Healthcare
‘Speech Neuroprosthesis’ Technology Restores Speech to Patient With Severe Paralysis
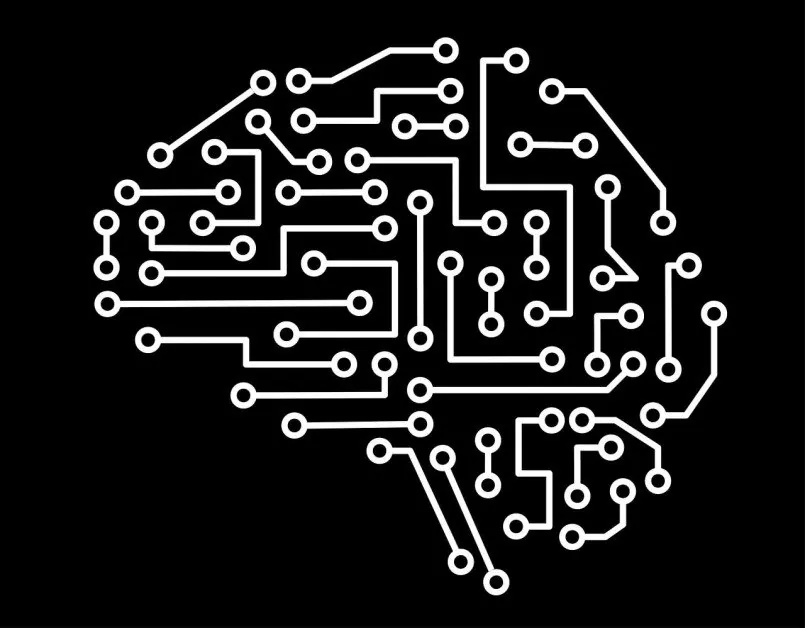
In another major development in artificial intelligence (AI) prosthetics, researchers at the University of California San Francisco have successfully developed a “speech neuroprosthesis” that partly restored speech to a man with severe paralysis. The new technology helped him speak in sentences when it translated signals from his brain to the vocal tract. The words then appeared as text on a screen.
The work involved the first participant of a clinical research trial, and it was part of a larger body of work that has been taking place for over ten years by UCSF neurosurgeon Edward Chang, MD, who has been attempting to develop a technology that enables people with paralysis to communicate even when they are unable to speak on their own.
The study was published on July 15 in the New England Journal of Medicine.
First System of Its Kind
Chang is the Joan and Sanford Weill Chair of Neurological Surgery at UCSF and Jeanne Robertson Distinguished Professor. He is also senior author of the study.
“To our knowledge, this is the first successful demonstration of direct decoding of full words from the brain activity of someone who is paralyzed and cannot speak,” said Chang. “It shows strong promise to restore communication by tapping into the brain's natural speech machinery.”
Work in this field traditionally revolves around restoring communication through spelling-based approaches to write out letters one-by-one in text. However, the new study focuses on translating signals that are actually intended to control muscles of the vocal system for speaking words. This is different from the traditional work, which focuses on the signals that move the arm or hand.
According to Chang, the new approach leverages the natural and fluid aspects of speech, and it could lead to far more advances in this area. He also said that spelling-based approaches that rely on typing, writing, and controlling a cursor are far slower.
“With speech, we normally communicate information at a very high rate, up to 150 or 200 words per minute,” he said. “Going straight to words, as we're doing here, has great advantages because it's closer to how we normally speak.”
Chang’s previous work relied on patients at the UCSF Epilepsy Center who were undergoing neurosurgery to detect what was causing their seizures, and it used electrode arrays that were placed on the surface of the patients’ brains. The patients had normal speech, and the results helped lead to the current trial for individuals with paralysis.
Some of the new methods developed by the team included a way to decode cortical activity patterns and statistical language to improve accuracy.
David Moses, PhD, is a postdoctoral engineer in the Chang Lab and another one of the lead authors.
“Our models needed to learn the mapping between complex brain activity patterns and intended speech,” said Moses. “That poses a major challenge when the participant can't speak.”
The First Participant
The trial’s first participant was a man in his late 30s who suffered a brainstem stroke over 15 years ago that left the connection between his brain and vocal tract and limbs severely damaged.
By developing a 50-word vocabulary that Chang’s team could use advanced computer algorithms to recognize, the participant was able to create hundreds of sentences expressing daily life concepts.
He was required to have a high-density electrode array implanted over his speech motor cortex, and following his recovery, over 22 hours of neural activity in this brain region was recorded over 48 sessions.
Sean Metzger, MS and Jessie Liu, BS, are both bioengineering doctoral students in the Chang Lab and were responsible for developing custom neural network models that could translate the patterns of recorded neural activity into specific intended words.
Following the test, the team found that the system could decode words from brain activity at a rate of up to 18 words per minute, and it was 93 percent accurate. The team applied an “auto-correct” function to the language model, which helped improve the accuracy.
“We were thrilled to see the accurate decoding of a variety of meaningful sentences,” Moses said. “We've shown that it is actually possible to facilitate communication in this way and that it has potential for use in conversational settings.”
The team will now expand the trial to include more participants suffering from severe paralysis and communication issues. They are also expanding the number of words in the vocabulary and working on improving the rate of speech.
“This is an important technological milestone for a person who cannot communicate naturally,” said Moses, “and it demonstrates the potential for this approach to give a voice to people with severe paralysis and speech loss.”
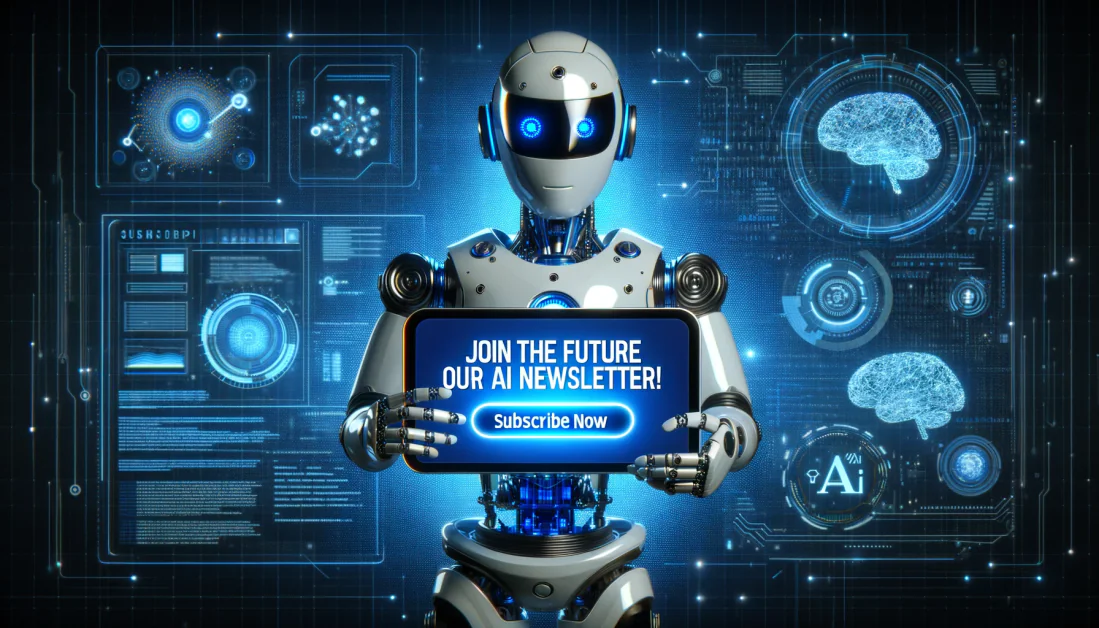
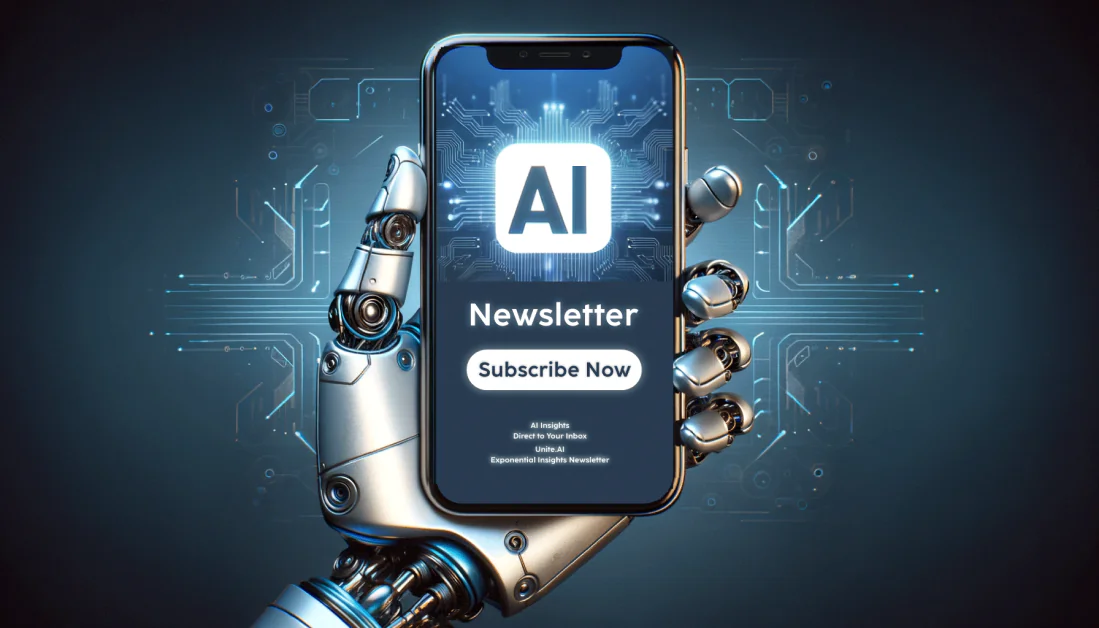