Artificial Intelligence
Researchers Create Alternative to GPU
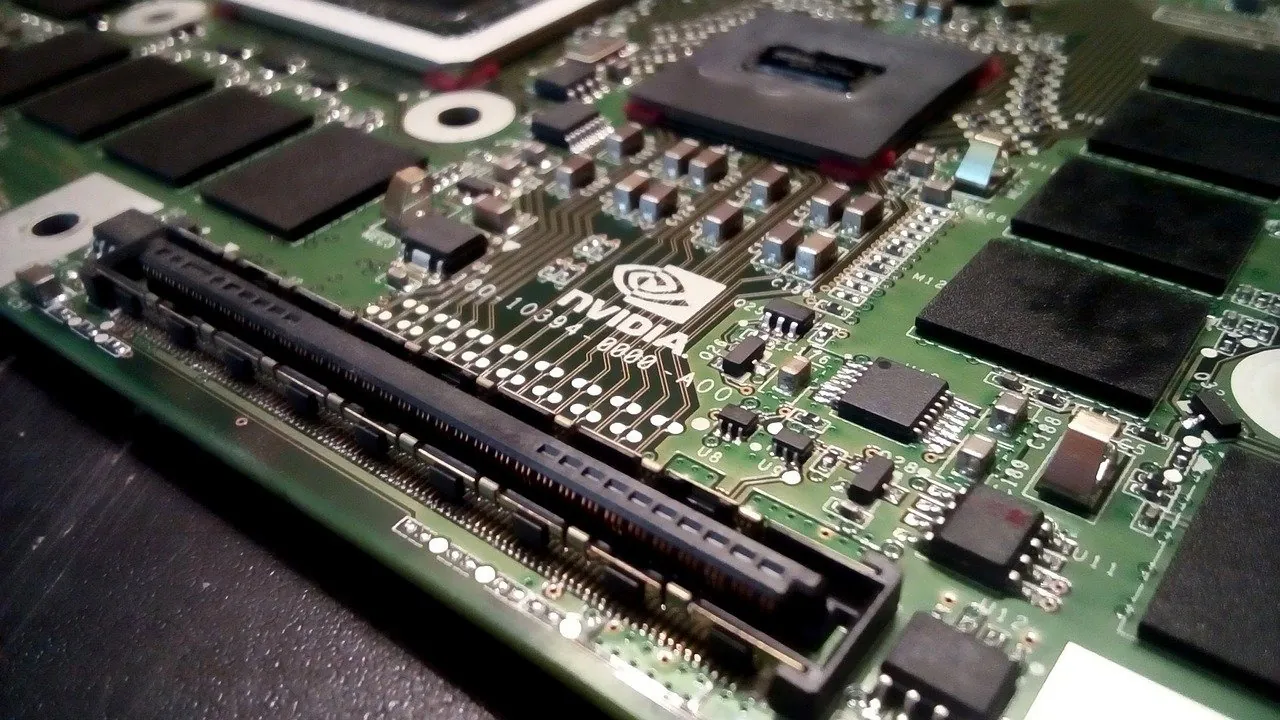
Computer scientists from Rice University, along with collaborators from Intel, have developed a more cost-efficient alternative to GPU. The new algorithm is called “sub-linear deep learning engine” (SLIDE), and it uses general-purpose central processing units (CPUs) without specialized acceleration hardware.
The results were presented at the Austin Convention Center, which holds the machine learning systems conference MLSys.
One of the biggest challenges within artificial intelligence (AI) surrounds specialized acceleration hardware such as graphics processing units (GPUs). Before the new developments, it was believed that in order to speed up deep learning technology, the use of this specialized acceleration hardware was required.
Many companies have placed great importance in investing in GPUs and specialized hardware for deep learning, which is responsible for technology such as digital assistants, facial recognition, and product recommendation systems. One such company is Nvidia, which creates the Tesla V100 Tensor Core GPUs. Nvidia recently reported a 41% increase in its fourth-quarter revenues compared to last year.
The development of SLIDE opens up entirely new possibilities.
Anshumali Shrivastava is an assistant professor in Rice’s Brown School of Engineering and helped invent SLIDE with graduate students Beidi Chen and Tharun Medini.
“Our tests show that SLIDE is the first smart algorithmic implementation of deep learning on CPU that can outperform GPU hardware acceleration on industry-scale recommendation datasets with large fully connected architectures,” said Shrivastava.
SLIDE gets past the challenge of GPUs because of its completely different approach to deep learning. Currently, the standard training technique for deep neural networks is “back propagation,” and it requires matrix multiplication. This workload requires the use of GPUs, so the researchers altered the neural network training so that it could be solved with hash tables.
This new approach greatly reduces the computational overhead for SLIDE. The current best GPU platform that companies like Amazon and Google use for cloud-based deep learning has eight Tesla V100s, and the price tag is around $100,000.
“We have one in the lab, and in our test case we took a workload that's perfect for V100, one with more than 100 million parameters in large, fully connected networks that fit in GPU memory,” Shrivastava said. “We trained it with the best (software) package out there, Google's TensorFlow, and it took 3 1/2 hours to train.
“We then showed that our new algorithm can do the training in one hour, not on GPUs but on a 44-core Xeon-class CPU,” he continued.
Hashing is a type of data-indexing method invented in the 1990s for internet search. Numerical methods are used to encode large amounts of information as a string of digits, which is called a hash. Hashes are listed to create tables that can be searched quickly.
“It would have made no sense to implement our algorithm on TensorFlow or PyTorch because the first thing they want to do is convert whatever you're doing into a matrix multiplication problem,” Chen said. “That is precisely what we wanted to get away from. So we wrote our own C++ code from scratch.”
According to Shrivastava, the biggest advantage of SLIDE is that it is data parallel.
“By data parallel I mean that if I have two data instances that I want to train on, let's say one is an image of a cat and the other of a bus, they will likely activate different neurons, and SLIDE can update, or train on these two independently,” he said. “This is much a better utilization of parallelism for CPUs.”
“The flipside, compared to GPU, is that we require a big memory,” he said. “There is a cache hierarchy in main memory, and if you're not careful with it you can run into a problem called cache thrashing, where you get a lot of cache misses.”
SLIDE has opened the door for new ways to implement deep learning, and Shrivastava believes it is just the beginning.
“We've just scratched the surface,” he said. “There's a lot we can still do to optimize. We have not used vectorization, for example, or built-in accelerators in the CPU, like Intel Deep Learning Boost. There are a lot of other tricks we could still use to make this even faster.”
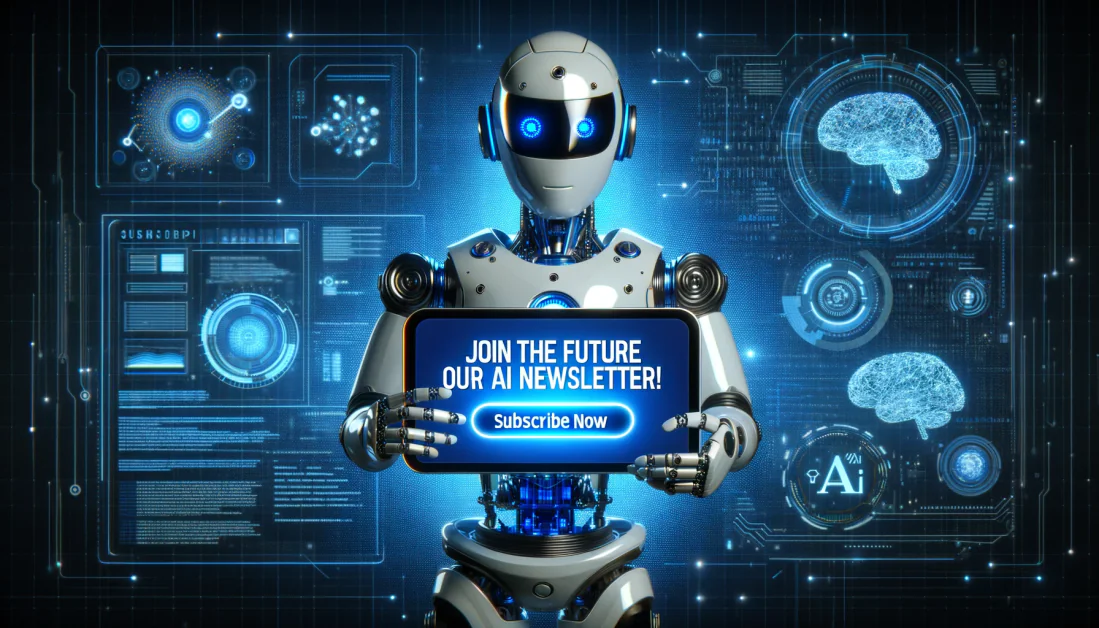
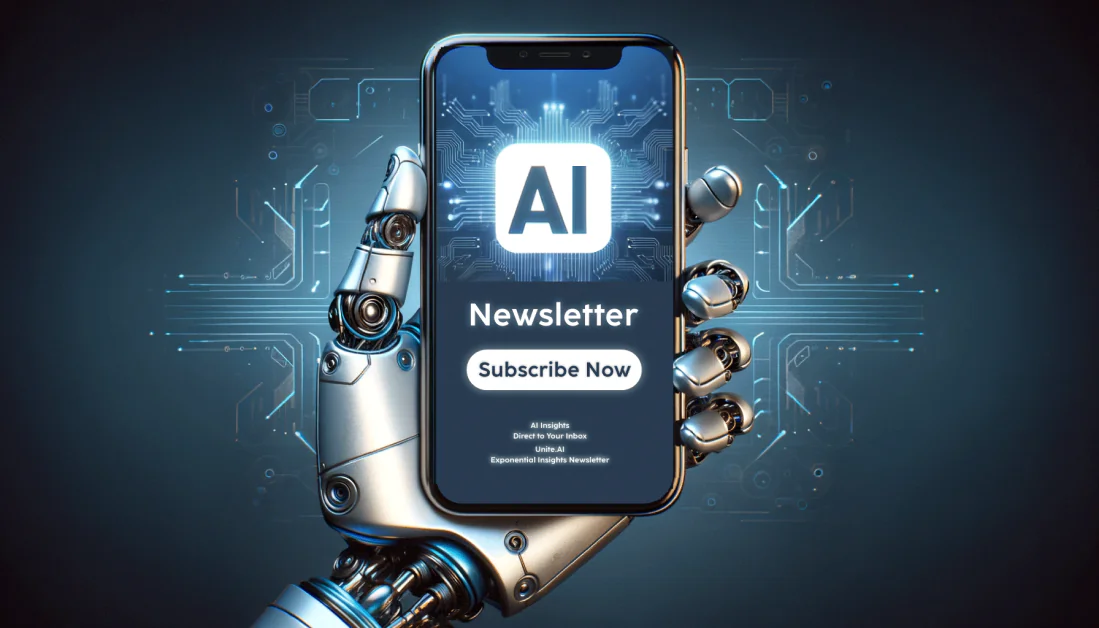