Robotics
Teaching Robots to Anticipate Human Preferences for Enhanced Collaboration
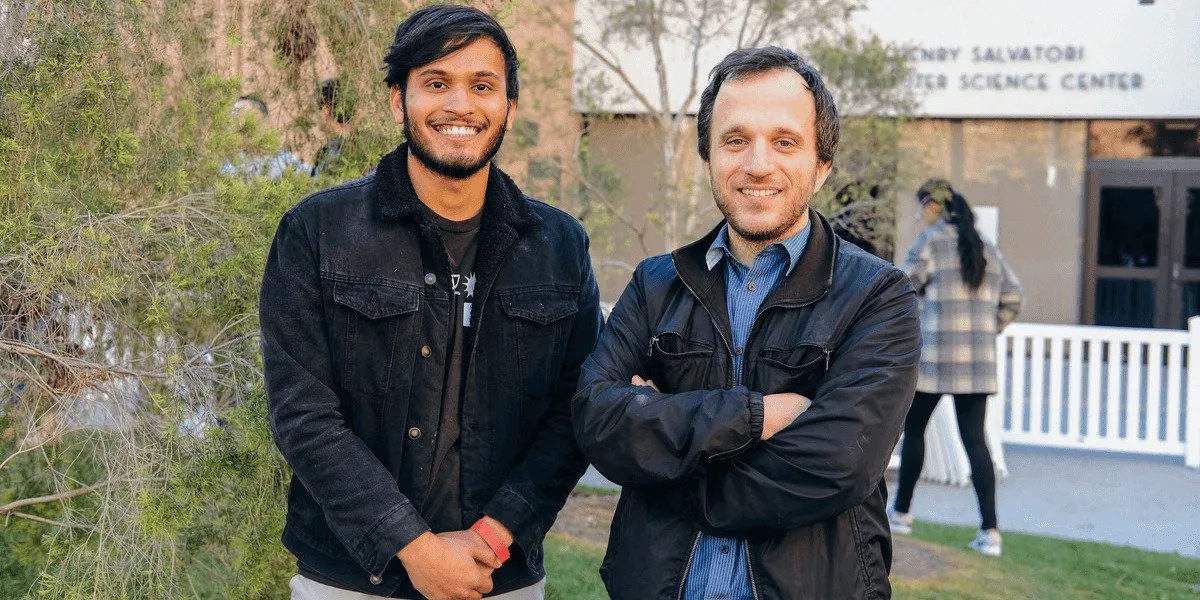
Humans possess the unique ability to understand the goals, desires, and beliefs of others, which is crucial for anticipating actions and collaborating effectively. This skill, known as “theory of mind,” is innate to us but remains a challenge for robots. However, if robots are to become truly collaborative helpers in manufacturing and daily life, they need to learn these abilities as well.
In a new paper, which was a finalist for the best paper award at the ACM/IEEE International Conference on Human-Robot Interaction (HRI), computer science researchers from USC Viterbi aim to teach robots to predict human preferences in assembly tasks. This will allow robots to one day assist in various tasks, from building satellites to setting a table.
“When working with people, a robot needs to constantly guess what the person will do next,” said lead author Heramb Nemlekar, a USC computer science PhD student supervised by Stefanos Nikolaidis, an assistant professor of computer science. “For example, if the robot thinks the person will need a screwdriver to assemble the next part, it can get the screwdriver ahead of time so that the person does not have to wait. This way the robot can help people finish the assembly much faster.”
A New Approach to Predicting Human Actions
Predicting human actions can be challenging, as different people prefer to complete the same task in various ways. Current techniques require people to demonstrate how they would like to perform the assembly, which can be time-consuming and counterproductive. To address this issue, the researchers discovered similarities in how individuals assemble different products and used this knowledge to predict preferences.
Instead of requiring individuals to “show” the robot their preferences in a complex task, the researchers created a small assembly task (referred to as a “canonical” task) that could be quickly and easily performed. The robot would then “watch” the human complete the task using a camera and utilize machine learning to learn the person's preference based on their sequence of actions in the canonical task.
In a user study, the researchers' system was able to predict human actions with around 82% accuracy. This approach not only saves time and effort but also helps build trust between humans and robots. It could be beneficial in industrial settings, where workers assemble products on a large scale, as well as for persons with disabilities or limited mobility who require assistance in assembling products.
Towards a Future of Enhanced Human-Robot Collaboration
The researchers' goal is not to replace human workers but to improve safety and productivity in human-robot hybrid factories by having robots perform non-value-added or ergonomically challenging tasks. Future research will focus on developing a method to automatically design canonical tasks for different types of assembly tasks and evaluating the benefits of learning human preferences from short tasks and predicting actions in complex tasks in various contexts, such as personal assistance in homes.
“A robot that can quickly learn our preferences can help us prepare a meal, rearrange furniture, or do house repairs, having a significant impact on our daily lives,” said Nikolaidis.
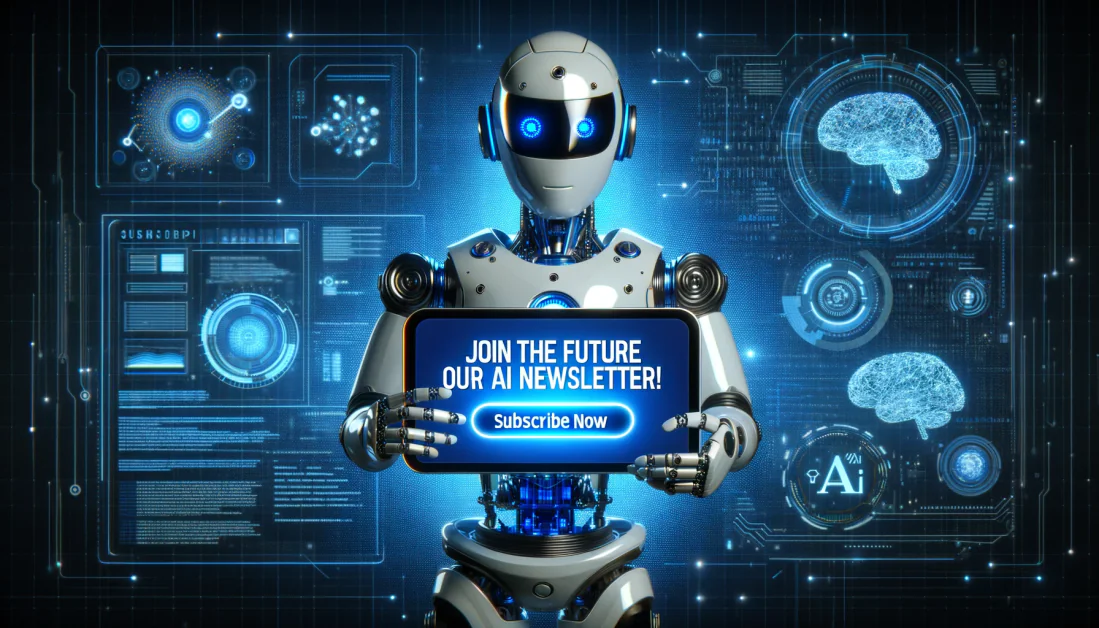
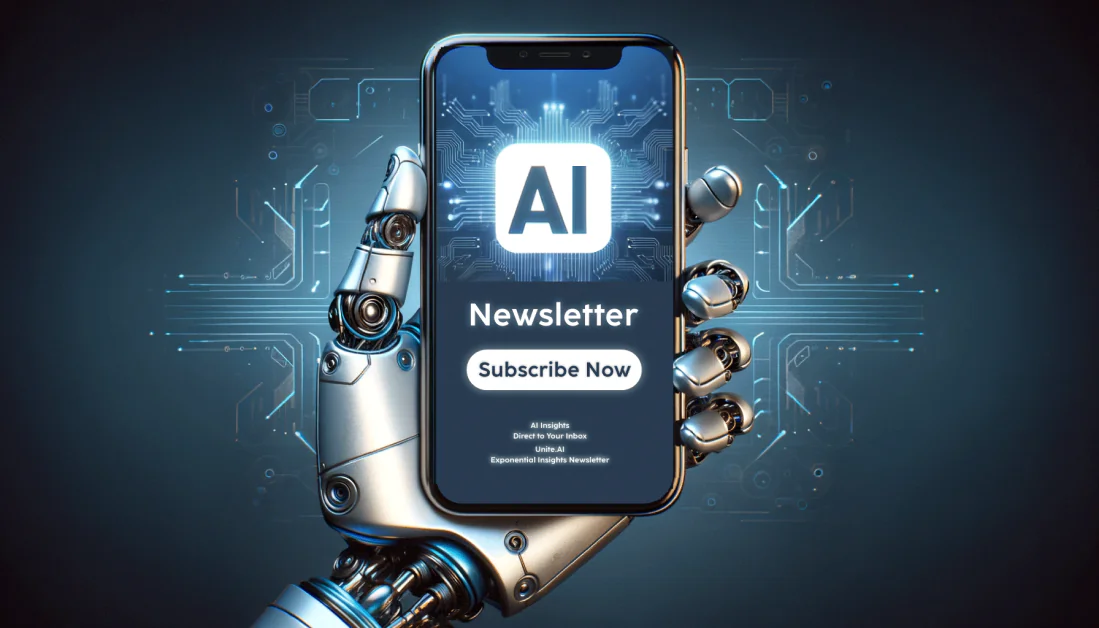