Interviews
Mohammad Omar, Co-Founder & CEO of LXT – Interview Series
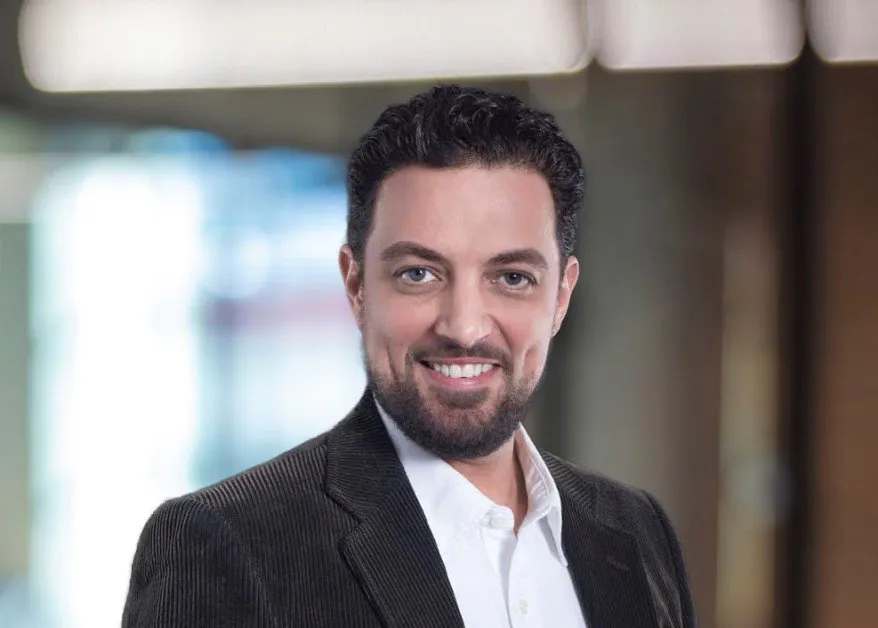
Mohammad Omar is the Co-Founder & CEO of LXT, an emerging leader in AI training data to power intelligent technology for global organizations, including the largest technology companies in the world. In partnership with an international network of contributors, LXT collects and annotates data across multiple modalities with the speed, scale, and agility required by the enterprise. Founded in 2010, LXT is headquartered in Toronto, Canada with a presence in the United States, Australia, India, Turkey, UK and Egypt.
Could you share the genesis story behind LXT?
LXT was founded in response to an acute need for data that my employer from twelve years ago was facing. At that time, the company needed Arabic data but didn’t have the right suppliers from which to source it. Being a risk-taker and entrepreneur by nature, I decided to resign from my role, set up a new company, and turn right back around to offer our services to my former employer. Right away we were given some of their most challenging projects which we successfully delivered on, and things just grew from there. Now over 12 years later, we have built a strong relationship with this company, becoming a go-to supplier for high-quality language data.
What are some of the biggest challenges behind deploying AI at scale?
That’s a great question, and we actually included that in our latest research report, The Path to AI Maturity. The top challenge that respondents cited was integrating their existing or legacy systems into AI solutions. This makes sense given the fact that we surveyed larger companies that would most likely have an array of tech systems across their organizations that need to be rationalized into a digital transformation strategy. Other challenges that respondents ranked highly were a lack of skilled talent, lack of training or resources, and sourcing quality data. I wasn’t surprised by these responses as they are commonly cited, and also of course because the data challenge is our organization’s reason for being.
When it comes to data challenges, LXT can both source data and label it so that machine learning algorithms can make sense of it. We are equipped to do this at scale and with agility, meaning that we deliver high-quality data very quickly. Clients often come to us when they are getting ready for a launch and want to make sure their product is well received by customers,
By working with us to source and label data, companies can address their resource and talent shortages by allowing their teams to focus on building innovative solutions.
LXT offers coverage for over 750 languages, but there are translation and localization challenges that go beyond the structure of language itself. Could you discuss how LXT confronts these challenges?
There certainly are translation and localization challenges – especially once you branch out beyond the most widely spoken languages that tend to have official status and the level of standardization that goes along with that. Many of the languages that we work in have no official orthography, so managing consistency across a team becomes a challenge. We address these and other challenges – e.g. detection of fraudulent behavior – by having rigorous processes in place for quality assurance. Again it was very apparent in the AI maturity research report that for most organizations working with AI data, quality sat at the top of the list of priorities. And most organizations surveyed expressed willingness to pay more to get this.
For companies who require data sourcing and data annotation, how early on in the application development journey should they begin sourcing this data?
We recommend that organizations create a data strategy as soon as they identify their AI use case. Waiting until the application is in development can lead to a lot of unnecessary rework, as the AI may learn the wrong things and have to be retrained by quality data, which can take time to source and integrate into the development process.
What’s the rule of thumb for knowing the frequency that data should be updated?
It really depends on the type of application you are developing and how often the data that supports it changes in a significant way. This means that data is a representation of real life, and over time, the data must be updated to provide an accurate reflection of what is happening in the world. We call this phenomenon model drift, of which there are two types, each requiring the retraining of algorithms.
- Concept drift occurs when a significant difference between the training data and the AI output changes, which can happen suddenly or more gradually. For instance, a retailer might use historical customer data to train an AI application. But when a massive shift in consumer reality occurs, the algorithm will need to be retrained in order to reflect this.
- Data drift takes place when the data used to train an application no longer reflects the actual data encountered when it enters production. This can be caused by a range of factors, including demographic shifts, seasonality or the situation of an application in a new geographic region.
LXT recently unveiled a report titled “The Path to AI Maturity 2023”. What were some of the takeaways in this report that took you by surprise?
It probably shouldn’t have come as a surprise, but the thing that really stood out was the diversity of applications. You might have expected two or three domains of activity to dominate, but when we asked where the respondents planned to focus their AI efforts, and where they planned to deploy their AI, it initially looked like chaos – the absence of any trend at all. But on sifting through the data, and looking at the qualitative responses, it became clear that the absence of a trend is the trend. At least through the eyes of our respondents, if you have a problem, then there is a real possibility that someone is working on an AI solution to it.
Generative AI is taking the world by storm, what is your view on how far language generative models can take the industry?
My personal take on this is that central to the real power of Generative Artificial Intelligence – I’m choosing to use the words here rather than the abbreviation for emphasis – is Natural Language Understanding. The ‘intelligence’ of AI is learned through language; the ability to address and ultimately solve complex problems is mediated through iterative and cumulative natural language interactions. With this in mind, I believe language generative models will be in lockstep with other elements of AI all the way.
What is your vision for the future of AI and for the future of LXT?
I am an optimist by nature and that will color my response here, but my vision for the future of AI is to see it improve quality of life for everyone; for it to make our world a safer place, a better place for future generations. At a micro level, my vision for LXT is to see the organization continue to build on its strengths, to grow and become an employer of choice, and a force for good, for the global community that makes our business possible. At a macro level, my vision for LXT is to contribute in a significant, meaningful way to the fulfillment of my optimistically skewed vision for the future of AI.
Thank you for the great interview, readers who wish to learn more should visit LXT.
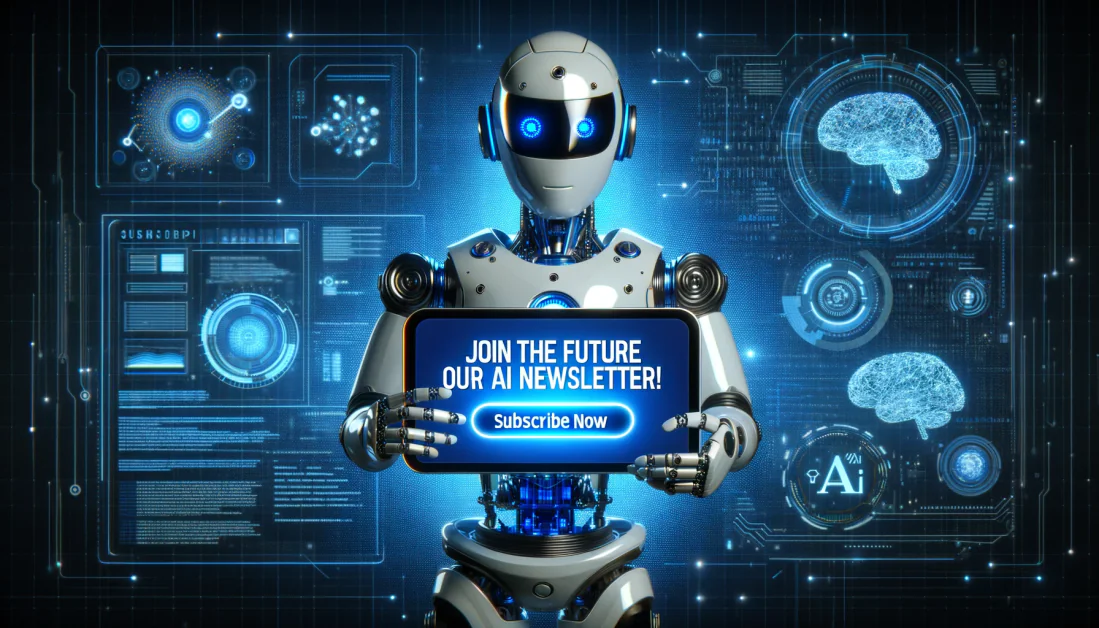
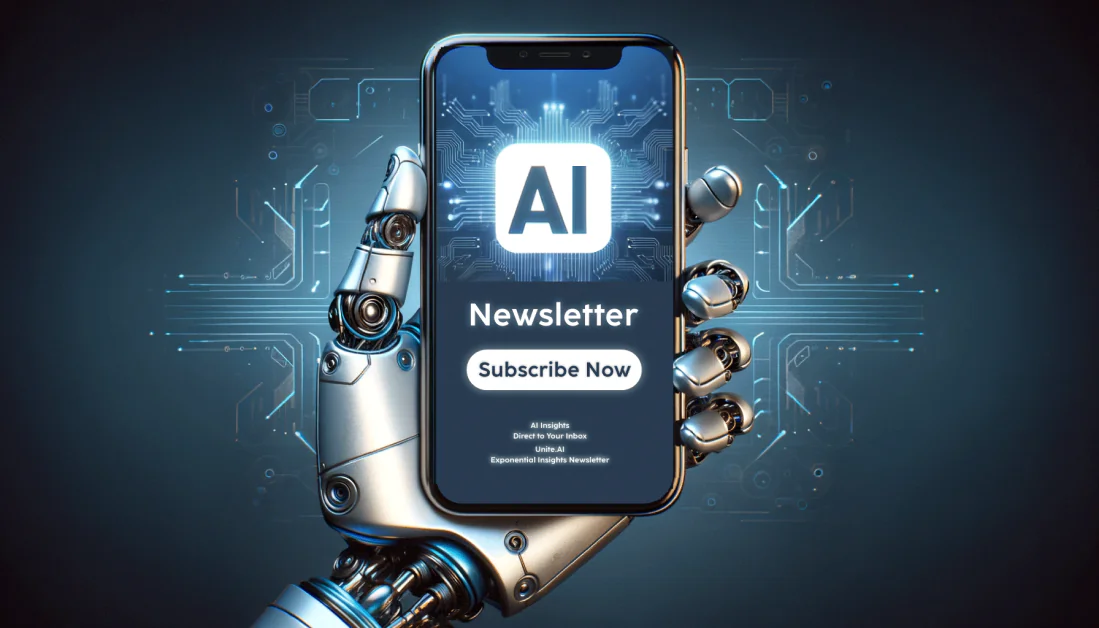