Artificial Intelligence
Neural Networks Learn Better by Mimicking Human Sleep Patterns
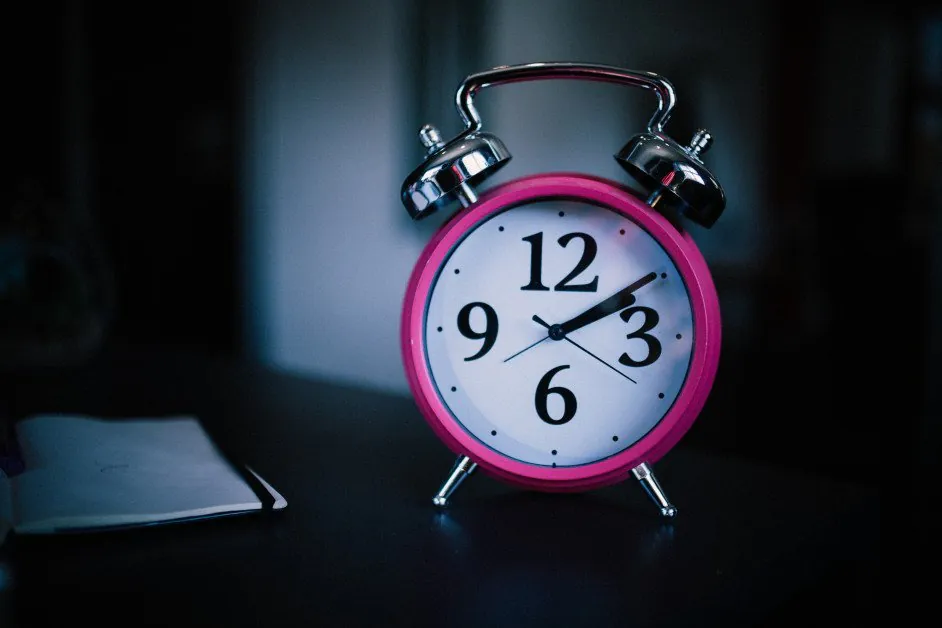
A team of researchers at the University of California – San Diego is exploring how artificial neural networks could mimic sleep patterns of the human brain to mitigate the problem of catastrophic forgetting.
The research was published in PLOS Computational Biology.
On average, humans require 7 to 13 hours of sleep per 24 hours. While sleep relaxes the body in many ways, the brain still stays very active.
Active Brain During Sleep
Maxim Bazhenov, PhD, is a professor of medicine and sleep researcher at University of California San Diego School of Medicine.
“The brain is very busy when we sleep, repeating what we learned during the day,” Bazhenov says. “Sleep helps reorganize memories and presents them in the most efficient way.”
Bazhenov and his team have published previous work on how sleep builds rational memory, which is the ability to remember arbitrary or indirect associations between objects, people or events. It also protects against forgetting old memories.
The Problem of Catasrophic Forgetting
Artificial neural networks draw inspiration from the architecture of the human brain to improve AI technologies and systems. While these technologies have managed to achieve superhuman performance in the form of computational speed, they have one major limitation. When neural networks learn sequentially, new information overwrites previous information in a phenomenon referred to as catastrophic forgetting.
“In contrast, the human brain learns continuously and incorporates new data into existing knowledge, and it typically learns best when new training is interweaved with periods of sleep for memory consolidation,” Bazhenov says.
The team used spiking neural networks that artificially mimic natural neural systems. Rather than being communicated continuously, information is transmitted as discrete events, or spikes, at certain time points.
Mimicking Sleep in Neural Networks
The researchers discovered that when spiking networks were trained on new tasks with occasional off-line periods mimicking sleep, the problem of catastrophic forgetting was mitigated. Similar to the human brain, the researchers say “sleep” enables the networks to replay old memories without explicitly using old training data.
“When we learn new information, neurons fire in specific order and this increases synapses between them,” Bazhenov says. “During sleep, the spiking patterns learned during our awake state are repeated spontaneously. It’s called reactivation or replay.
“Synaptic plasticity, the capacity to be altered or molded, is still in place during sleep and it can further enhance synaptic weight patterns that represent the memory, helping to prevent forgetting or to enable transfer of knowledge from old to new tasks.”
The team found that by applying this approach to artificial neural networks, it helped the networks avoid catastrophic forgetting.
“It meant that these networks could learn continuously, like humans or animals,” Bazhenov continues. “Understanding how the human brain processes information during sleep can help to augment memory in human subjects. Augmenting sleep rhythms can lead to better memory.
“In other projects, we use computer models to develop optimal strategies to apply stimulation during sleep, such as auditory tones, that enhance sleep rhythms and improve learning. This may be particularly important when memory is non-optimal, such as when memory declines in aging or in some conditions like Alzheimer’s disease.”
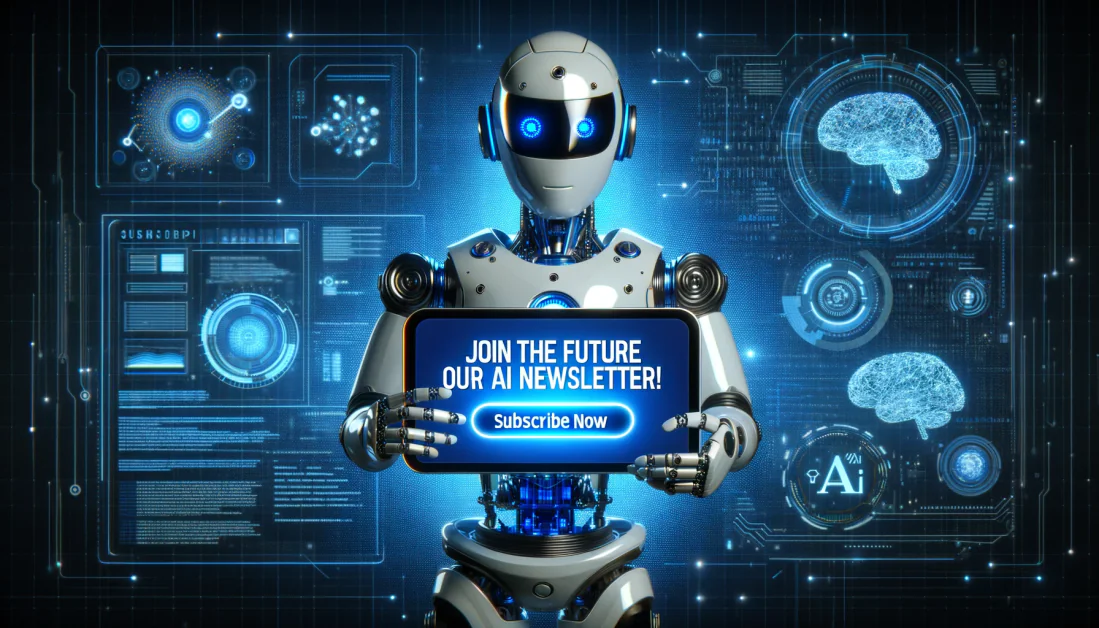
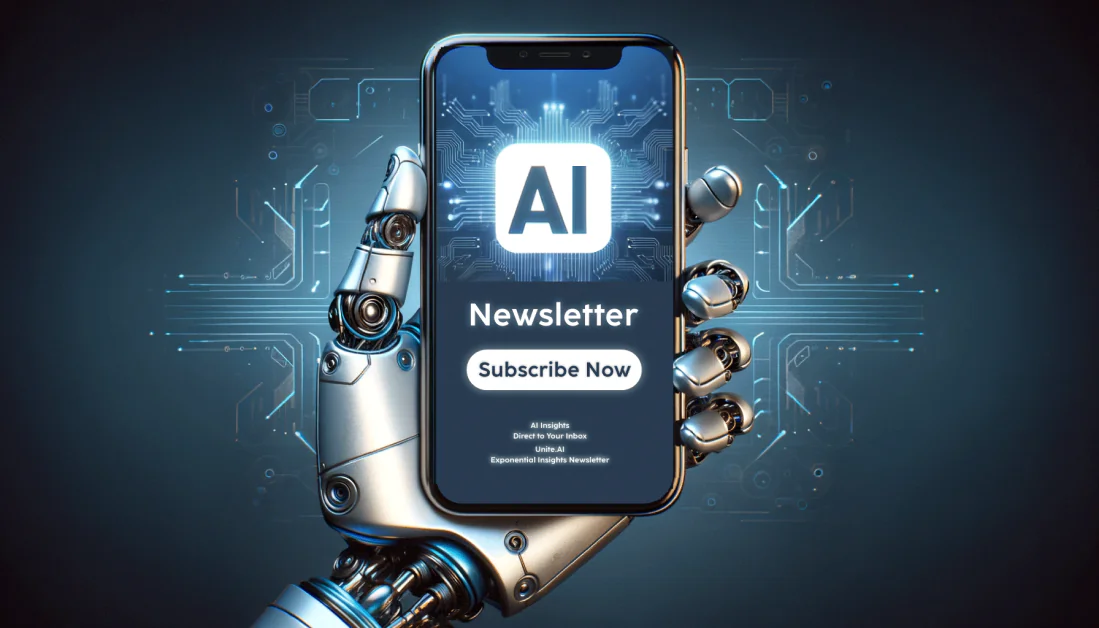