Interviews
Orr Danon, CEO & Co-Founder of Hailo – Interview Series
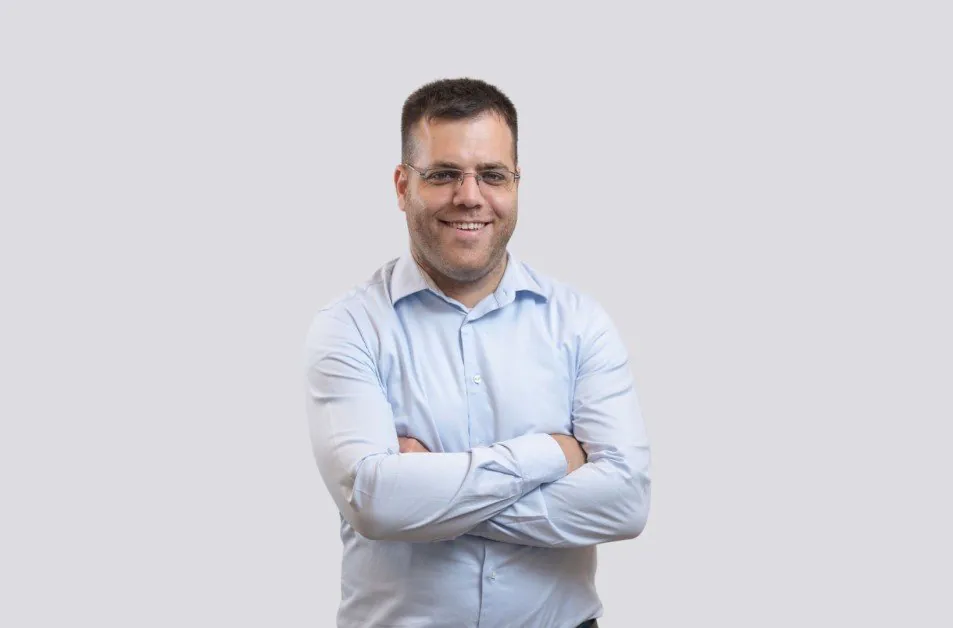
Orr Danon, is the CEO & Co-Founder of Hailo, a company with the mission of enabling smart edge technologies to reach their full potential. The solution Hailo presents bridges the gap between existing and future AI technologies and the compute capacity needed to power these applications. The company is focused on building AI processors efficient and compact enough to compute and interpret vast amounts of data in real-time.
Could you share the genesis story behind Hailo?
I co-founded Hailo in 2017 along with colleagues I had met previously in the Israeli Defense Forces’ (IDF) elite technology unit. While working with my co-founders Rami Feig and Avi Baum on IoT (Internet of Things) solutions, a lesser-known construct – “Deep Learning” – kept popping up throughout our research. Eventually, we brought together experts in the field to develop a new deep-learning solution that aimed at solving the shortcomings of aging computer architecture in order to enable smart devices to operate more effectively and efficiently at the edge. After Rami’s unfortunate passing, the Hailo team saw his vision through – creating Hailo’s groundbreaking AI processor.
Could you briefly explain why edge computing is often a superior solution to cloud computing?
When we started Hailo, disruptive AI technologies were largely limited to the cloud, or large data centers, as they are costly, require high computing power and extensive hardware to run, and consume a significant amount of energy. We believe that AI is helping create a better, safer, more productive, and more exciting world, but for this to happen, AI needs to be available at the edge as well. For implementation of real-time and low-latency applications on devices such as network-connected cameras, vehicles, and IoT devices, processing at the source is essential for effective operation. With edge AI, we can fully harness a number of key use cases powering the future of smart cities, intelligent transportation, autonomous driving, video management systems (VMS), Industry 4.0, and more.
What are some of the challenges behind processing visual data on the edge?
The goal is to get as much performance and as many features as can be packed into edge devices so they can process an enormous amount of visual data swiftly and with little latency; yet one of the key constraints is power consumption – both in terms of how much power can be delivered to the device and the heat generated by the processor.
With intelligent cameras, for example, manufacturers need an AI processor to fit into a 2-3W envelope because the camera cannot use fan cooling and because it generally will have a limited power supply. These are acute pain points because at such low power, performance is extremely limited when using most of the processors on the market.
How did Hailo reimagine AI Processor architecture?
We did so by specifically designing an AI processor that is built to work on edge devices, taking into account the size and power limitations. By doing so, we enable unprecedented compute power on edge devices, enabling them to run AI more efficiently and effectively and to perform sophisticated deep learning applications such as object detection, object recognition, segmentation, and others, with performance levels previously possible only in the cloud. This unique architecture allows multi-stream and multi-application processing, improving the performance and cost-effectiveness of edge devices.
One example of the use of this architecture is Video Management Systems (VMS). These systems are being utilized in areas with numerous cameras, such as office buildings, stadiums, smart city applications and highways to better manage safety and security, including monitoring for emergencies and accidents, suspicious activity, traffic management, access control, toll collection and more. For many years, enterprises relied entirely on manual processes when it came to collecting, analyzing, and storing video data. Now, with Hailo’s unique neural network architecture, VMS can carry out multiple tasks in parallel, in real time, enabling the processing of more channels and more applications concurrently. Applications include advanced license plate recognition (LPR), traffic monitoring, behavioral detection, and more.
Could you discuss the neural network processing core and your approach of calculating neural networks in parallel versus sequentially?
Our AI processor combines multiple innovations which address the fundamental properties of neural networks. We applied an innovative control scheme that is based on a combination of hardware and software to reach very low joules per operation with a high degree of flexibility.
Our unique dataflow-oriented architecture adapts to the structure of the neural network and allows high-resource utilization. The Hailo dataflow compiler is composed of full stack software, co-designed with our hardware, to enable efficient deployment of neural networks. The dataflow compiler receives the user model as input. As part of the build flow, the dataflow compiler breaks down each of the network layers to the required computational elements, generating a resource graph which is a representation of the target network. The dataflow compiler then matches the target network’s resource graph to the physical resources available on the processor, generating a customized data pipe for the target network. When carried out in this fashion, running a model on a device is highly efficient, using minimal compute resources at all times.
What are some of the current Hailo-based platforms that are available for businesses?
The Hailo-8™ processor and the AI modules can be plugged into a variety of edge devices, helping power multiple sectors with superior AI capabilities – including automotive, smart cities, smart retail, and industry 4.0.
Hailo has partnered with leading VMS and ISV players such as Innovatrics, Network Optix, GeoVision, and Art of Logic, to enable top-performing video analytics at scale.
How much time can these solutions save clients that are integrating AI solutions?
Sourcing integrated solutions that run on established VMS platforms is time saving, but this is not the main benefit of the system. The Hailo-based VMS solutions enable more streams to run in parallel, and more applications to be processed for each stream.
The ability to harness AI to process multiple video streams also means that only specific events need to be streamed to the cloud for storage, enabling significant savings on bandwidth and storage capacity.
What are some lessons that you’ve learned from deploying deep learning applications in edge devices?
We’ve seen firsthand how AI at the edge will play a key role in driving innovation across a wide variety of sectors in the coming years. As businesses seek solutions that ensure their devices are more powerful, versatile, responsive and secure, the cloud will continue giving way to edge devices and hybrid models. Those who succeed in implementing AI at the edge will gain an edge across the board.
What’s your vision for the future of edge computing?
Edge computing — specifically AI at the edge — has the ability to completely transform how the world around us works, enabling devices such as intelligent cameras, smart vehicles, autonomous robots, advanced traffic management tools, smart construction, smart factories and more. AI at the edge has the power to change anything and everything, enabling new applications to make our world smarter and safer. Hailo’s AI processing technology is a major enabler of all these use cases. We will continue to partner with manufacturers and innovators across the globe to make these solutions more accessible.
Thank you for the great interview, readers who wish to learn more should visit Hailo.
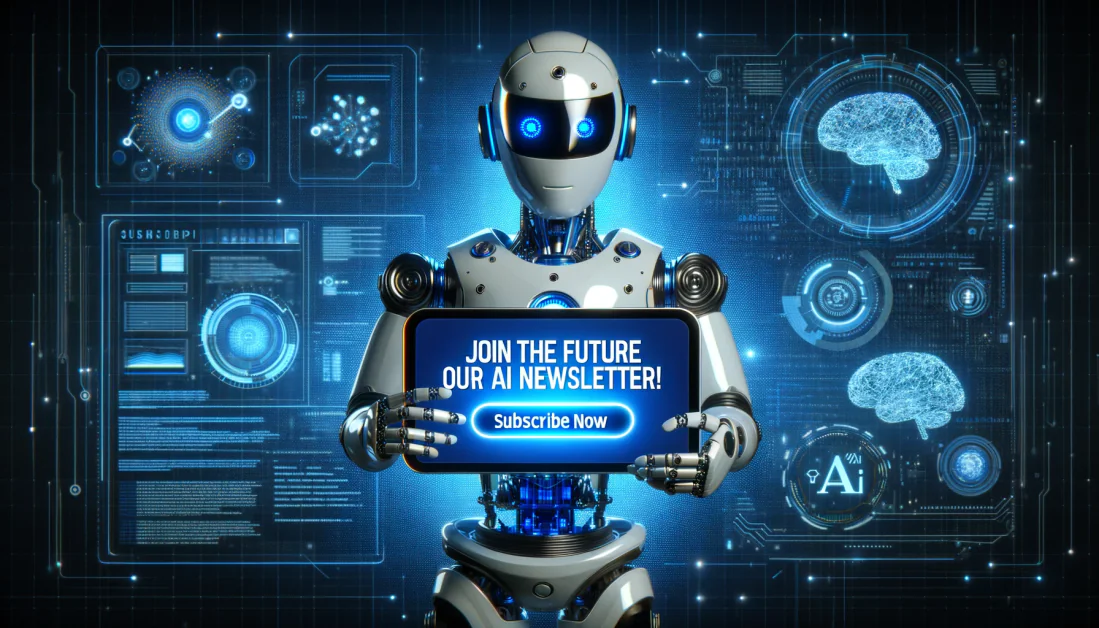
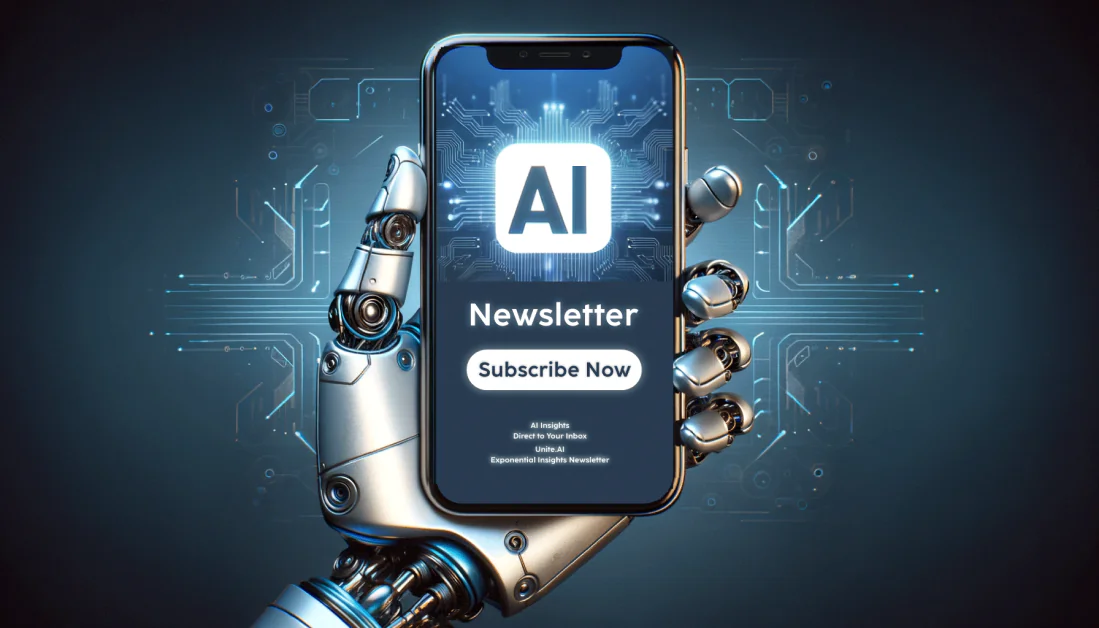