Artificial Intelligence
New Study Attempts to Improve Hate Speech Detection Algorithms
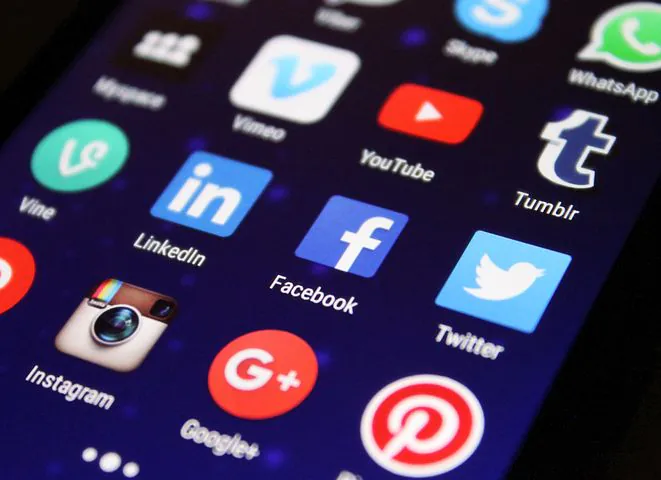
Social media companies, especially Twitter, have long faced criticism for how they flag speech and decide which accounts to ban. The underlying problem almost always has to do with the algorithms that they use to monitor online posts. Artificial intelligence systems are far from perfect when it comes to this task, but there is work constantly being done to improve them.
Included in that work is a new study coming out of the University of Southern California that attempts to reduce certain errors that could result in racial bias.
Failure to Recognize Context
One of the issues that doesn’t receive as much attention has to do with algorithms that are meant to stop the spread of hateful speech but actually amplify racial bias. This happens when the algorithms fail to recognize context and end up flagging or blocking tweets from minority groups.
The biggest problem with the algorithms in regard to context is that they are oversensitive to certain group-identifying terms like “black,” “gay,” and “transgender.” The algorithms consider these hate speech classifiers, but they are often used by members of those groups and the setting is important.
In an attempt to resolve this issue of context blindness, the researchers created a more context-sensitive hate speech classifier. The new algorithm is less likely to mislabel a post as hate speech.
The Algorithm
The researchers developed the new algorithms with two new factors in mind: the context in regard to the group identifiers, and whether there are also other features of hate speech present in the post, like dehumanizing language.
Brendan Kennedy is a computer science Ph.D. student and co-lead author of the study, which was published on July 6 at ACL 2020.
“We want to move hate speech detection closer to being ready for real-world application,” said Kennedy.
“Hate speech detection models often ‘break,' or generate bad predictions, when introduced to real-world data, such as social media or other online text data, because they are biased by the data on which they are trained to associate the appearance of social identifying terms with hate speech.”
The reason the algorithms are oftentimes inaccurate is that they are trained on imbalanced datasets with extremely high rates of hate speech. Because of this, the algorithms fail to learn how to handle what social media actually looks like in the real world.
Professor Xiang is an expert in natural language processing.
“It is key for models to not ignore identifiers, but to match them with the right context,” said Ren.
“If you teach a model from an imbalanced dataset, the model starts picking up weird patterns and blocking users inappropriately.”
To test the algorithm, the researchers used a random sample of text from two social media sites that have a high-rate of hate speech. The text was first hand-flagged by humans as prejudiced or dehumanizing. The state-of-the-art model was then measured against the researchers' own model for inappropriately flagging non-hate speech, through the use of 12,500 New York Times articles with no hate speech present. While the state-of-the-art models were able to achieve 77% accuracy in identifying hate vs non-hate, the researcher’s model was higher at 90%.
“This work by itself does not make hate speech detection perfect, that is a huge project that many are working on, but it makes incremental progress,” said Kennedy.
“In addition to preventing social media posts by members of protected groups from being inappropriately censored, we hope our work will help ensure that hate speech detection does not do unnecessary harm by reinforcing spurious associations of prejudice and dehumanization with social groups.”
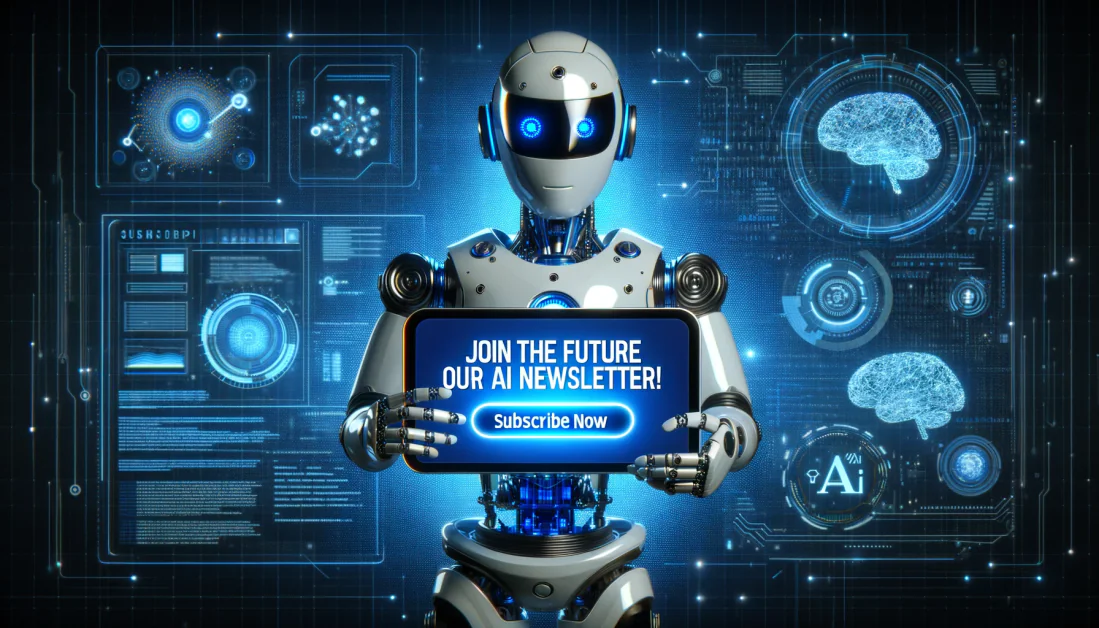
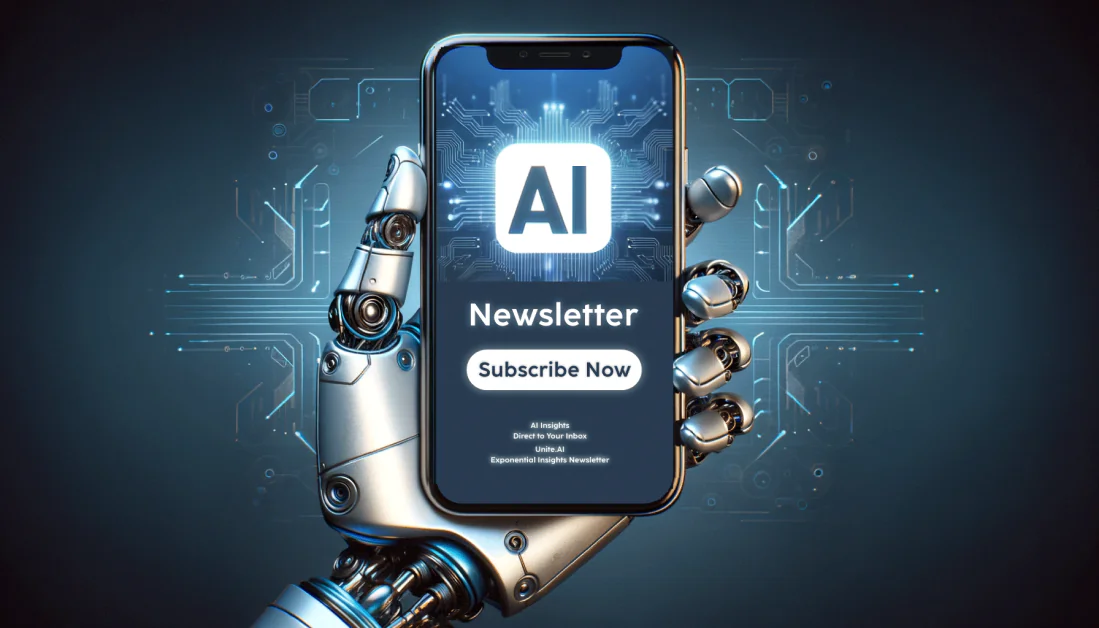