Artificial Intelligence
Technique Enables AI to Think Far Into Future
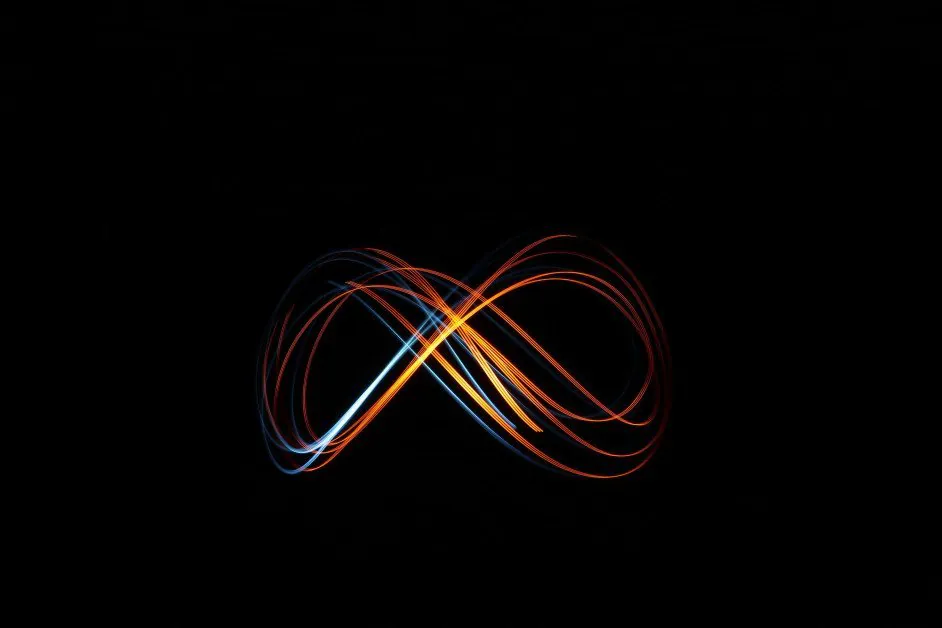
A team of researchers from MIT, the MIT-IBM Watson AI Lab, and other institutions has developed a new approach that enables artificial intelligence (AI) agents to achieve a farsighted perspective. In other words, the AI can think far into the future when considering how their behaviors can include the behaviors of other AI agents when completing a task.
The research is set to be presented at the Conference on Neural Information Processing Systems.
AI Considering Other Agents’ Future Actions
The machine-learning framework created by the team enables cooperative or competitive AI agents to consider what other agents will do. This is not just over the next steps but rather as time approaches infinity. The agents adapt their behaviors accordingly to influence other agents’ future behaviors, helping them arrive at optimal, long-term solutions.
According to the team, the framework could be used, for example, by a group of autonomous drones working together to find a lost hiker. It could also be used by self-driving vehicles to anticipate the future moves of other vehicles to improve passenger safety.
Dong-Ki Kim is a graduate student in the MIT Laboratory for Information and Decision Systems (LIDS) and lead author of the research paper.
“When AI agents are cooperating or competing, what matters most is when their behaviors converge at some point in the future,” Kim says. “There are a lot of transient behaviors along the way that don’t matter very much in the long run. Reaching this converged behavior is what we really care about, and we now have a mathematical way to enable that.”
The problem tackled by the researchers is called multi-agent reinforcement learning, with reinforcement learning being a form of machine learning where AI agents learn by trial and error.
Whenever there are multiple cooperative or competing agents simultaneously learning, the process can become far more complex. As agents consider more future steps of the other agents, as well as their own behavior and how it influences others, the problem requires too much computational power.
AI Thinking About Infinity
“The AI’s really want to think about the end of the game, but they don’t know when the game will end,” Kim says. “They need to think about how to keep adapting their behavior into infinity so they can win at some far time in the future. Our paper essentially proposes a new objective that enables an AI to think about infinity.”
It’s impossible to integrate infinity into an algorithm, so the team designed the system in a way that agents focus on a future point where their behavior will converge with other agents. This is referred to as equilibrium, and an equilibrium point determines the long-term performance of agents.
It is possible for multiple equilibria to exist in a multi-agent scenario, and when an effective agent actively influences the future behaviors of other agents, they can reach a desirable equilibrium from the agent’s perspective. When all agents influence each other, they converge to a general concept referred to as an “active equilibrium.”
FURTHER Framework
The team’s machine learning framework is called FURTHER, and it enables agents to learn how to adjust their behaviors based on their interactions with other agents to achieve active equilibrium.
The framework relies on two machine-learning modules. The first is an inference module that enables an agent to guess the future behaviors of other agents and the learning algorithms they use based on prior actions. The information is then fed into the reinforcement learning module, which the agent relies on to adapt its behavior and influence other agents.
“The challenge was thinking about infinity. We had to use a lot of different mathematical tools to enable that, and make some assumptions to get it to work in practice,” Kim says.
The team tested their method against other multiagent reinforcement learning frameworks in different scenarios where the AI agents using FURTHER came out ahead.
The approach is decentralized, so the agents learn to win independently. On top of that, it is better designed to scale when compared to other methods that require a central computer to control the agents.
According to the team, FURTHER could be used in a wide range of multi-agent problems. Kim is especially hopeful for its applications in economics, where it could be applied to develop sound policy in situations involving many interacting entities with behaviors and interests that change over time.
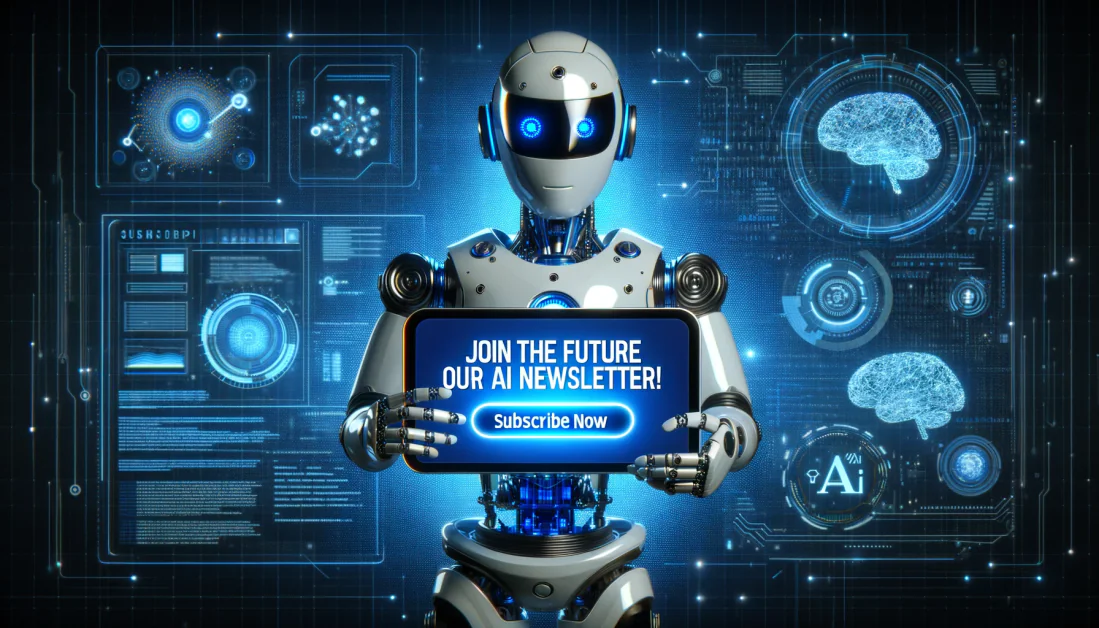
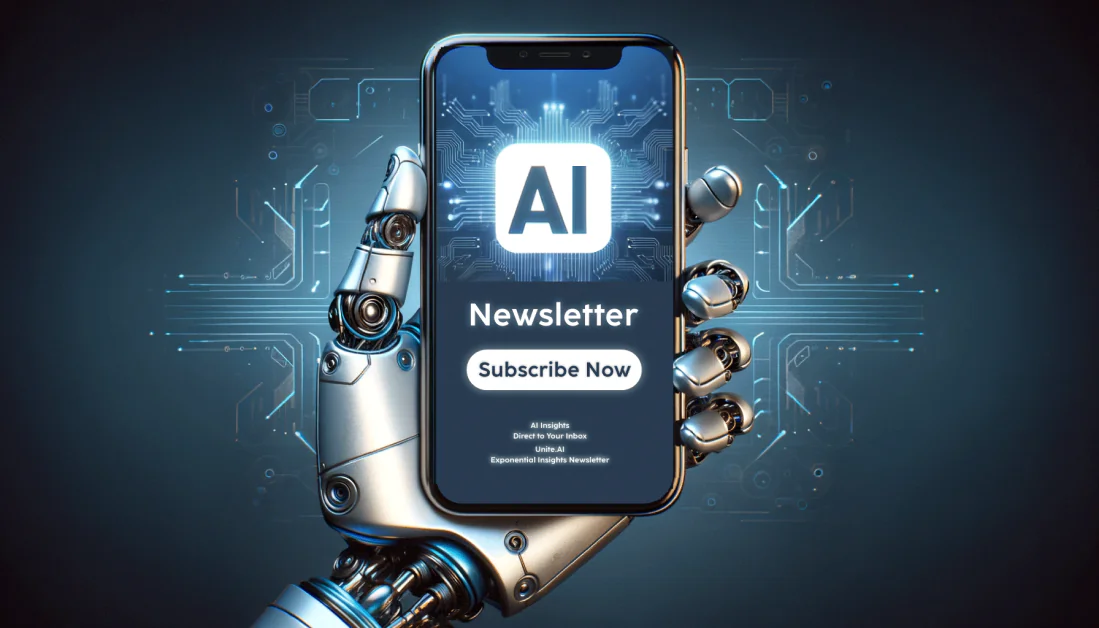