Ethics
Researchers Develop Algorithms Aimed At Preventing Bad Behaviour in AI
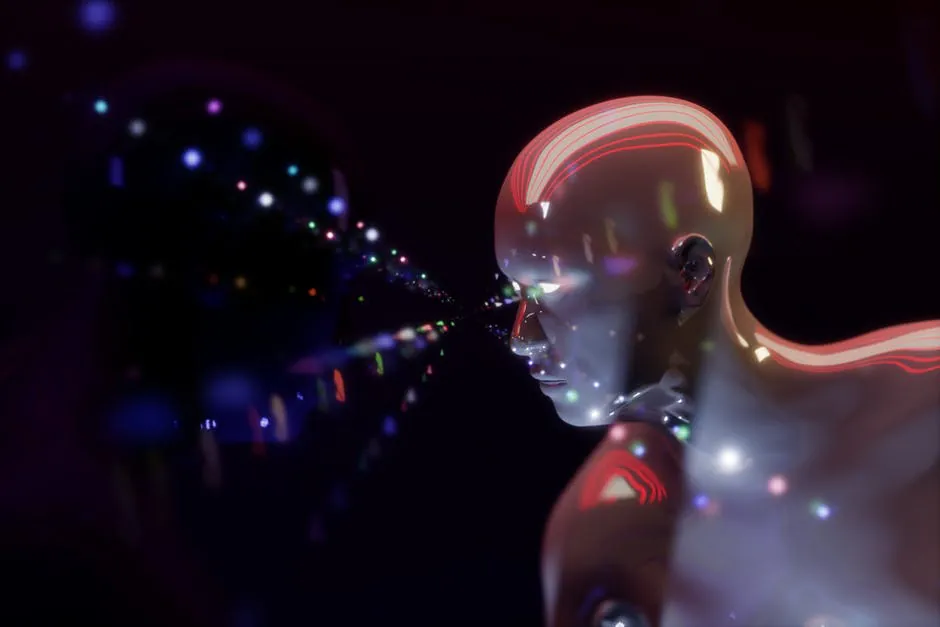
Along with all the advancements and advantages artificial intelligence has exhibited so far, there were also reports of undesirable side effects like racial and gender bias in AI. So as sciencealert.com poses the question “how can scientists ensure that advanced thinking systems can be fair, or even safe?”
The answer may be laying the report by researchers at Stanford and the University of Massachusetts Amherst, titled Preventing undesirable behavior of intelligent machines. As eurekaalert.org notes in its story about this report, AI is now starting to handle sensitive tasks, so “policymakers are insisting that computer scientists offer assurances that automated systems have been designed to minimize, if not completely avoid, unwanted outcomes such as excessive risk or racial and gender bias.”
The report this team of researchers presented “outlines a new technique that translates a fuzzy goal, such as avoiding gender bias, into the precise mathematical criteria that would allow a machine-learning algorithm to train an AI application to avoid that behavior.”
The purpose was, as Emma Brunskill, an assistant professor of computer science at Stanford and senior author of the paper points out “we want to advance AI that respects the values of its human users and justifies the trust we place in autonomous systems.”
The idea was to define “unsafe” or “unfair” outcomes or behaviors in mathematical terms. This would, according to the researchers, making it possible “to create algorithms that can learn from data on how to avoid these unwanted results with high confidence.”
The second goal was to “develop a set of techniques that would make it easy for users to specify what sorts of unwanted behavior they want to constrain and enable machine learning designers to predict with confidence that a system trained using past data can be relied upon when it is applied in real-world circumstances.”
ScienceAlert says that the team named this new system ‘Seldonian' algorithms, after the central character of Isaac Asimov's famous Foundation series of sci-fi novels. Philip Thomas, an assistant professor of computer science at the University of Massachusetts Amherst and first author of the paper notes, “If I use a Seldonian algorithm for diabetes treatment, I can specify that undesirable behavior means dangerously low blood sugar or hypoglycemia.”
“I can say to the machine, ‘While you're trying to improve the controller in the insulin pump, don't make changes that would increase the frequency of hypoglycemia.' Most algorithms don't give you a way to put this type of constraint on behavior; it wasn't included in early designs.”
Thomas adds that “this Seldonian framework will make it easier for machine learning designers to build behavior-avoidance instructions into all sorts of algorithms, in a way that can enable them to assess the probability that trained systems will function properly in the real world.”
For her part, Emma Brunskill also notes that “thinking about how we can create algorithms that best respect values like safety and fairness is essential as society increasingly relies on AI.”
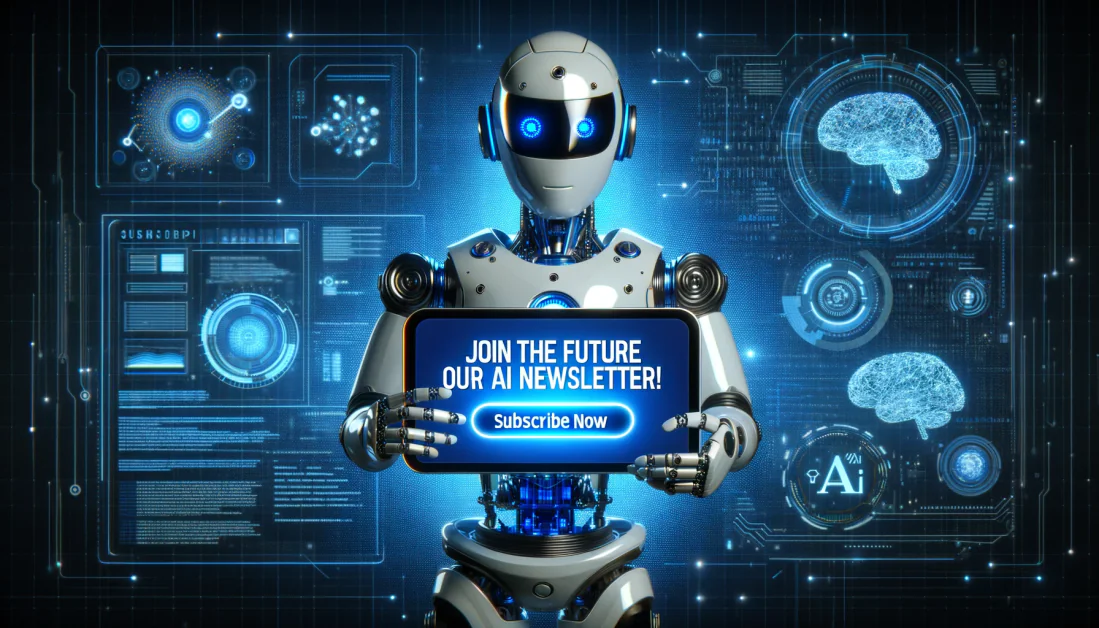
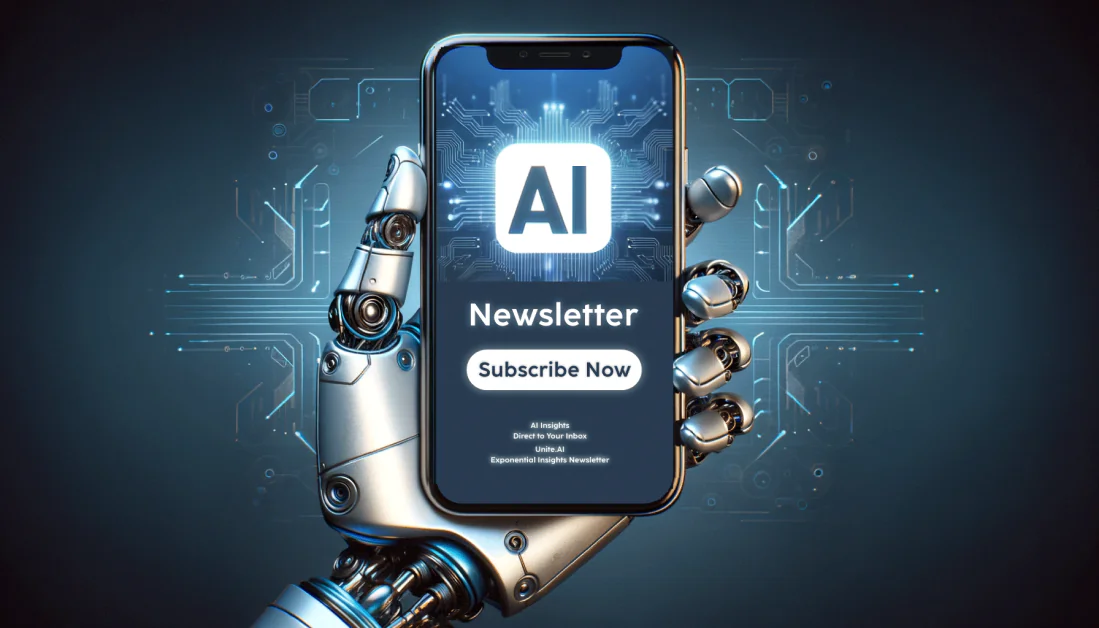