Interviews
Johnson Thomas, MD, AIBx – Interview Series
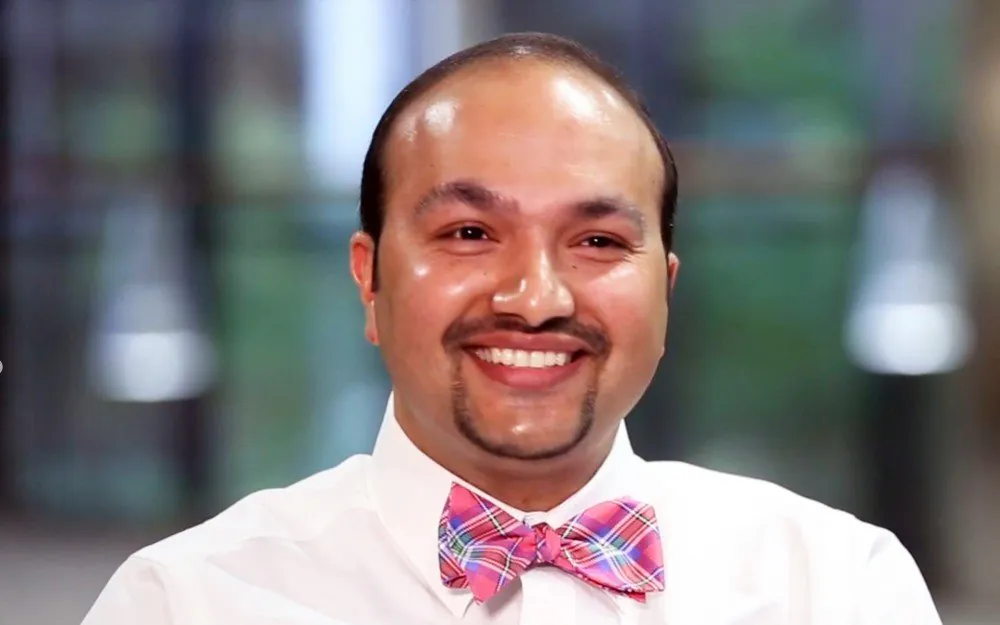
Johnson Thomas is a physician with a special interest in artificial intelligence. In addition to practicing medicine, he also enjoys programming.
His recent research project AIBx uses AI to classify thyroid nodules. This is also an explainable AI, which increases physician’s trust in the predictions, Research was published in Thyroid journal.
You’re both a practicing physician at Mercy Clinic Endocrinology in Springfield, MO, and you are a machine learning specialist. How did you find your love of both the medical world and programming eventually intersect?
I was always fascinated with computers. Growing up in India, I was fortunate to go to a school with computers. We had an active computer club and they taught us GWBasic. This was a residential school and students could use computers during their free time in the evening or during lunch breaks. So, me and my friend used to go to the lab and write codes. We were mainly creating small arcade like games.
When I graduated from the 12th grade, it was hard to decide between computer engineering and medicine. My father and grandfather were doctors. My father was excited about his work and he loved helping people. He was also very happy with his work. So finally, I decided to go to med school. Even there, I used to code a little bit. Over the years I finished med school, internal medicine residency and later completed my specialization in endocrinology. After I started working, I had a bit more time to explore coding. Around this time AI and machine learning was becoming more popular. So, I started taking online courses and then started doing small projects using publicly available medical data sets.
Your most recent research project AIBx uses AI to classify thyroid nodules, into being either positive or negative for cancer. What was it that inspired you to work on this project?
One of the areas I specialize inside endocrinology is thyroid nodules and thyroid cancers. We were doing hundreds of biopsies a year but only a few of them were cancers. This does not seem like an efficient way to use our resources. This was back in 2015. At that time, I could only work with numerical data. So, I collected ultrasound features of thyroid nodules into an excel sheet and used it to create a machine learning model using XGboost. This was deployed as a website at www.TUMScore.com.
We presented this research in Canada during the annual American Thyroid Association meeting in 2017. But this was still very subjective. Just like beauty lies in beholder’s eye, ultrasound features depends on who is reading it. There is lot of intra and interobserver variations. So, I started exploring options that are more objective. This led to image classification. But the problem with image classification is that it is mostly not explainable. How can a physician trust the algorithm? So, we decided to emulate a physician’s thought process.
Most physicians have an idea of how a cancerous thyroid nodule should look. They mentally compare a new ultrasound image to this mental picture. Based on this we decided to create an image similarity algorithm. So, when a physician uploads an image to AIBx it pulls out similar images from our database along with the actual diagnosis of those nodules. The operating physician can look at these images and accept or reject the output from AIBx. This process increases the physician’s trust in the algorithm.
How big of a dataset was originally used when you launched this project?
Thyroid ultrasound images are grayscale and they have only few patterns. Since we were using image similarity model, we didn’t need a large dataset. We had 2025 images in our database representing most of the common thyroid cancer varieties. They came from different ultrasound machines.
When it comes to deep learning big data is important. Have you seen an improvement in the diagnosis rates over time as more thyroid ultrasound images are entered into the database?
Adding more data and using different preprocessing techniques to increase the available data has helped us to improve our algorithm. Initially we used images with square aspect ratio but later we added images without square aspect ratio and that has improved our outcomes.
Are the images exclusively from your clinic, or are you having other clinics provide you with additional ultrasound images?
The present model has images from Mercy Springfield endocrinology clinic and Mercy hospital. Physicians from other health systems and countries have reached out to us to conduct a validation study using their data. We are very excited about this opportunity.
How accurate is the AI compared to a trained MD?
We compared results of AIBx to established metrics of current classification systems. In real world practice, there is wide variability in results. Positive predictive value (probability that subjects with a positive test truly have the disease) can be as low as 2% using current classification systems. What this means is that if the system predicts that 100 nodules has cancer, in reality only 2 among those 100 nodules will actually have cancer. AIBx has a positive predictive value of 65.9 percent and negative predictive value of 93.2%
How many unnecessary biopsies could we potentially reduce with this type of AI?
Based on our research, using AIBx we could have avoided more than half (57.3%) of the biopsies. But this needs to be validated using images outside our health system.
How can hospitals, MDs, or other interested parties assist with these projects?
We welcome collaboration from other MDs and hospital system. They can contact us through our website www.ThyroidBx.com or email us at [email protected]
How long do you believe it will be until machine learning replaces MDs for the diagnosis of most cancers?
Media portrays AI and physicians as competing entities. Both have their strengths and weaknesses. Symbiosis of physicians and AI, enhancing our capabilities to serve our patients is better than the current system. Because of the complementary nature of their roles, I do not think that AI will replace MDs in the near future.
What has you most excited for AI when it comes to healthcare?
I hope that AI will free the physicians from the data entry job to do what we were called for, to listen empathize and cure when we can.
We are excited to give this project additional exposure. For anyone who wishes to learn more please visit Thyroid BX.
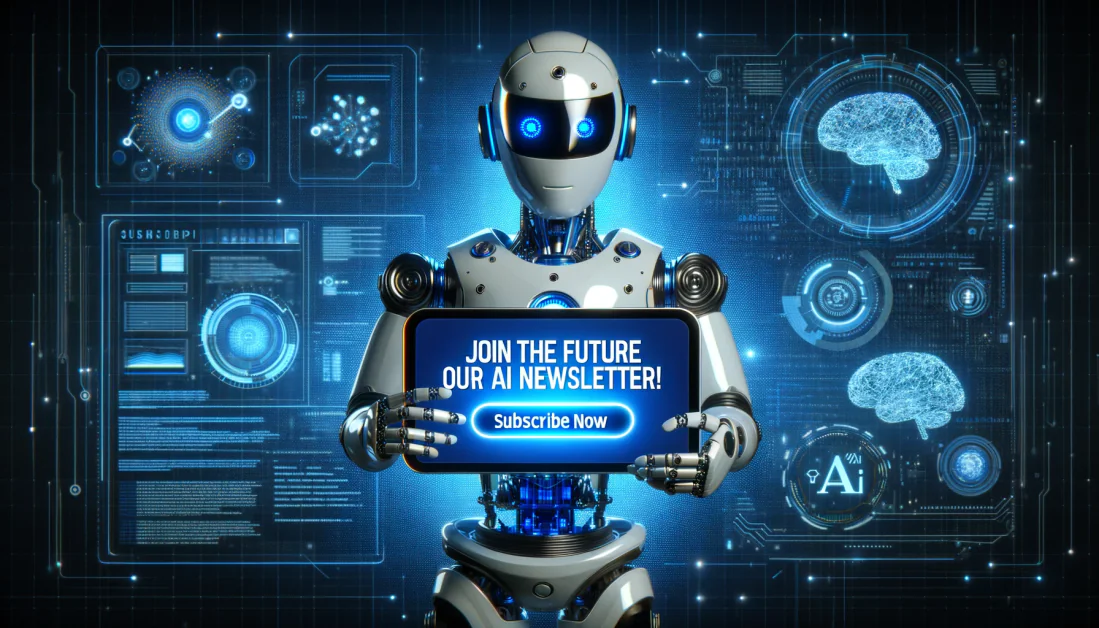
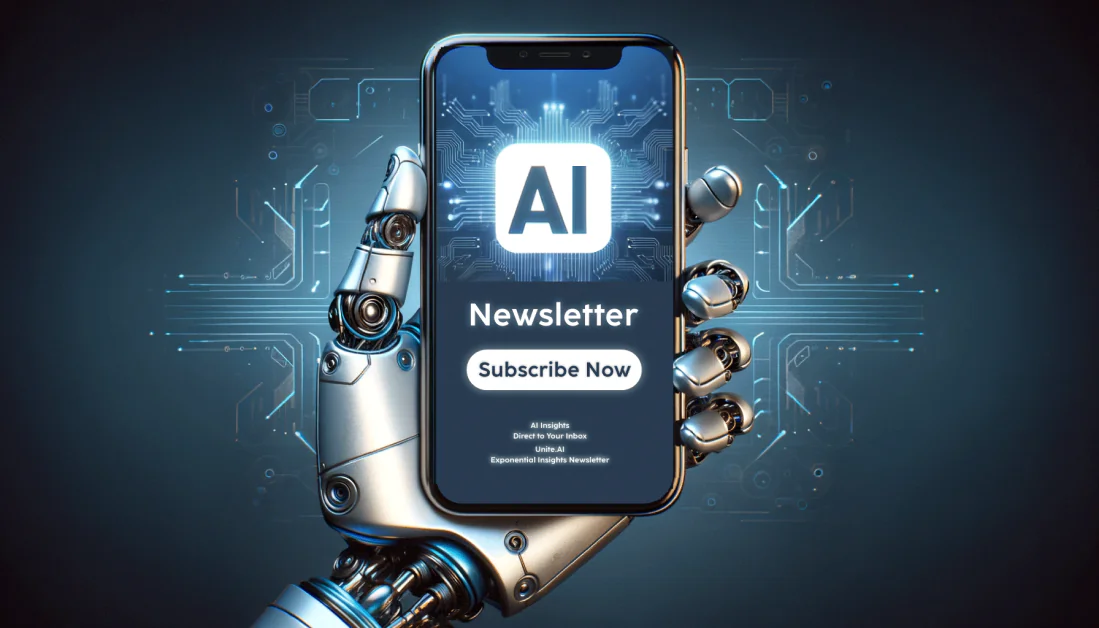