Artificial Intelligence
MIT: Measuring Media Bias in Major News Outlets With Machine Learning
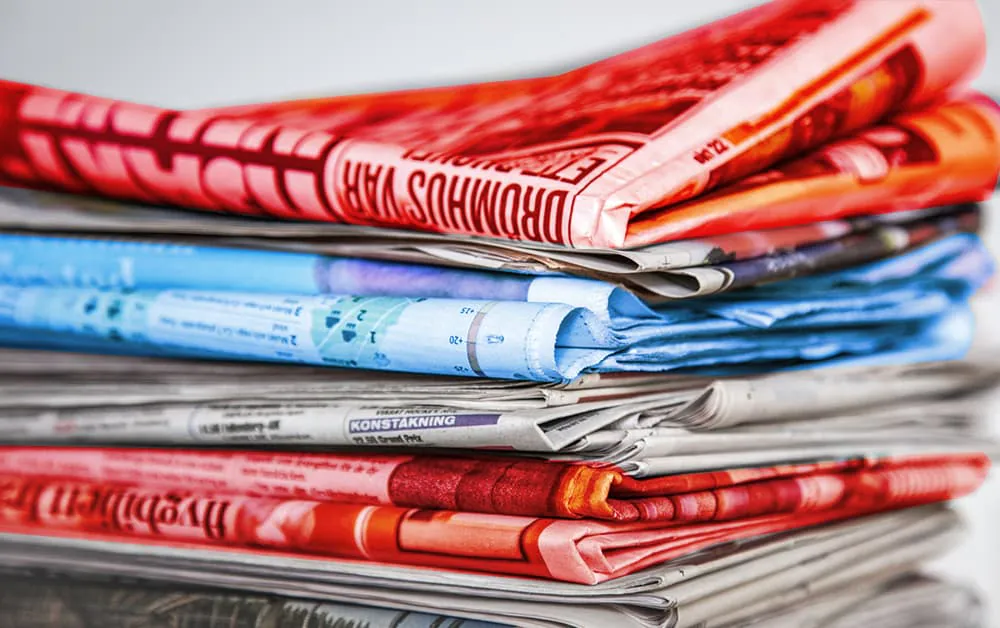
A study from MIT has used machine learning techniques to identify biased phrasing across around 100 of the largest and most influential news outlets in the US and beyond, including 83 of the most influential print news publications. It's a research effort that shows the way towards automated systems that could potentially auto-classify the political character of a publication, and give readers a deeper insight into the ethical stance of an outlet on topics that they may feel passionately about.
The work centers on the way topics are addressed with particular phrasing, such as undocumented immigrant | illegal Immigrant, fetus | unborn baby, demonstrators | anarchists.
The project used Natural Language Processing (NLP) techniques to extract and classify such instances of ‘charged' language (on the assumption that apparently more ‘neutral' terms also represent a political stance) into a broad mapping that reveals left and right-leaning bias across over three million articles from around 100 news outlets, resulting in a navigable bias landscape of the publications in question.
The paper comes from Samantha D'Alonzo and Max Tegmark at MIT's Department of Physics, and observes that a number of recent initiatives around ‘fact checking', in the wake of numerous ‘fake news' scandals, can be interpreted as disingenuous and serving the causes of particular interests. The project is intended to provide a more data-driven approach to studying the use of bias and ‘influencing' language in a supposedly neutral news context.
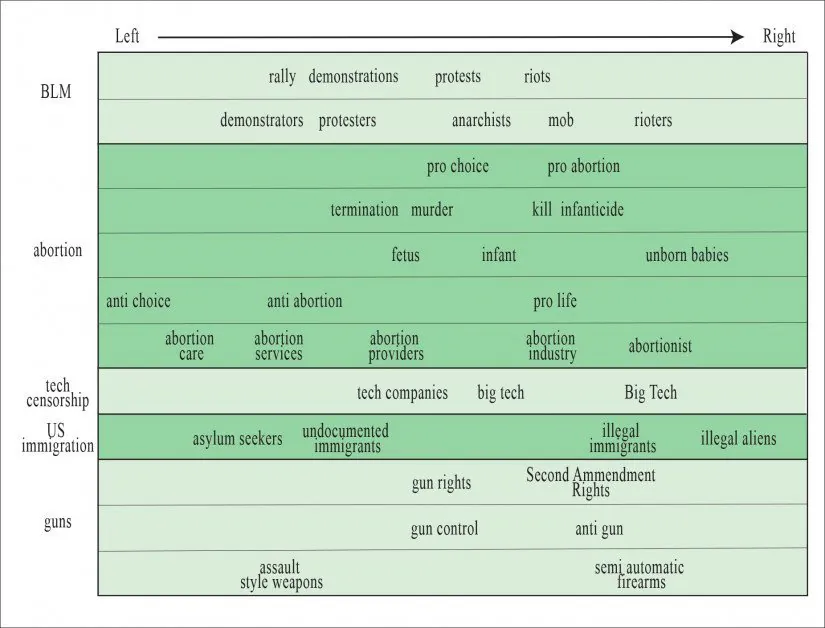
A spectrum of (literally) left-to-right phrases, as derived from the study. Source: https://arxiv.org/pdf/2109.00024.pdf
NLP Processing
The source data from the study was obtained from the open source Newspaper3K database, and comprised 3,078,624 articles obtained from 100 media news sources, including 83 newspapers. The newspapers were selected on the basis of their reach, while online media sources also included articles from the military news analysis site Defense One, and Science.
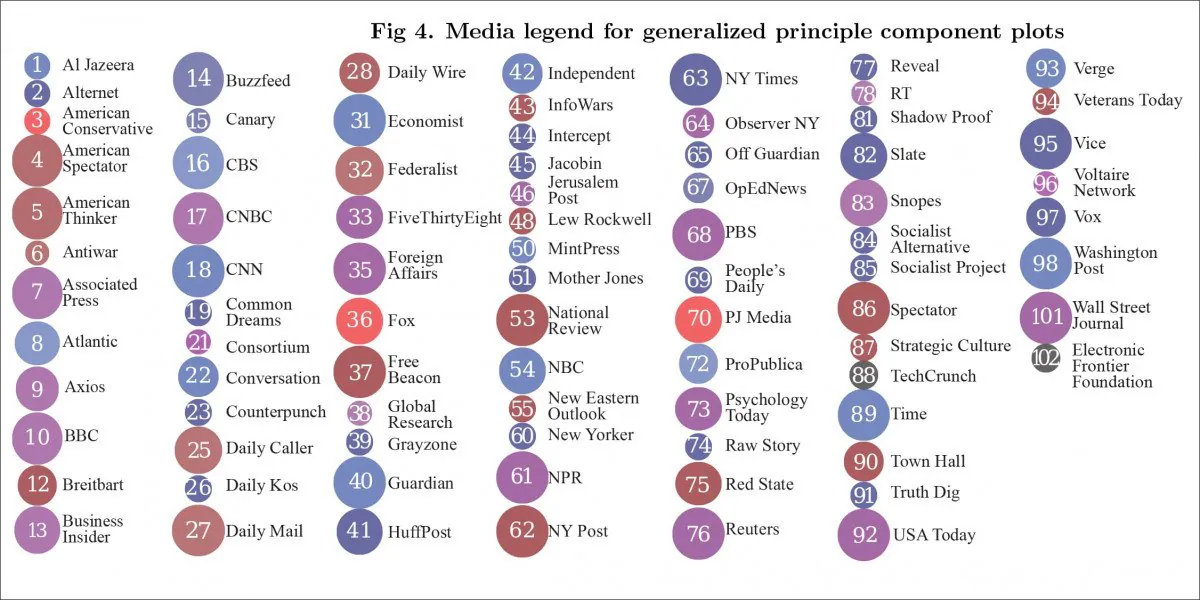
The sources used in the study.
The paper reports that the downloaded text was ‘minimally' pre-processed. Direct quotes were eliminated, since the study is interested in the language chosen by journalists (even though quote selections are in themselves an interesting field of study).
British spellings were changed to American to standardize the database, all punctuation removed, and all but ordinal numbers also removed. Initial sentence capitalization was converted to lower-case, but all other capitalization retained.
The first 100,000 most common phrases were identified, and finally ranked, purged and merged into a phrase list. All redundant language that could be identified (such as ‘Share this article' and ‘article republished') was likewise deleted. Variations across essentially identical phrases (i.e. ‘big tech' and ‘Big Tech', ‘cybersecurity' and ‘cyber security') were standardized.
‘Nutpicking'
The initial test was on the topic ‘Black lives matter', and was able to discern phrase bias and valent synonyms across the data.
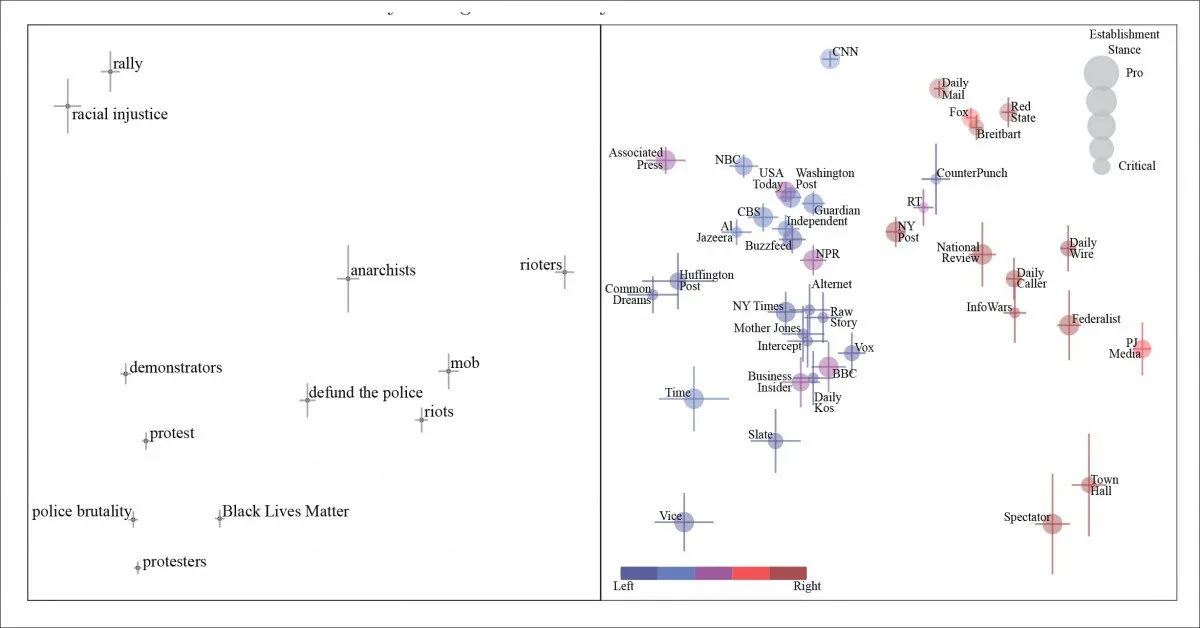
Generalized principle components for articles about Black Lives Matter (BLM). We see people participating in civil action characterized, literally and figuratively left to right, as demonstrators, anarchists and, at the right-most end of the spectrum, as ‘rioters'. The newspapers originating the phrase are represented in the right-hand panel.
While ‘protestors' transit from ‘anarchists' to ‘rioters' as we slide along the political stance of the outlet in question, the paper notes that the NLP extraction and analysis stance is hindered by the practice of ‘nutpicking' – where a media outlet will quote a phrase that's seen as valid by a differing political segment of society, and can (apparently) rely on its readership to view the phrase negatively. The paper cites ‘defund the police' as an example of this.
Naturally, this means that a ‘left-leaning' phrase appears in an otherwise right-wing context, and represents an unusual challenge for an NLP system that's relying on codified phrases to act as signifiers for political stances.
Such phrases are ‘bi-valent' [SIC] , whereas certain other phrases have such a universally negative connotation (i.e. ‘infanticide') that they are always represented as negative across a range of outlets.
The research also reveals similar mappings for ‘hot' topics such as abortion, tech censorship, US immigration and gun control.
Hobby Horses
There are certain controversial political leanings in media outlets that do not split predictably in this way, such as the topic of military spending. The paper found that ‘left-leaning' CNN ended up next to the right-leaning National Review and Fox News on this subject.
In general, however, political stance can be determined by other phrases, such as preferring the phrase ‘military-industrial complex' over the more right-leaning ‘defense industry'. The results show that the former is used by establishment-critical outlets such as Canary and American Conservative, while the latter is used more often by Fox and CNN.
The research establishes several other progressions from establishment-critical to pro-establishment language, including the gamut from ‘shot dead' to the more passive ‘the killing of'; ‘inmate felons' to ‘incarcerated people'; and ‘oil producers' to ‘big oil'.
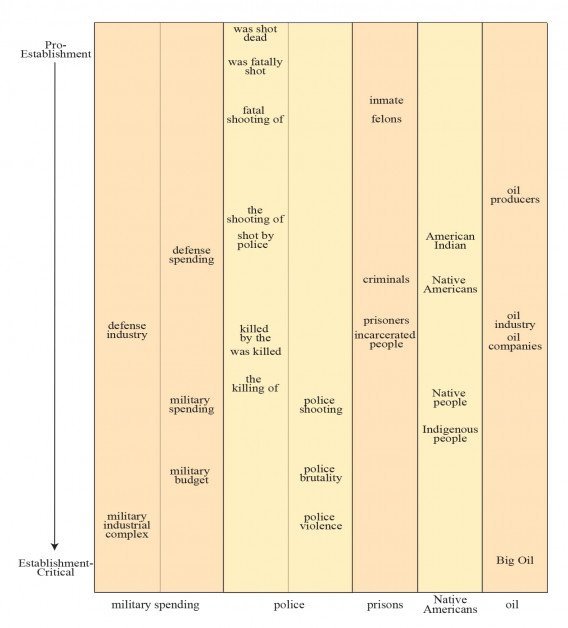
Valent synonyms with establishment bias, top to bottom.
The research acknowledges that outlets will ‘swing away' from their base political stance, either at a linguistic level (such as the use of bi-valent phrases), or for various other motives. For instance, the venerable right-wing UK publication The Spectator, established in 1828, frequently and prominently features left-wing thought pieces that abrade against the general political flow of its content stream. Whether this is done out of a sense of impartial reporting or to periodically inflame its core readership into traffic-generating comment-storms is a matter of conjecture – and not an easy case for a machine learning system that's looking for clear and consistent tokens.
These particular ‘hobby horses' and ambiguous use of ‘jarring' viewpoints among individual news organizations somewhat confuses the left-right mapping that the research ultimately offers, though providing a broad indication of political affiliation.
Withheld Significance
Though dated September 2nd and published at the end of August 2021, the paper has gained relatively little traction. Partially this could be because critical research aimed at the mainstream media is unlikely to be enthusiastically received by it; but it could also be due to the reluctance of the authors to produce clear and unambiguous graphs stratifying where influential and powerful media publications stand on various issues, along with aggregated values indicating the extent to which a publication leans towards the left or the right. In effect, the authors seem to take pains to dampen the potential incendiary effect of the results.
Likewise, the extensive published data from the project shows frequency counts of incidents of words, but seems to be anonymized, making it difficult to get a clear picture of media bias across the publications studied. Without operationalizing the project in some way, this leaves only the selected examples presented in the paper.
Later studies of this nature would possibly be more useful if they were to consider not only the phrasing used for topics, but whether the topic was covered at all, since silence speaks volumes, and has in itself a distinct political character that often speaks to more than just budgetary limitations or other pragmatic factors that may inform news selection.
Nonetheless, the MIT study seems to be the largest of its type to date, and could form the framework for future classification systems, and even secondary technologies such as browser plug-ins that might alert casual readers to the political color of the publication they are currently reading.
Bubbles, Bias and Blowback
Additionally, it would have to be considered whether such systems would further compound one of the most controversial aspects of algorithmic recommendation systems – the tendency to lead a viewer into environments where they never see a contrasting or challenging viewpoint, which is likely to further retrench the reader's stance on core issues.
Whether or not such a content bubble is a ‘safe environment', an impediment to intellectual growth, or a protection against partial propaganda, is a value judgement – a philosophical matter that is difficult to approach from the mechanistic, statistical standpoint of machine learning systems.
Further, much as the MIT study has taken pains to let the data define the results, the classifying of the political value of phrases is inevitably also a kind of value judgement, and one which cannot easily withstand the ability of language to recodify toxic or controversial content into novel phrases that aren't in the handbook, the forum rules or the training database.
If codification of this kind were to become embedded in popular online systems, it seems likely that an ongoing effort to map the ethical and political temperature of major news outlets could develop into a cold war between AI's ability to discern bias and the publishers' ability to express their standpoint in an evolving idiom designed to routinely outpace machine learning's understanding of semantics.
14/09/21 – 1.41 GMT+2 – Changed ‘100 newspapers' to ‘100 news outlets'
4:58pm – Corrected paper citation to include Samantha D'Alonzo, and related corrections.
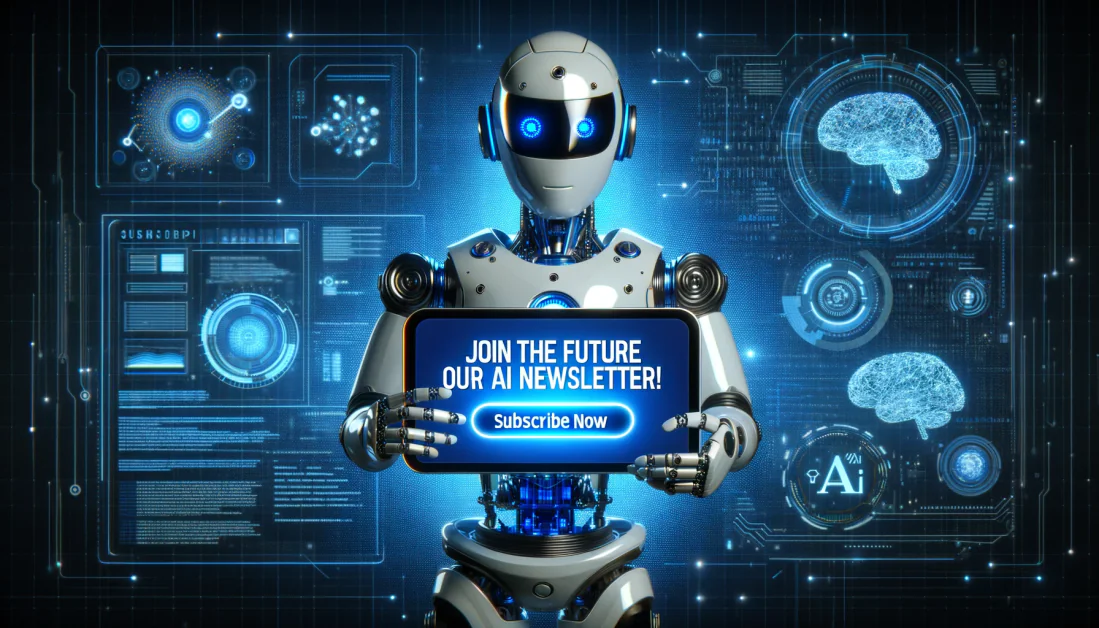
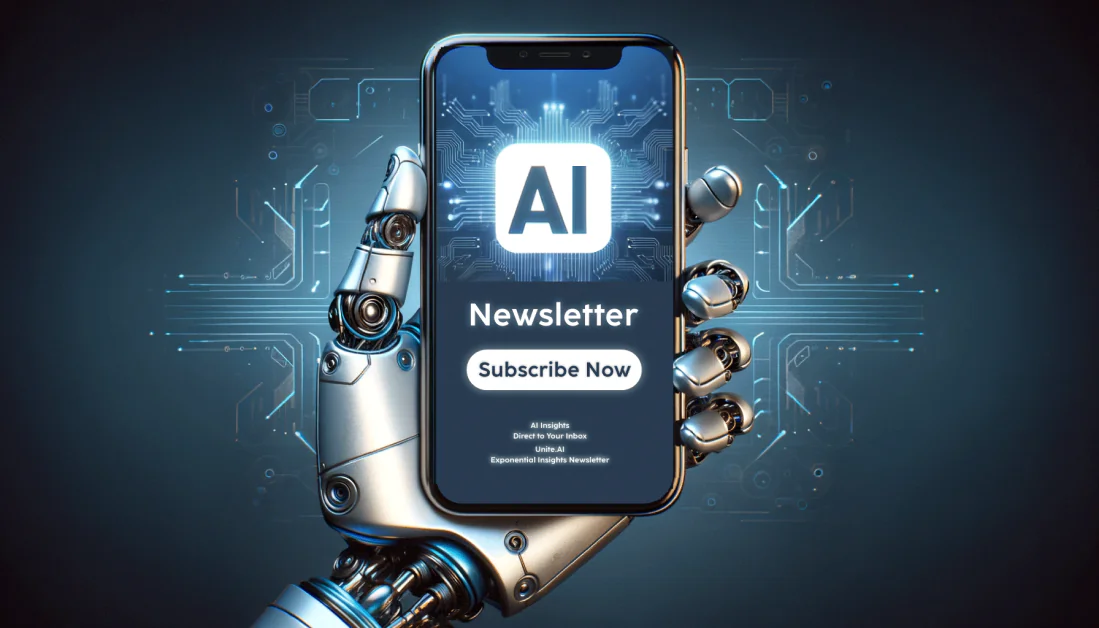