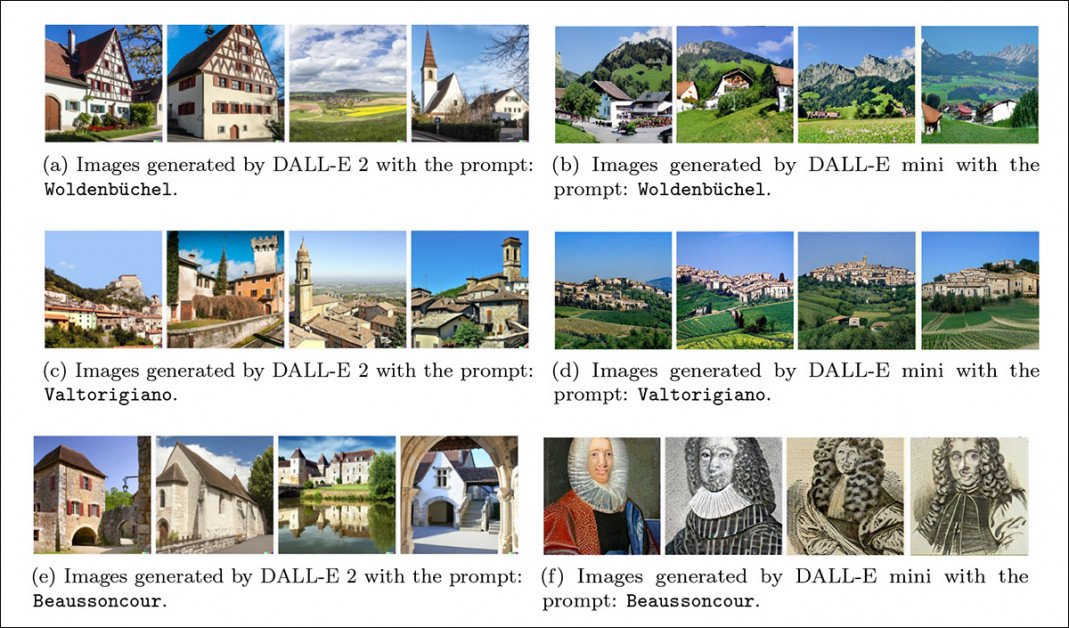
The words used for these prompts to DALL-E 2 and DALL-E Mini are redolent of real names, but are in themselves utter nonsense. Nonetheless, the systems have ‘picked up the atmosphere' of the words.
There appears to be some crossover between macaronic and evocative prompting. The paper states:
‘It seems that differences in training data, model size, and model architecture may cause different models to parse prompts like voiscellpajaraux and eidelucertlagarzard in either “macaronic” or “evocative” fashion, even when these models are proven to be responsive to both prompting methods.'
The paper concludes:
‘While various properties of these models – including size, architecture, tokenization [procedure] and training data – may influence their vulnerability to text-based adversarial attacks, preliminary evidence discussed in this work suggests that some of these attacks may nonetheless work somewhat reliably across models.'
Arguably the biggest obstacle to true experimentation around these methods is the risk of being flagged and banned by the host system. DALL-E 2 requires an associated phone number for each user account, limiting the number of ‘burner accounts' that would likely be needed to truly test the boundaries of this kind of lexical hacking, in terms of subverting the existing moderation methods. Currently, DALL-E 2's primary safeguard remains volatility of access.
First published 9th August 2022.
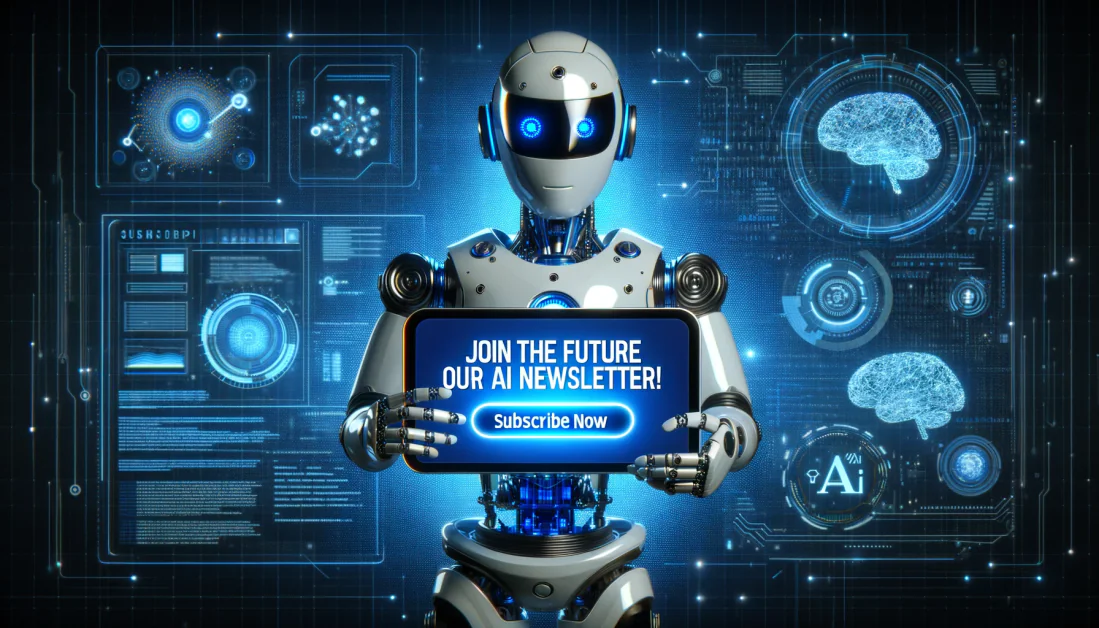
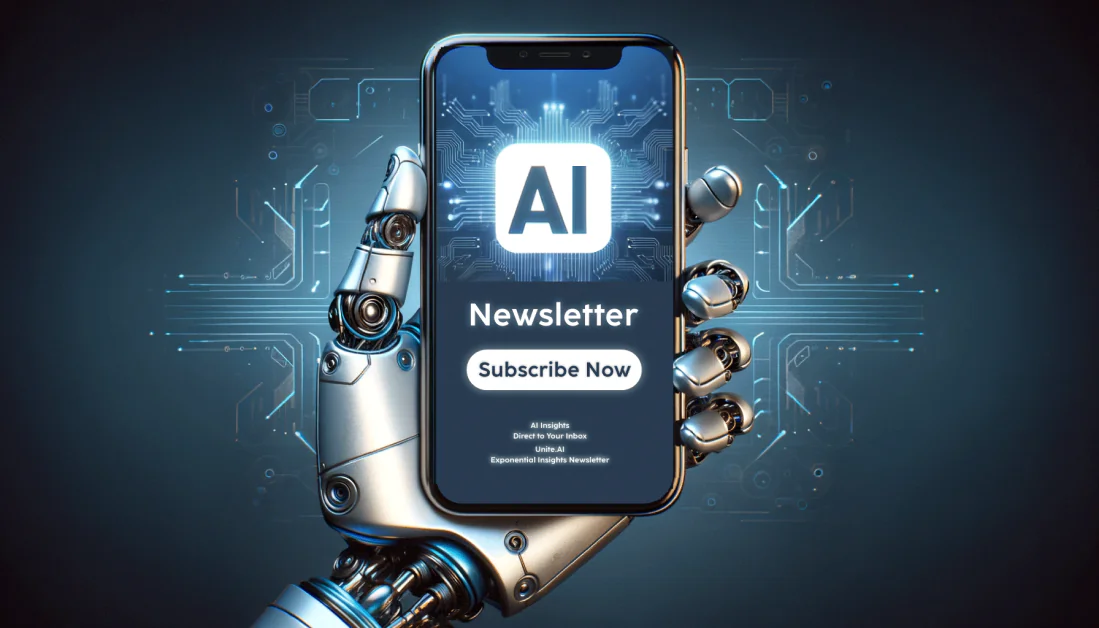