Investments
Machine Learning for Investment Management in 2021
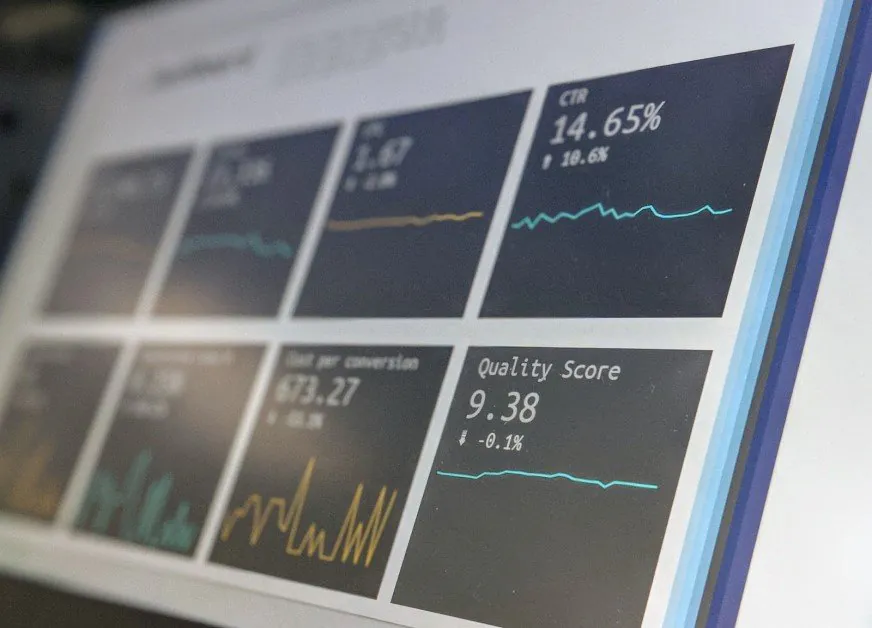
Investing is an integral part of banking, and it is also the riskiest part. Is there a way to protect your assets from unjustified risks and increase the efficiency of each new investment? Yes, machine learning and artificial intelligence in banking are pushing its boundaries, making it even more promising, profitable, smart, and secure. In this article, we will look at the future of these technologies for the fintech sector and focus on the use of AI and machine learning for investment management.
Artificial Intelligence in Banking 2021 – What to Expect
To date, artificial intelligence in banking is one of the most promising technologies to utilize for different purposes. Because of the great opportunities for customization, data analysis, and different tasks solving capabilities, as well as the affordable price to implement, the use of AI and machine learning in banking is one more fintech trend that is evolving, according to this infographic.
Machine Learning in Banking Benefits
This rapid, and most importantly, pervasive rise in the value of artificial intelligence and machine learning for banking has strong foundations, as these technologies promise completely new and highly effective benefits.
- Advanced data analysis. Previously, banks were forced to analyze data with limited access to information. For example, when a client comes with a request to issue a loan, the decision was made only on the basis of statements of income, current assets and liabilities of the client, and his credit history. At the moment, artificial intelligence in banking allows you to analyze huge amounts of information, up to the user's request in social networks, in order to make more competent and less risky decisions.
- The cutting edge advantage. Machine learning in banking may make you more competitive depending on the task you want to solve with its help. Further, we will dwell on artificial intelligence in banking case studies so you will be able to find the task that is relevant for your business and solvable with AI at the same time.
- Costs cut. Depending on the way you use AI and ML for your financial institution, it may cut you some costs. For example, using robo-advisors as a part of your support team may lower the cost of staff maintenance.
- Better security. Artificial intelligence in banking can be applied in different ways if you want to achieve more security. For example, credit card fraud detection using machine learning has become a fairly standard application of the technology, while innovative cameras with face recognition can determine if a client's intentions are false judging by their facial expressions.
Artificial Intelligence in Banking Case Studies Examples
As for the practical application of these innovations in the sphere of baking and finances, AI and ML may be successfully used for:
- Customer service improvement. For example, a chatbot may help the customers navigate the bank’s website and application, suggest making regular payments, and notify users in the case of overspendings. Even more advanced chatbots already help customers with their budget planning, money-saving, and investment management.
- Risk assessment. Making money out of money is always a risky process so AI and ML can help correctly assess the risks when issuing a loan, and make sure that the customer isn’t engaged in money laundering and terrorist financing. What’s more, machine learning for investment management and risk evaluation is quite useful as well.
- Fraud detection with AI and machine learning. Credit card fraud detection using machine learning is not the only example of utilizing this technology for security purposes. In addition, artificial intelligence can protect your employees ‘inboxes from phishing emails, as well as keep your customers' data safe in mobile banking applications. Alternatively, you can use a ready-made security solution, or partner with a company with fraud detection expertise like SPD Group to create your own fraud prevention system based on the core threats you face.
- Investment management. As for machine learning for investment management, below are more insights into this topic.
What Is Meant by Investment Management?
As defined by Investopedia, “Investment management refers to the handling of financial assets and other investments—not only buying and selling them. Management includes devising a short- or long-term strategy for acquiring and disposing of portfolio holdings. It can also include banking, budgeting, and tax services and duties, as well.”
How ML and AI Can Benefit Investment Management?
If we take one more look at the infographics above, we will discover that machine learning for investment management can be helpful with all of the core aspects of this process.
- Make new forms of data subjected to an accurate analysis. You can never be sure that you are taking into account all the information you know. In turn, AI is able to dig deeper and find invisible relationships that directly affect investment benefits.
- Decrease the influence of human biases in the decision-making process. AI has no emotions and is totally indifferent to which decision you will make. Its task is to only suggest a better deal with an unbiased look.
- Clarify the possible risks and opportunities. As we said, risk assessment is a competent machine learning advantage for investment management. Thanks to this opportunity, you will be able to make the soundest investment decisions.
- Make accurate predictions. Artificial intelligence for investment management may also be powered by a predictor which will allow you to get as accurate predictions as possible taking into account the real-time and historical data.
- Suggest the best decision being guided by certain parameters. For example, if you are looking for the opportunity to invest in real estate in a certain region, there are already two parameters the model may be guided by when looking for the best options.
What Are the Main Types of Investing Strategies and How ML Can Be Useful for Each
Here is how machine learning and artificial intelligence may work to make different investment strategies safe and beneficial.
Strategy definition | The way to apply ML and AI | |
Value Investing | This strategy means investing in undervalued but promising securities. | ML and AI can search for such stocks using data analysis predictive abilities. |
Income Investing | The purpose of this strategy is to get a constant flow of passive income. | The task of AI and ML will be analyzing different markets and current trends with the goal of finding the most passive income promising deals. |
Growth Investing | The goal of this strategy is to increase capital. The simplest example is a deposit with an interest capitalization option. | An AI-powered system may analyze different options, calculate the possible capital increase for a given period, and suggest the best solution from all the available. |
Small-Cap Investing | This is the strategy of investments in the stocks of the companies with a small market capitalization. | AI and ML may search and suggest stocks from the most promising small-cap companies. |
Socially Responsible Investing | This strategy suggests investing in green and/or socially promising projects. | The ML model may research the current social trends to find out which projects are the most promising to invest in. |
Conclusion
As you can see, machine learning and artificial intelligence are quite promising for banking and finance, especially in an environment of increased risk and lack of guarantees, that is, in investment management and countering banking fraud. In 2021, you should focus on these challenges, and leverage fintech innovation to gain an additional edge as well.
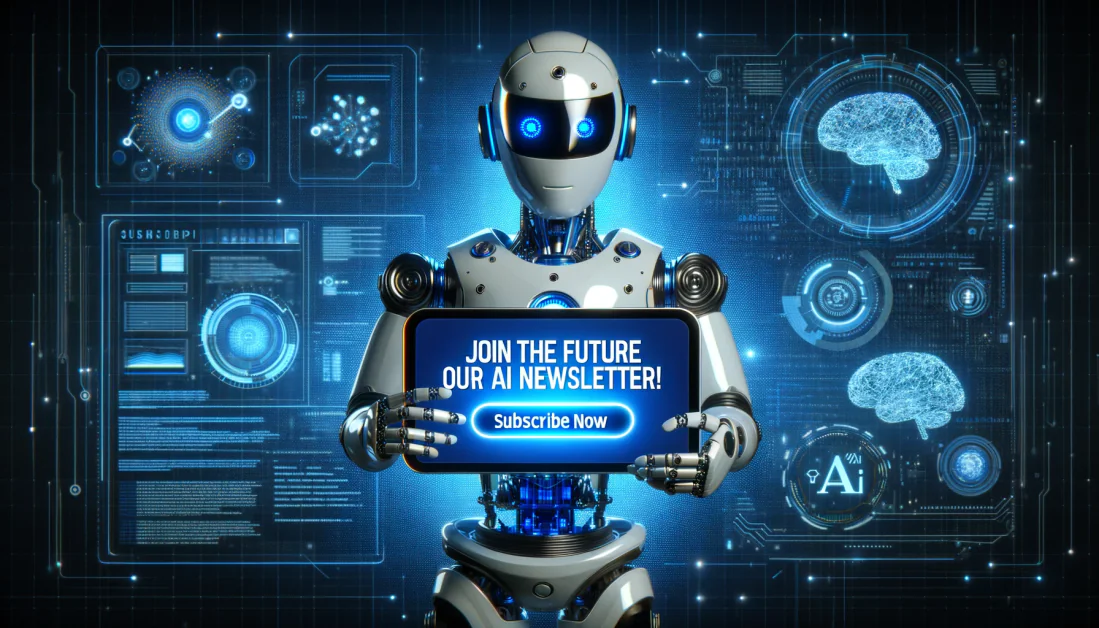
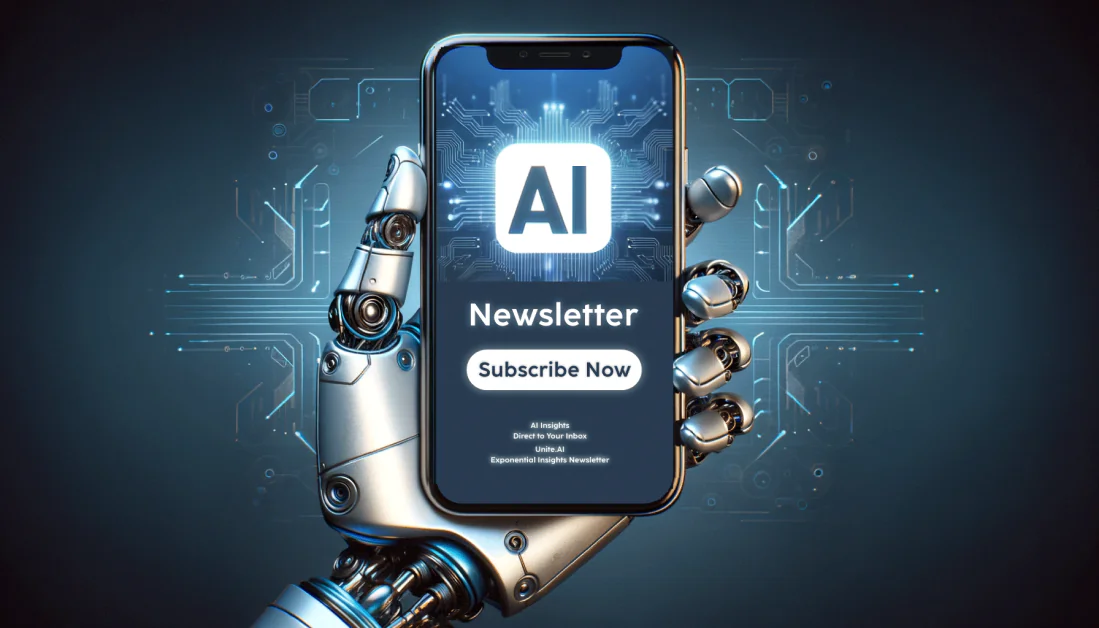