Interviews
Sohaib Khan, Co-Founder & CEO of Hazen.ai – Interview Series
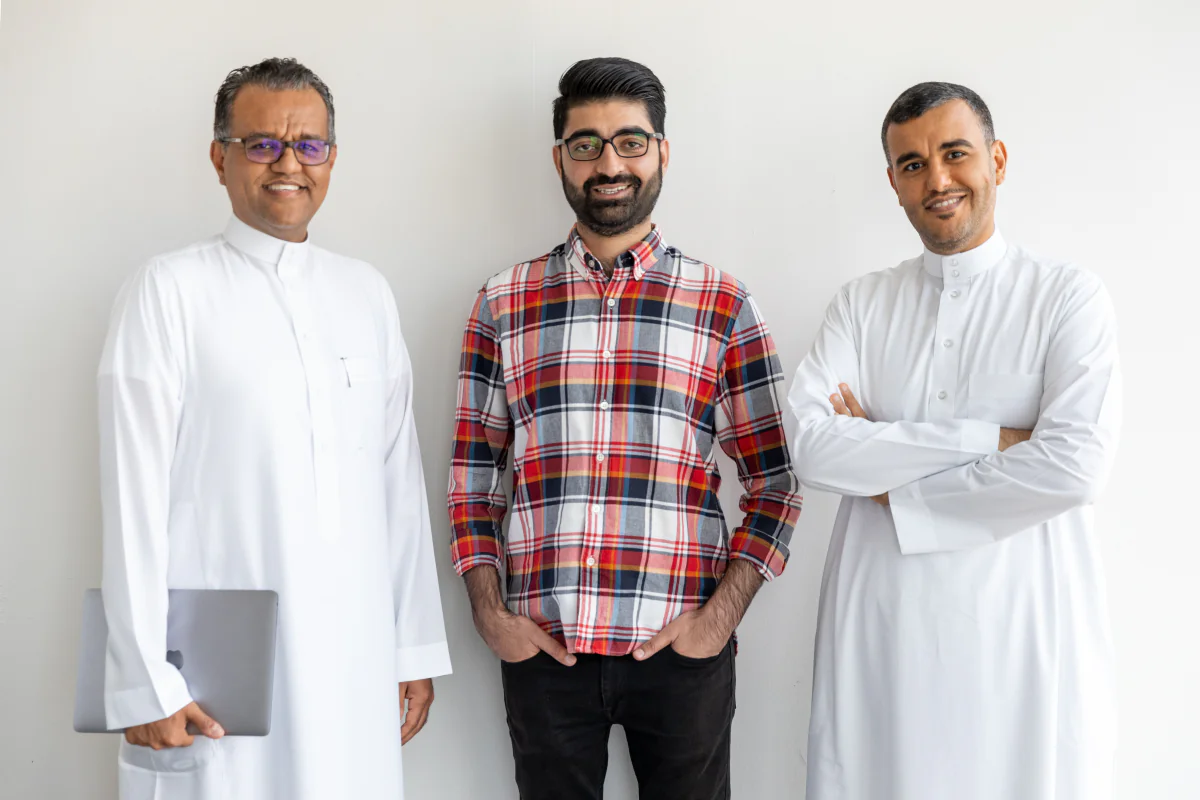
Sohaib Khan, is the Co-Founder & CEO of Hazen.ai, a company that uses computer vision and deep learning to design intelligent traffic analytics software that is designed to ‘understand’ the motion of every vehicle.
What initially attracted you to the field of AI?
It was during undergraduate that I first read about how stereo-vision (or binocular vision – estimating depth from two cameras) works. That got me hooked on exploring computer vision more. Interestingly, I first read about it in a book that I picked up from a traditional Friday market where they sold old used books on a road-side sidewalk in our hometown. I went on to do a PhD in this field from the US.
You were previously a professor at one of the largest universities in Pakistan The Lahore University of Management Sciences (LUMS). What were your teaching and research interests?
When I joined LUMS after my PhD, I built what was the first graduate research lab in the university, from funding I received from a large grant from a defense organization. The graduate program in CS was very new, and there were no research labs at that time. I taught Computer Vision for 12+ years at LUMS, and had an active lab in this field. In the beginning, computer vision was hardly taught at any Pakistani university, but later on, in became a standard subject, and actually, many of my students are now also teaching in Pakistani universities.
Can you discuss what inspired you to launch a startup that specializes in computer vision and deep learning algorithms for video analytics?
Computer Vision, for a long time, was largely an experimental research field, with limited applications in products. This was primarily because the maturity of algorithms needed for building products was not there. For a product, the image understanding algorithm has to work in a variety of imaging and lighting conditions, and not in just some very controlled experiments. We had a joke amongst the graduate students in our lab when I was doing my PhD back in 2000, that if you can find three images on which your algorithm works, you can write a paper. If it works on three videos, you get a very good paper! The point is that a lot of vision algorithms worked only in carefully curated laboratory scenarios, and were not very robust.
But now things have changed. With the advent of deep learning in 2012, we have seen some very rapid and fascinating progress in image understanding. When we saw that, we felt that now the timing is right to perhaps build solid products that can have a significant impact.
What type of traffic violations can Hazen.ai monitor?
Our aim is to be able to identify all types of dangerous driving behavior on the roads. This is driving by our overarching objective of reducing road fatalities. Every 24 seconds, someone dies in a road crash, which is equivalent to about 15 787-8 Dreamliners crashing every single day! So this is really what motivates us. That is why we are building software that can detect different types of dangerous and unsafe behaviors, like unsafe lane changes, illegal turns, jumping a red-light or a stop-sign, blocking a pedestrian crossing, not wearing a seat-belt or texting-while-driving. We are also working towards building features in our software specifically for the safety of pedestrians and cyclists, because more than half the fatalities in road-crashes occur in the vulnerable road-user segment of pedestrians, cyclists and motor-cyclists.
What are some of the unique challenges behind using computer vision to monitor objects moving at such high speeds?
There are two types of challenges: First is the performance of computer vision algorithms themselves – you want to have a product that can work in challenging traffic conditions 24/7 in all lighting variations. While there has been a lot of progress technically towards this goal, there are still countries in which the density of road-users is so high, like clusters of motor-bikes or pedestrians in very close proximity, that it is still challenging for algorithms to track them individually and understand the scene. But secondly, a bigger challenge is making a solid product out of computer vision algorithms, that can be deployed on limited hardware resources at the edge, and can be monitored and managed easily despite being distributed all over the city. Since computer vision products handle a lot of video data, deploying them on the edge, as an IoT device, and managing them effectively, remains a difficult task.
What’s the process for the end user to configure the software to different road configurations?
Every intersection provides a unique scenario, in terms of traffic volume, lane configuration and the type of vehicle, cyclists or pedestrian interactions. Moreover, the interest of traffic managers might be specific, to identify a particular type of traffic behavior at each site. For example, the traffic police might disallow a U-turn at an intersection to smooth out traffic flow, and are interested in capturing that statistic. This is why we have kept our software configurable to different scenarios. When a camera is set up with our software, we configure it through a simple process for what the end-user requires at that site. Internally, we have built a high-level language in which we can compactly describe traffic scenarios of interest in a simple way. This allows us to configure a site quickly for our customers.
What type of hardware is needed to operate this system?
Video analytics requires significant compute power. We have optimized our code to run on the smaller Nvidia GPUs which can be deployed at the edge, like their Jetson series, and also on Intel CPUs for certain features that we offer. In recent years, more powerful edge hardware is becoming available at a reasonable price point, so this is really driving a lot of exciting applications.
Can you discuss if any jurisdictions are currently trialing or using the Hazen.ai technology?
We now have on-going trials in several countries, UK, USA, Egypt, Saudi Arabia, Pakistan, Oman, Peru and are engaging potential customers in other countries too.
Is there anything else that you would like to share about Hazen.ai?
Overall, we feel that traffic safety technologies have not progressed enough, compared to the scale of the problem. However, now the time is right, because of the massive progress in computer vision and deep learning, as well as cheap availability of camera and compute hardware. We will see many more applications of edge-based computer vision in the coming years. These are the fundamentals that drive Hazen.ai.
Thank you for the interview, readers who wish to learn more should visit Hazen.ai
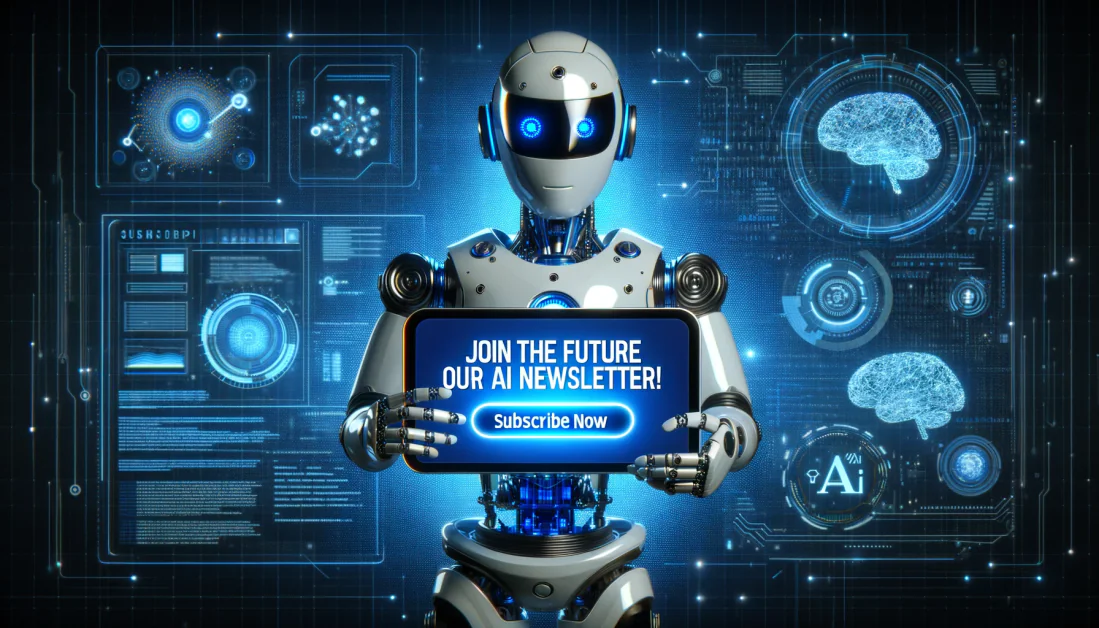
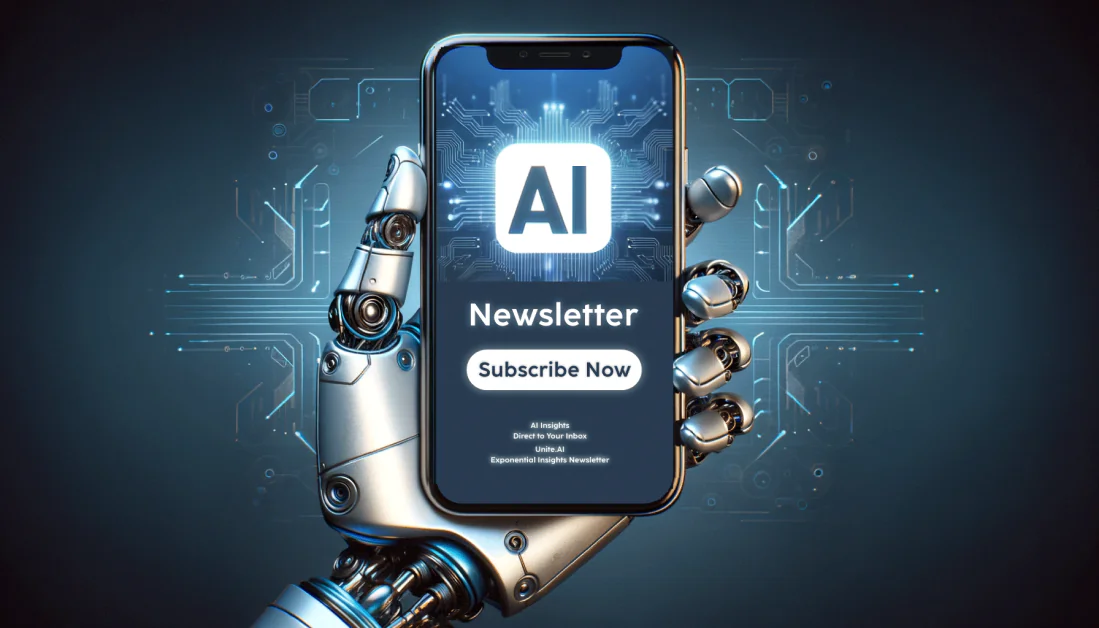